Evaluation of a Multi-Model Ensemble Prediction System
crossref(2022)
摘要
A multi-model ensemble prediction system that integrates UM (Unified Model) global and ensemble models, ECMWF global and ensemble models, and KIM (Korean Integrated Model) global model was established and its performance was evaluated. KIM is KMA’s new generation model which is based on the cubed sphere grid system and has 12km horizontal resolution and 91 vertical levels. It was developed over 9 years from 2011 to 2019 and operationally launched in April 2020.The optimal mean field was produced by weighted average of 5 models, where the weights were given inversely proportional to the error of each model.As a result of comparing RMSE (Root Mean Square Error) of the multi-model ensemble with 5 member models, a multiple-model ensemble showed better performance than any other member model. It was better than ECMWF ensemble model which showed the best performance among member models. In RMSE score, Mean Surface pressure was improved by 3.1% and 500hPa geopotential height was improved by 2.1% against ECMWF ensemble model. The performance of a multi-model ensemble by various weight calculation methods which are used for weighted averaging was compared. It was found that horizontally domain-averaged weight, having the different value for the forecast time gave the best performance. Giving the detailed and complex weights differently for each forecast time and each grid point rather degraded the performance of a multi-model ensemble.In BIAS verification, it was found that the BIAS of the multi-model ensemble showed the value between the maximum BIAS and the minimum BIAS of member models. Therefore, it was found that when the BIAS of the member models was distributed in a balanced positive and negative directions without being biased in one direction, the multi-model ensemble showed the smallest BIAS.
更多查看译文
AI 理解论文
溯源树
样例
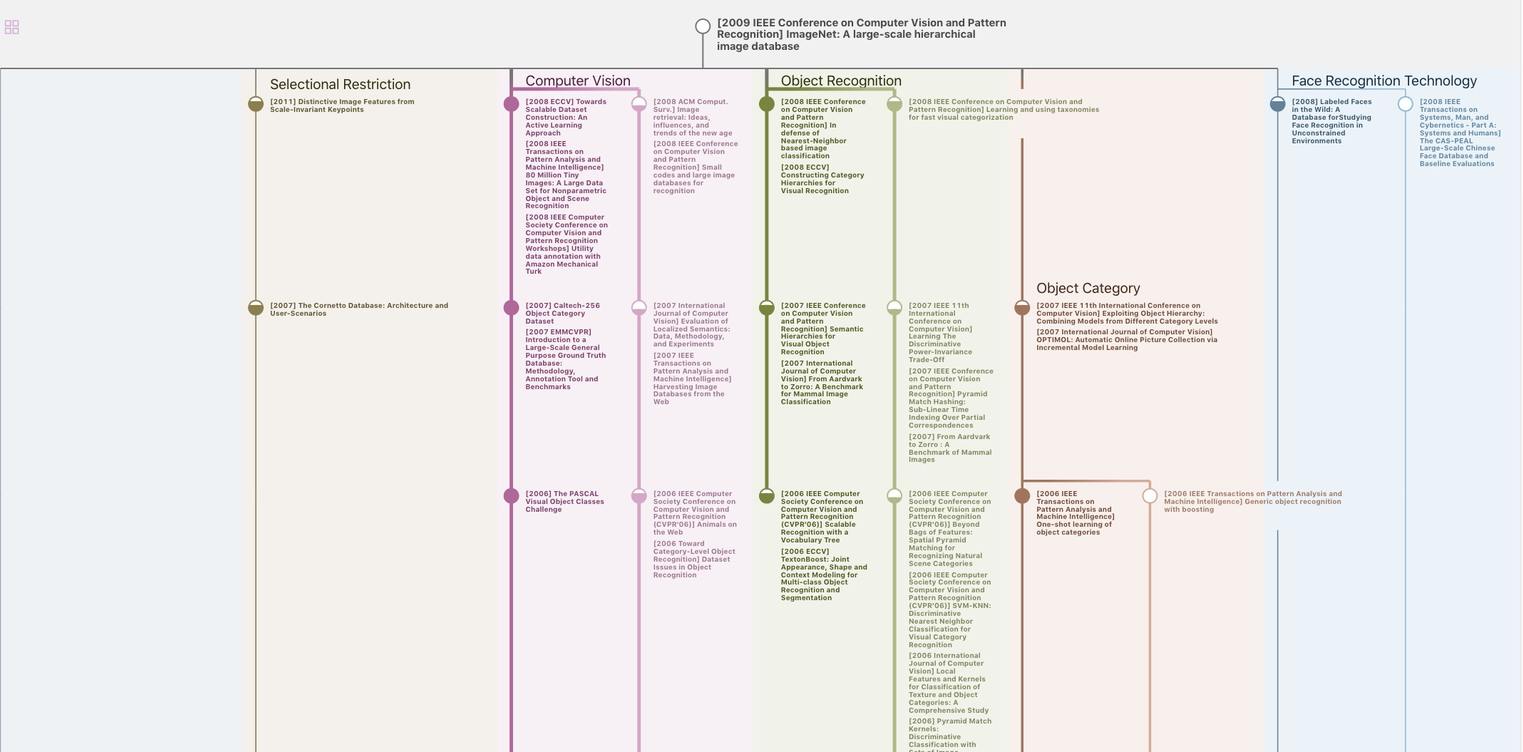
生成溯源树,研究论文发展脉络
Chat Paper
正在生成论文摘要