A Fully-Computational Approach for Quantifying the Transferability in Sleep Stage Scoring
SSRN Electronic Journal(2022)
摘要
Recently, many studies have proposed deep learning models for scoring sleep stages based on single-channel EEG, which is a promising method for remote sleep monitoring. However, two questions arise when ones want to apply these models to new datasets, especially wearable devices. First, when annotations on a target dataset are unavailable, which different data characteristics affect the scoring performance the most and by how much? Second, when the annotations become available, which dataset should be used as the source of transfer learning to maximize the scoring performance? In this paper, we propose a new means of computationally quantifying the impact that different data characteristics of datasets have on the scoring performance, and the transferability of a deep learning model. The quantification is done via training and evaluating models under various transfer configurations where the source and target dataset have different recording channels, recording environments, and subject conditions. For the first question, the environments and subject's conditions (~9.6-28.2%) had more impact on the performance than the channels (~5.0-5.9%). For the second question, the source datasets with EEG channels from the frontal and the central areas of the brain were more useful than the parietal and the occipital areas. The MASS-SS1 and MASS-SS5 datasets were the most useful transfer sources for the target datasets with the 30s and 20s epoch lengths. For the first time, this study quantifies the impact of different data characteristics between the training and the test set on the scoring performance and suggests a better transfer source.
更多查看译文
AI 理解论文
溯源树
样例
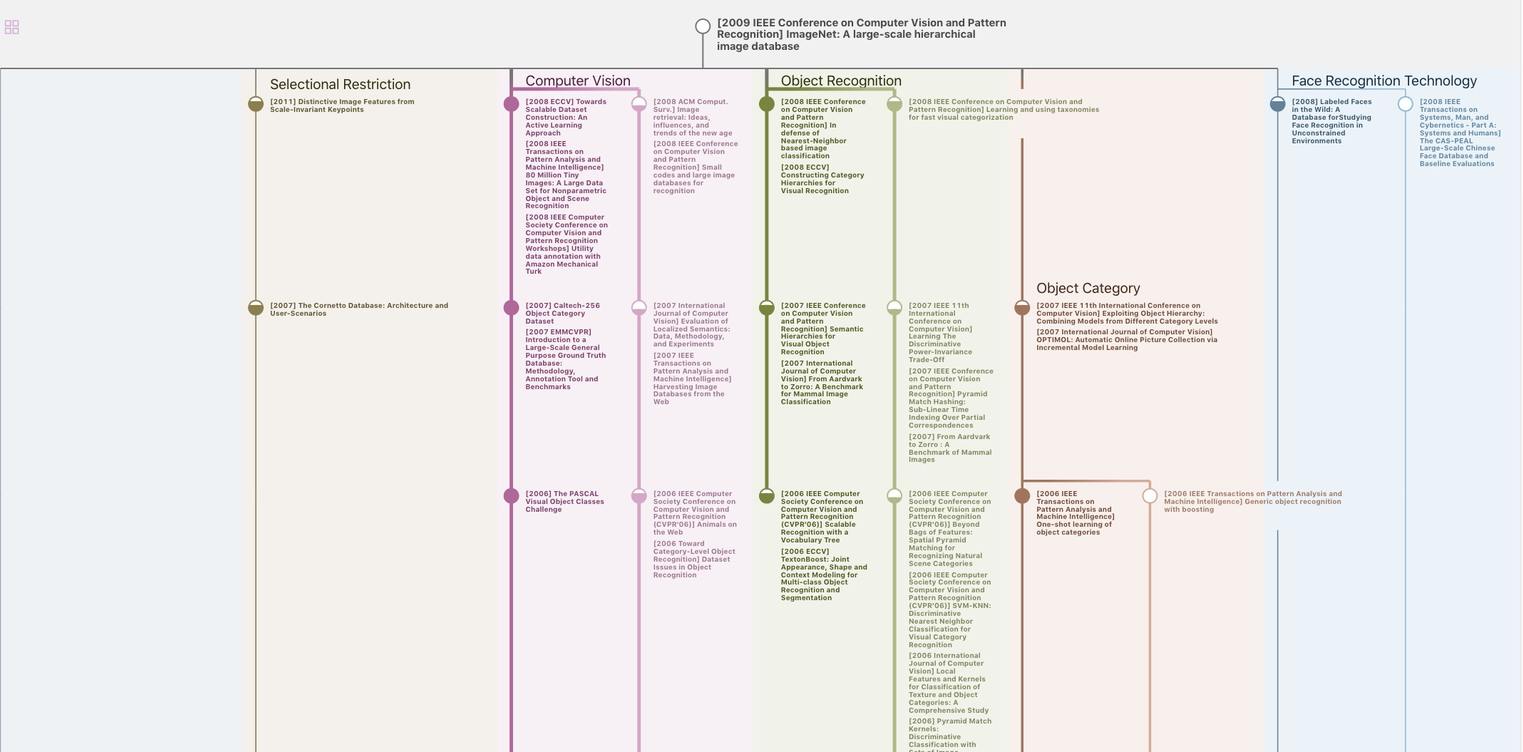
生成溯源树,研究论文发展脉络
Chat Paper
正在生成论文摘要