Deep learning model for predicting the presence of stromal invasion of breast cancer on digital breast tomosynthesis
Research Square (Research Square)(2022)
摘要
Abstract Purpose:To develop a deep learning (DL)-based algorithm to predict the presence of stromal invasion of breast cancer on digital breast tomosynthesis (DBT).Materials and Methods:The institutional review board approved this retrospective study and waived the requisite to obtain the informed consent from the patients. Initially, 499 patients (mean age of 50.5 years, ranging from 29 to 90 years) who were referred to our hospital suggestive of breast cancer and performed DBT between March 1, 2019 and August 31, 2019, were enrolled in this study. Out of the 499 patients, 140 patients who were performed surgery with diagnosed breast cancer were finally selected. Based on the pathological reports, 140 patients were divided to be categorized as 20 patients with non-invasive cancer and 120 patients with invasive cancer. Xception architecture was used for the DL model to differentiate non-invasive cancer and invasive cancer. Diagnostic performance of the DL model was assessed by accuracy, sensitivity, specificity, and areas under the receiver operating characteristic curve (AUC).Results:The average accuracy, sensitivity, specificity, and AUC were 0.897, 0.909, 0.758, and 0.749, respectively.Conclusion:The proposed DL model trained on DBT images is useful to predict the presence of stromal invasion of breast cancer.Secondary abstractThe proposed deep learning (DL) model trained on digital breast tomosynthesis (DBT) images is useful to predict the presence of stromal invasion of breast cancer.
更多查看译文
关键词
breast cancer,deep learning model,deep learning,stromal invasion
AI 理解论文
溯源树
样例
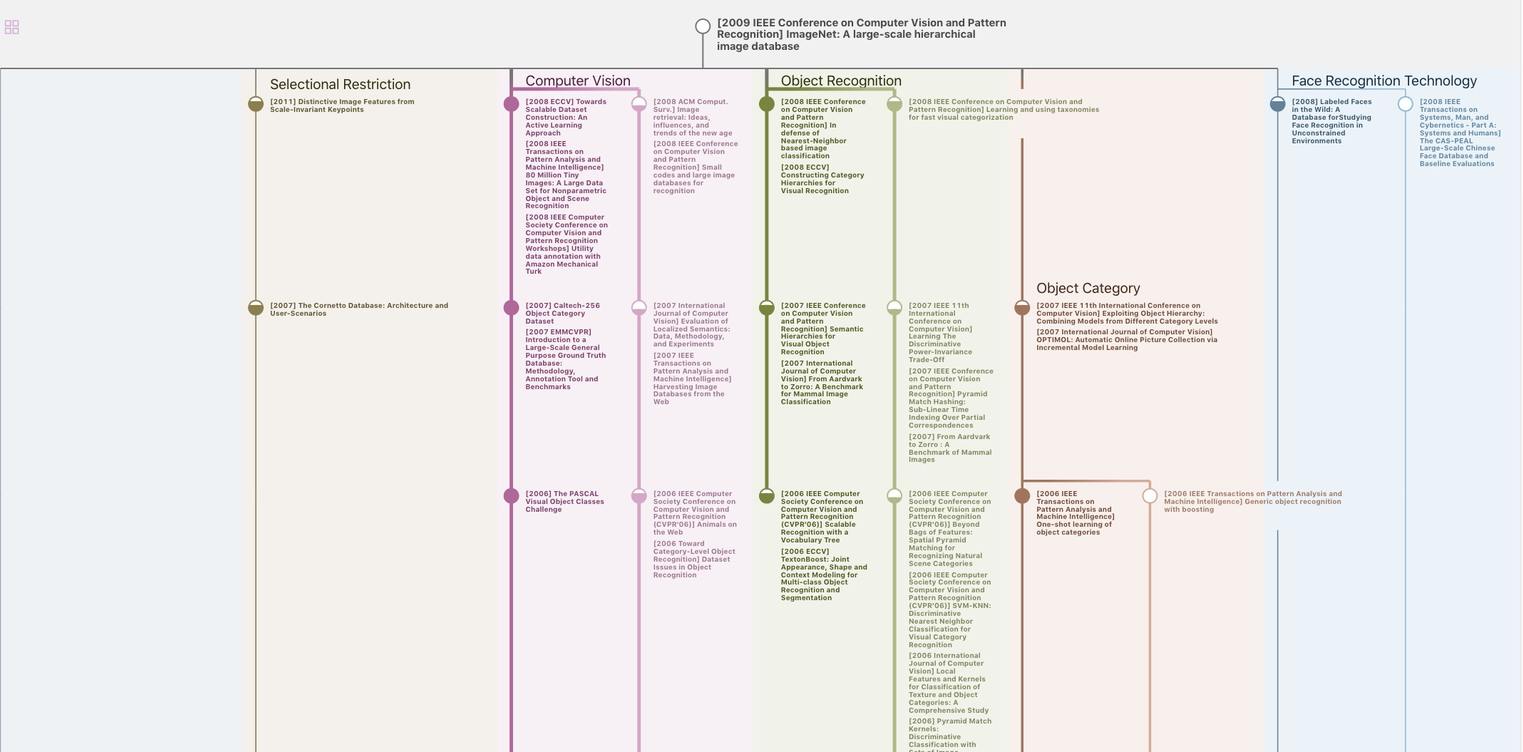
生成溯源树,研究论文发展脉络
Chat Paper
正在生成论文摘要