Climate change detection and attribution in extreme precipitation using compact representations 
crossref(2022)
摘要
<p>In the BMBF project climXtreme CoDEx, we analyze and explore methods for data compression to detect and attribute changes in the frequency and severity of extreme weather events with respect to climate change. Particularly for local processes on the atmospheric mesoscale, climate change signals are often masked by additional variability, resulting in poor signal-to-noise ratios. Appropriate reduction of degrees of freedom can improve the signal-to-noise ratio, increasing the potential to detect less strong signals in this setting. Our focus is on unsupervised learning approaches such as principal component analysis that are specifically designed for extremes.</p><p>We focus on extreme precipitation in Germany and analyze how different data compression techniques can be used in a detection and attribution (D&A) study. Among others, we use the approach of Cooley and Thibaud (2019), who decompose the pairwise dependence matrix as an analogue to the covariance matrix for extreme dependencies. We use principal component analysis and find an index that accounts for precipitation extremes due to common extremes in the first principal components. We use this index for a D&A study.</p><p>We further suggest that changes in extreme precipitation may depend on the spatial scale, e.g., when thinking about stratiform and convective precipitation events caused by different atmospheric conditions. Therefore, separate investigation of different event types might be necessary for robust and reliable D&A. This motivates our approach to filter different event types using the dual-tree wavelet transform, as previously applied by Buschow and Friederichs (2021). We propose a scale-dependent D&A study based on the filtered precipitation data.</p>
更多查看译文
AI 理解论文
溯源树
样例
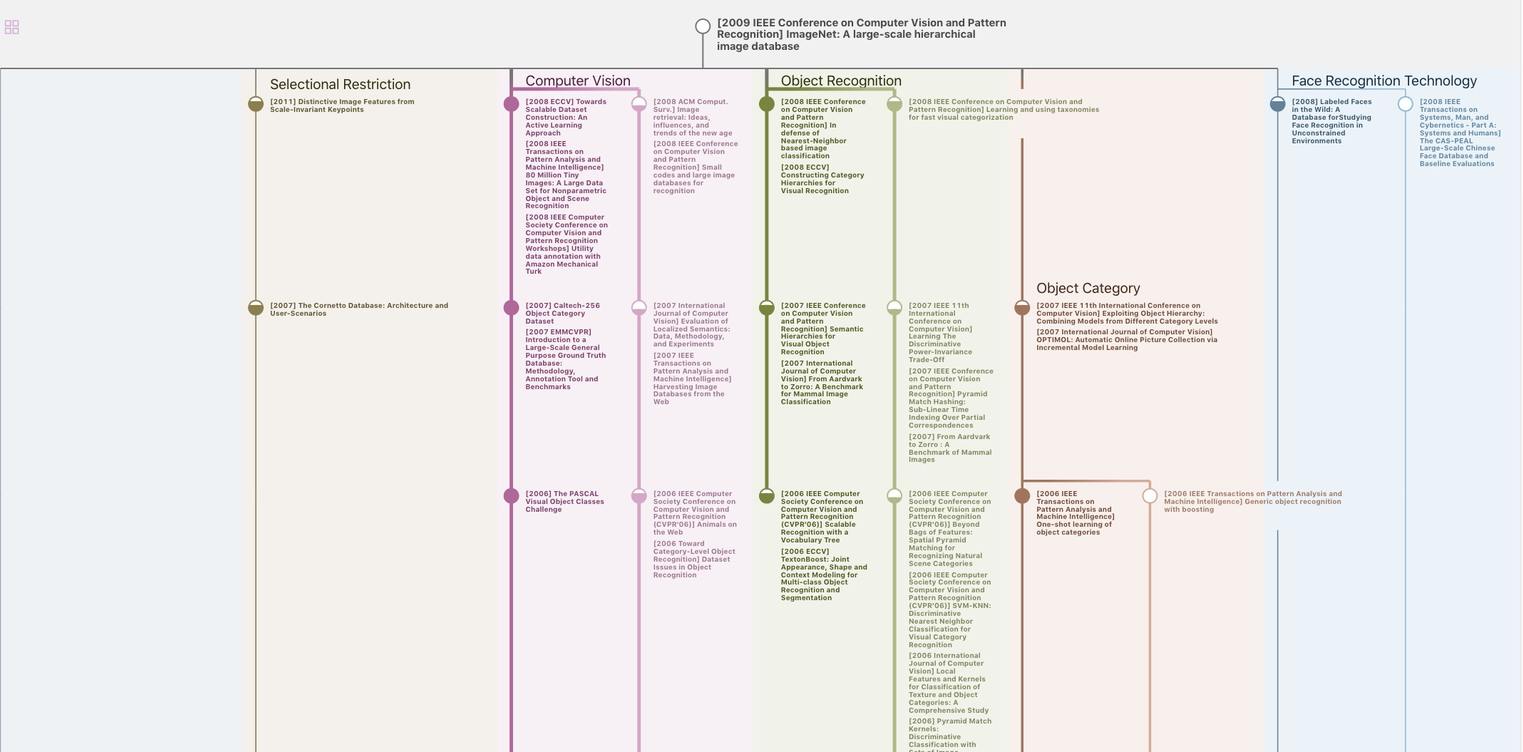
生成溯源树,研究论文发展脉络
Chat Paper
正在生成论文摘要