The LMR-SSIGN-MAPS model Predicts Disease-free Survival in Patients with Localized Clear Cell Renal Cell Carcinoma
Research Square (Research Square)(2022)
摘要
Abstract Background: To develop a comprehensive model for predicting disease-free survival (DFS) in patients with localized clear cell renal cell carcinoma (ccRCC). Methods: A retrospective analysis of 612 patients was performed between 2010 and 2015.The least absolute shrinkage and selection operator (LASSO) Cox regression analysis was performed to identify significant predictors. Furthermore, the multivariate Cox regression analysis was performed to reconfirm risk factors, then the prognosis model was constructed. The Harrell’s concordance index (C-index) was conducted to assess the model’s accuracy for predicting DFS in localized ccRCC.Results: The lymphocyte-to-monocyte ratio (LMR) (HR: 1.04, P = 0.009), the Mayo Clinic stage, size, grade, and necrosis score (SSIGN) (HR: 1.36, P < 0.001) and Mayo adhesive probability score (MAPS) (HR: 1.99, P<0.001) were the significant risk factors screened by LASSO Cox regression and reconfirmed by multivariate Cox regression analysis in 44 variables. The LMR-SSIGN-MAPS model was constructed by combining the LMR, SSIGN, and MAPS. In the training and validation cohorts, the Harrell’s C-index was larger for the LMR-SSIGN-MAPS model (0.854, 0.848) than the SSIGN score (0.782, 0.772). The Kaplan-Meier survival analysis demonstrated the significant association between higher LMR-SSIGN-MAPS score and poorer DFS (P < 0.001) in the overall cohorts.Conclusions: The LMR-SSIGN-MAPS model which consists of preoperative inflammation biomarkers, perinephric adipose tissue image-based scoring system, and pathological features showed the strengths of easy-to-use and high predictability and might be also used as a promising prognosis model in predicting DFS for patients with localized ccRCC.
更多查看译文
关键词
carcinoma,lmr-ssign-maps,disease-free
AI 理解论文
溯源树
样例
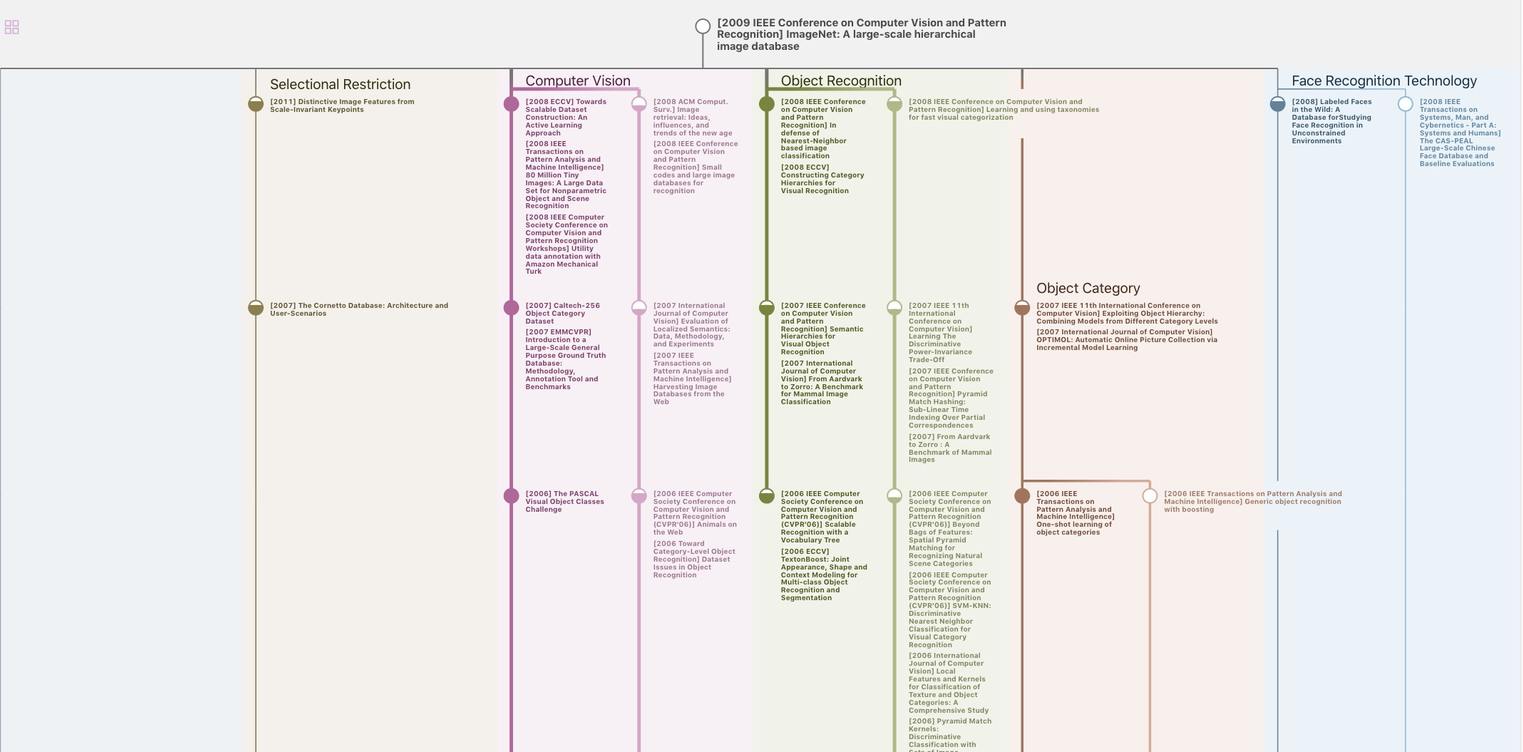
生成溯源树,研究论文发展脉络
Chat Paper
正在生成论文摘要