Abstract 5858: A multi-omics signature for patients’ risk classification in prostate cancer
Cancer Research(2022)
摘要
Abstract Background: Effective biomarkers are urgently needed in the clinical settings. However, most biomarkers are currently developed from single type of omics data. The goal of this study is to identify prognostic prostate cancer signatures using transcriptomics and metabolomics profiles jointly aiming to capture wider spectrum of biological information. Methods: In this study, we included 94 tumor and 48 adjacency normal samples with both transcriptomics and metabolomics profiles from Dana-Farber/Harvard Cancer Center SPORE Prostate Cancer Cohort. There were 85 patients being followed up with median length of 2.02 years including 3 lethal and 8 progression cases. We first constructed a multi-omics covering network that contained minimal set of variable pairs but sufficiently rich to account for observed inter-patient variations. The network was built on known gene-metabolite interaction pairs from Pathway Commons as prior knowledge. Next, we used a diffusion process with each of connected gene-metabolite pairs as seeds to identify candidate signatures in the network. Signature sizes were controlled under 5 by adjusting the tree height during pruning. Hierarchical clustering and survival analyses were then performed to stratify patients into two risk groups for disease-free survival probability. Only the prognostic signatures with power higher than 0.9 from bootstrapping were kept for external validation and functional analyses. Since to our best knowledge, there is no publicly available dataset with both transcriptomics and metabolomics to validate the multi-omics signature we identified. We thus trained a rank-based kTSP classifier using gene expression data in SPORE cohort as a surrogate signature and validated it in TCGA prostate cancer cohort independently. Results: Constructed covering network consisted of 12 metabolites and 54 genes with inter-patient heterogeneities being captured efficiently. We identified one high-powered multi-omics signature (Gene: EGLN3; Metabolites: succinate, trans-4-hydroxyproline) that exhibited good prognostic value where high-risk patients had significant less time of disease-free survival (log rank test: p=0.019, power: 0.974). In addition, genomic variations were observed in different percentages of patients in high and low risk groups including NCOR1(SNV, 70.6% vs 96.3% p=0.025), NKX3.1(CNV, 29.6% vs 64.7% p=0.031) and TNFRSF10C (CNV, 29.6% vs 64.7%, p=0.031). No evidence indicated the patient grouping by the signature depend on Gleason scores (p=0.44). The surrogated gene signature contained 6 pairs of genes that can effectively classify TCGA patients into two prognostic groups (log rank test: p=0.048). Still, no evidence indicated the surrogate gene signature is associated with Gleason score (p=0.23) in TCGA dataset. Conclusions: We identified a prognostic multi-omics signature (EGLN3, succinate, trans-4-hydroxyproline) with high statistical power. Citation Format: Zhuoran Xu, Elisa Benedetti, Ryan Carelli, Jacob Rosenthal, Hubert Pakula, Mohamed Omar, Renato Umeton, David Brundage, Jan Krumsiek, Massimo Loda, Luigi Marchionni. A multi-omics signature for patients’ risk classification in prostate cancer [abstract]. In: Proceedings of the American Association for Cancer Research Annual Meeting 2022; 2022 Apr 8-13. Philadelphia (PA): AACR; Cancer Res 2022;82(12_Suppl):Abstract nr 5858.
更多查看译文
关键词
prostate cancer,risk classification,multi-omics
AI 理解论文
溯源树
样例
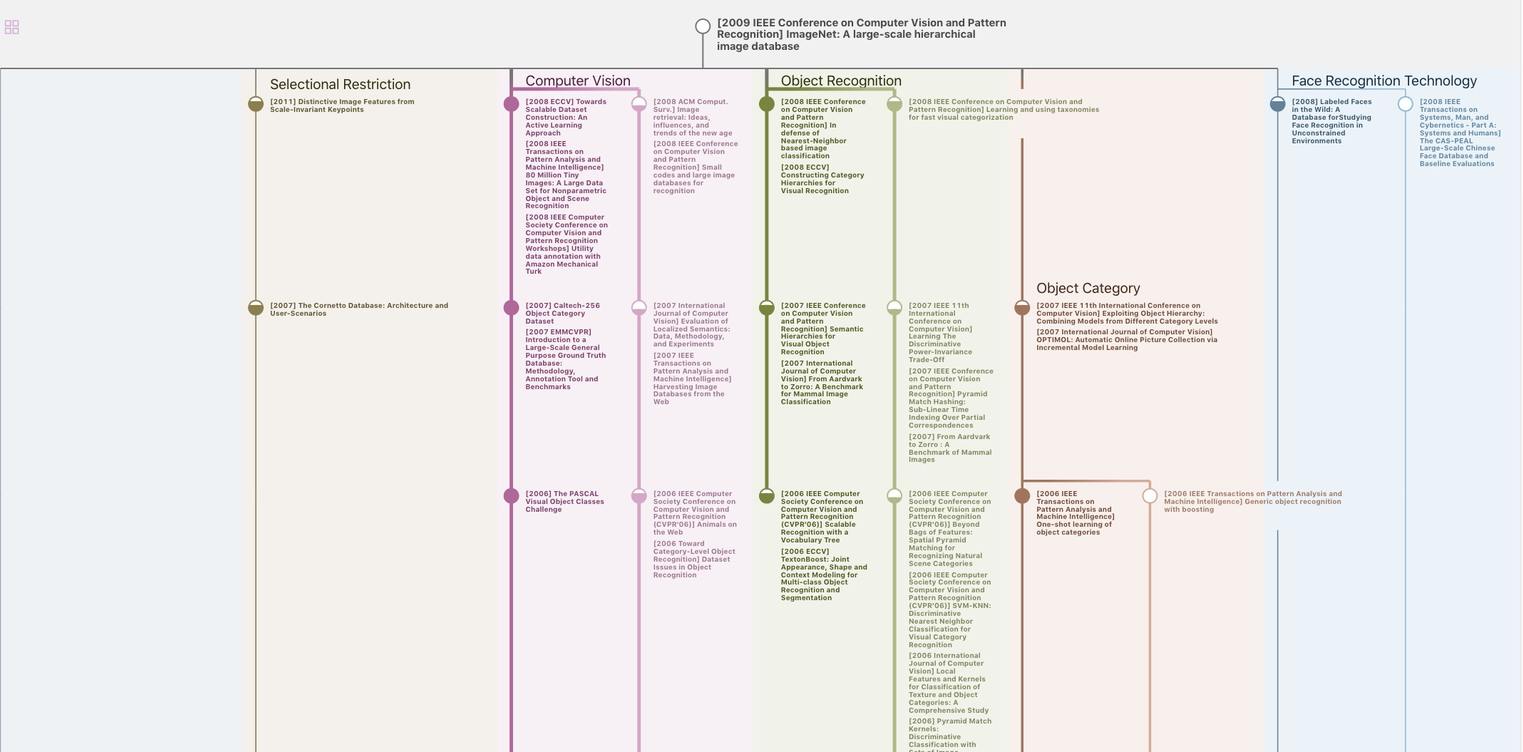
生成溯源树,研究论文发展脉络
Chat Paper
正在生成论文摘要