Inequality-Constrained 3D Morphable Face Model Fitting
IEEE TRANSACTIONS ON PATTERN ANALYSIS AND MACHINE INTELLIGENCE(2024)
摘要
3D morphable model (3DMM) fitting on 2D data is traditionally done via unconstrained optimization with regularization terms to ensure that the result is a plausible face shape and is consistent with a set of 2D landmarks. This paper presents inequality-constrained 3DMM fitting as the first alternative to regularization in optimization-based 3DMM fitting. Inequality constraints on the 3DMM's shape coefficients ensure face-like shapes without modifying the objective function for smoothness, thus allowing for more flexibility to capture person-specific shape details. Moreover, inequality constraints on landmarks increase robustness in a way that does not require per-image tuning. We show that the proposed method stands out with its ability to estimate person-specific face shapes by jointly fitting a 3DMM to multiple frames of a person. Further, when used with a robust objective function, namely gradient correlation, the method can work "in-the-wild" even with a 3DMM constructed from controlled data. Lastly, we show how to use the log-barrier method to efficiently implement the method. To our knowledge, we present the first 3DMM fitting framework that requires no learning yet is accurate, robust, and efficient. The absence of learning enables a generic solution that allows flexibility in the input image size, interchangeable morphable models, and incorporation of camera matrix.
更多查看译文
关键词
3D reconstruction,3D shape estimation,analysis-by-synthesis,face analysis,face reconstruction,morphable models
AI 理解论文
溯源树
样例
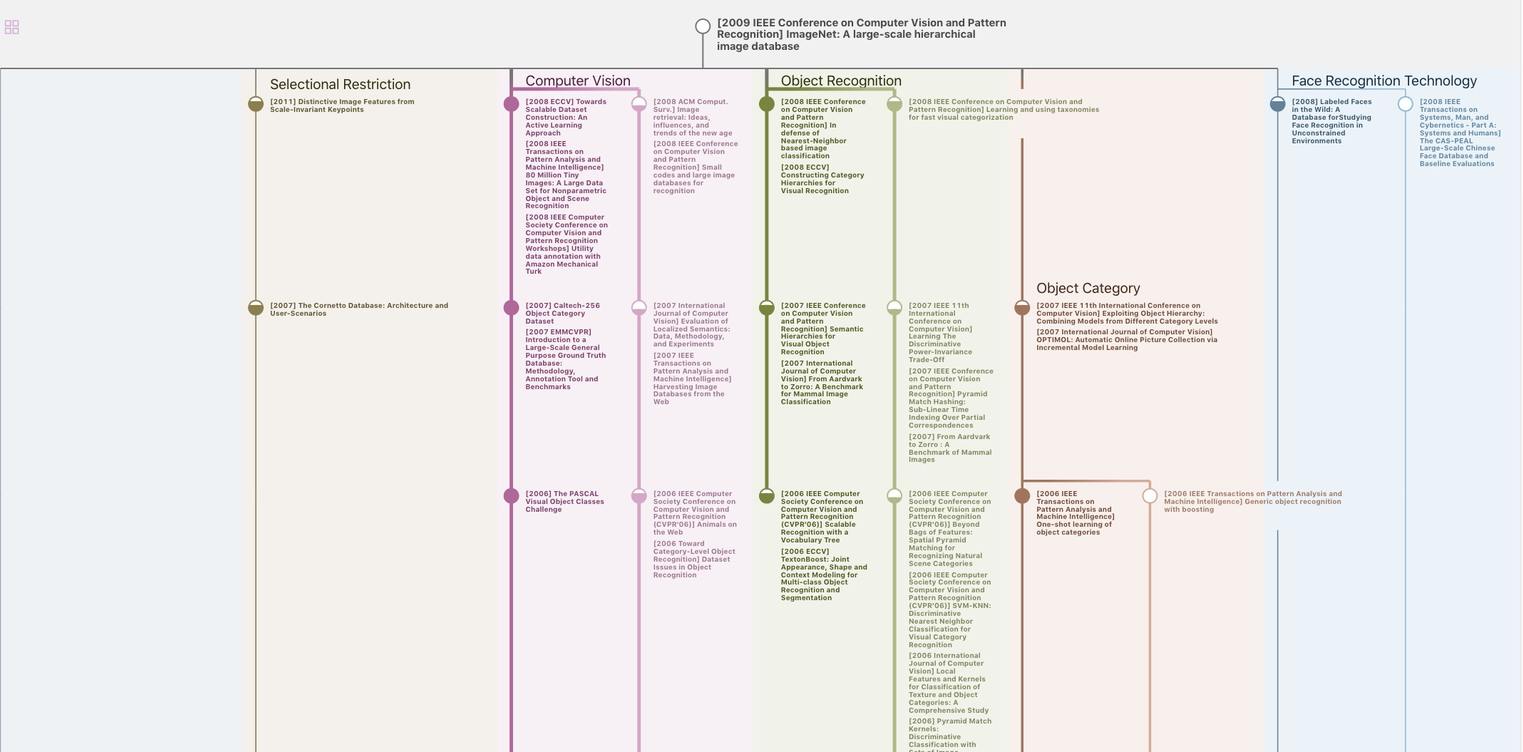
生成溯源树,研究论文发展脉络
Chat Paper
正在生成论文摘要