Abstract 2810: Modeling pHLA:TCR interactions for effective TCR therapies: Leveraging AI and molecular dynamics
Cancer Research(2022)
摘要
Abstract Background: The purpose of our study is to aid the development of adoptive T-cell receptor (TCR) -based cancer therapies with an AI platform that models binding between peptide-human leukocyte antigen (pHLA) complexes and TCRs. One of the main challenges in the field is finding the right pHLA target and the TCR that binds it. The development of in silico methods that can reduce the cost and time of laboratory research is the ‘holy grail’ of novel TCR therapies design. Molecular Dynamics (MD) simulation is a computational method allowing to gain a direct insight into the movements of protein complexes and giving the possibility to study the dynamics of the pHLA:TCR interaction. The combination of the MD and AI models helps us to increase the accuracy of the pHLA:TCR binding prediction. Methods: We conducted large-scale MD simulations of the crystal structures of 130 pHLA:TCR complexes available in the Protein Data Bank (PDB). Next, we analyzed the obtained trajectories in terms of formed bonds and other physico-chemical properties. This led us to postulate an MD model of interaction further used as an inductive bias for the AI binding prediction model, in which we applied deep learning on public single-cell pHLA:TCR datasets to estimate the probability of binding.In order to validate and improve our platform, we are building a pHLA:TCR interaction database with paired alpha and beta chain TCR sequences from a cohort of 100 colorectal cancer patients (NCT04994093). Results: We established the agretopicity and epitopicity of peptide residues within the pHLA:TCR systems based on the characteristics of the bonds formed between peptides and HLAs or TCRs in MD simulations. Additionally, the incorporation of other molecular properties, e.g. solvent accessible surface area, improved our AI pHLA:TCR model. In order to refine our results, we also account for the HLA type and physico-chemical properties of peptides. The introduction of the detailed TCR-peptide interaction matrix allowed the model to generalize to previously unseen pHLA targets. Conclusions: Rational design of effective TCR therapies can benefit from novel pHLA:TCR models for which the predictive accuracy is driven by the synergistic AI-MD approach. Citation Format: Alexander Myronov, Sławomir Stachura, Anna Sanecka-Duin, Oskar Gniewek, Łukasz Grochowalski, Maciej Jasiński, Paulina Król, Joanna Marczyńska-Grzelak, Piotr Skoczylas, Daniel Wojciechowski, Giovanni Mazzocco, Mikołaj Mizera, Jan Kaczmarczyk, Agnieszka Blum. Modeling pHLA:TCR interactions for effective TCR therapies: Leveraging AI and molecular dynamics [abstract]. In: Proceedings of the American Association for Cancer Research Annual Meeting 2022; 2022 Apr 8-13. Philadelphia (PA): AACR; Cancer Res 2022;82(12_Suppl):Abstract nr 2810.
更多查看译文
关键词
effective phlatcr therapies,phlatcr interactions,molecular dynamics
AI 理解论文
溯源树
样例
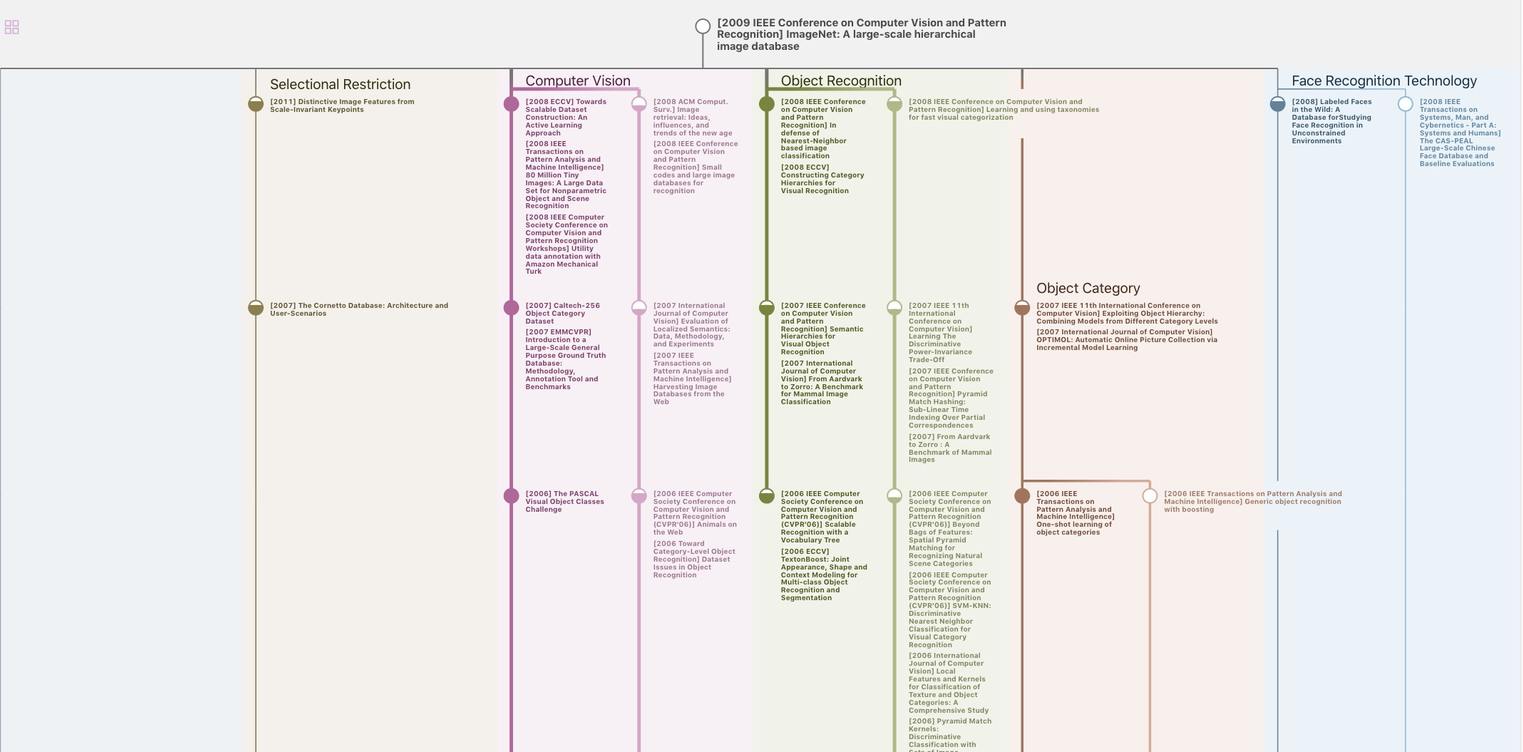
生成溯源树,研究论文发展脉络
Chat Paper
正在生成论文摘要