Taking ACTION: A Contemporary Prognostic Tool for Pediatric VAD Mortality
ASAIO Journal(2022)
摘要
Background: During the previous era of pediatric ventricular assist device (VAD) support, the Interagency Registry for Mechanically Assisted Circulatory Support (INTERMACS) profile served as a valuable tool for risk assessment. With the maturation of pediatric VAD field, however, it would be necessary to have a modern assessment system, given the increasing complexity of support strategies. We sought to develop a contemporary risk assessment tool to identify pediatric VAD patients at high risk for mortality on device using readily available pre-implantation clinical data. Methods: Data from the Advanced Cardiac Therapies Improving Outcomes Network (ACTION) registry on all patients supported with a VAD from 2012 to 2021 were included. Training (80%) and testing (20%) datasets were created randomly and potential risk factors for mortality were identified using multivariable logistic regression in the training dataset. A simplified risk prediction model was developed using an open-source, gradient-boosted decision tree machine learning library, known as Extreme Gradient Boosting (XGBoost). Predictive performance was assessed by the area under the receiver operating characteristic (ROC) curve in the testing dataset. Results: A total of 838 patients were included in this study with a median age of 5.7 years [IQR 0.78-15.0]. There were 113 deaths on device (13.5%). Nine significant risk factors at the time of VAD implant were included in the final predictive model (Table 1). ROC analysis of the final model demonstrated good discrimination with an area under the curve (AUC) of 0.95 (Figure 1). Summary: Our model predicts pediatric VAD mortality with good accuracy and provides a framework for establishing pediatric specific risk profiles that may help guide interventions, improve family counseling, and promote situational awareness among providers. TABLE. - Risk Factors Included in the Final Predictive Model Survival on DeviceN = 725 Death on DeviceN = 113 P-value* Odds Ratios(95% CI) Weight (kg); (Median [IQR]) 23.5 [8.4-58.0] 12.8 [5.4-54.9] <.001 0.99 (0.99-1.00) eGFR (Median [IQR]) 75.7 [55.5-104.3] 50.6 [29.2-74.3] <.001 0.98 (0.93-0.99) Diagnosis (%)CHDHCM 223 (30.8)4 (0.6) <.0010.022 <.0010.022 3.81 (2.52-5.75)4.92 (1.09-22.26) VAD Type (%)Paracorporeal Continuous Device 135 (18.6) 38 (33.6) <.001 2.21 (1.44-3.41) Pre-VAD Support (%)ECMO 148 (20.4) 44(38.9) <.001 2.49 (1.64-3.78) Dialysis/ RRTTPN 32 (4.4)329 (45.4) 15 (13.3)70 (62.0) <.0010.001 3.31 (1.73-6.34)1.96 (1.30-2.94) Mechanical ventilation Paralysis 326 (45.0)89 (12.3) 77 (68.1)26 (23.0) <.0010.002 2.62 (1.72-3.99)2.14 (1.31-3.49) eGFR- estimated glomerular filtration rate; CHD- congenital heart disease; HCM- hypertrophic cardiomyopathy; VAD- ventricular assist device; ECMO- extracorporeal membrane oxygenation; TPN- total parenteral nutrition; RRT-renal replacement therapy*Chi-square or Fisher’s exact test (F) for discrete variables; Kruskal–Wallis test for continuous variables.
更多查看译文
关键词
mortality,contemporary prognostic tool
AI 理解论文
溯源树
样例
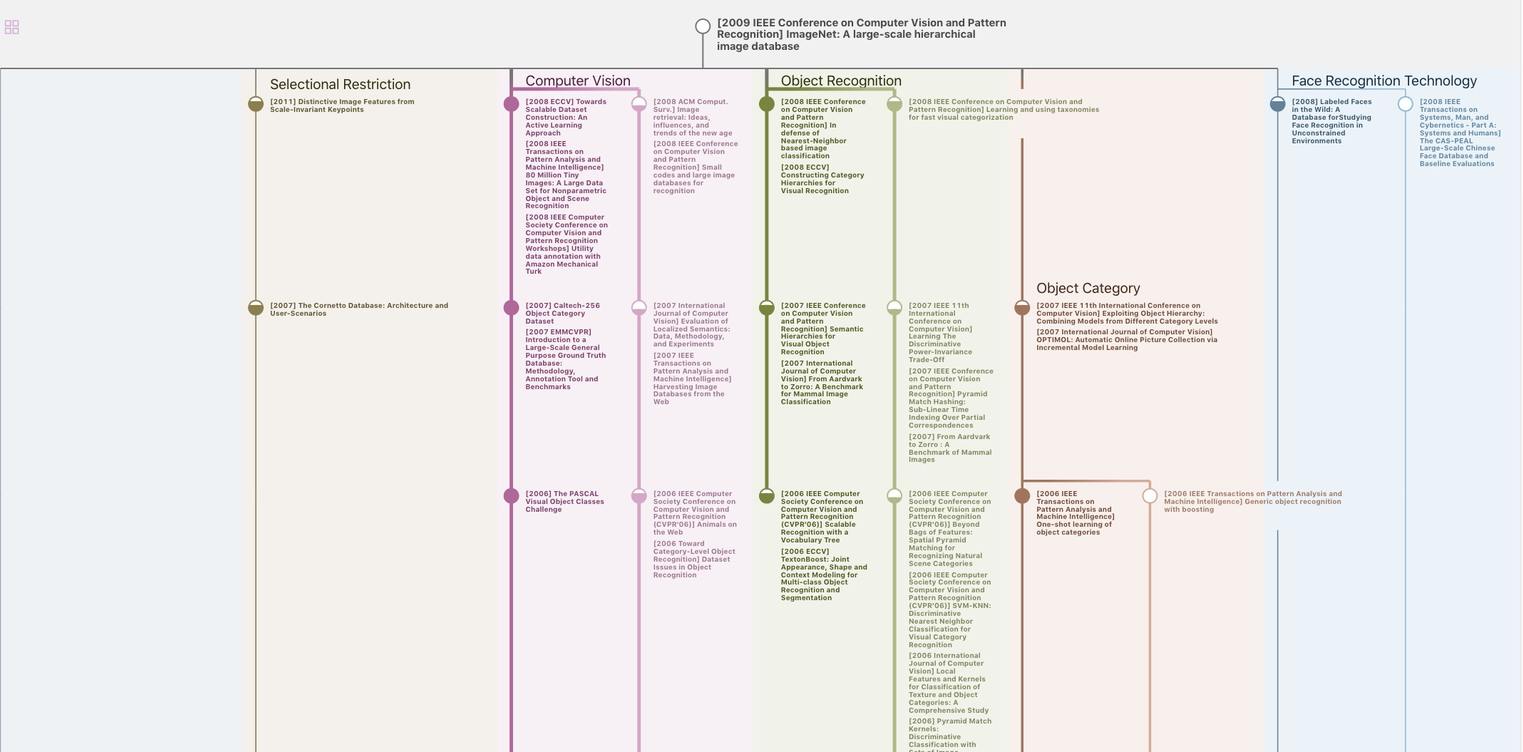
生成溯源树,研究论文发展脉络
Chat Paper
正在生成论文摘要