Benchmarking Polyp Segmentation Methods in Narrow-Band Imaging Colonoscopy Images
IEEE Journal of Biomedical and Health Informatics(2023)
摘要
In recent years, there has been significant progress in polyp segmentation in white-light imaging (WLI) colonoscopy images, particularly with methods based on deep learning (DL). However, little attention has been paid to the reliability of these methods in narrow-band imaging (NBI) data. NBI improves visibility of blood vessels and helps physicians observe complex polyps more easily than WLI, but NBI images often include polyps with small/flat appearances, background interference, and camouflage properties, making polyp segmentation a challenging task. This paper proposes a new polyp segmentation dataset (PS-NBI2K) consisting of 2,000 NBI colonoscopy images with pixel-wise annotations, and presents benchmarking results and analyses for 24 recently reported DL-based polyp segmentation methods on PS-NBI2K. The results show that existing methods struggle to locate polyps with smaller sizes and stronger interference, and that extracting both local and global features improves performance. There is also a trade-off between effectiveness and efficiency, and most methods cannot achieve the best results in both areas simultaneously. This work highlights potential directions for designing DL-based polyp segmentation methods in NBI colonoscopy images, and the release of PS-NBI2K aims to drive further development in this field.
更多查看译文
关键词
Polyp segmentation,narrow-band imaging,colonoscopy images,deep learning,benchmark
AI 理解论文
溯源树
样例
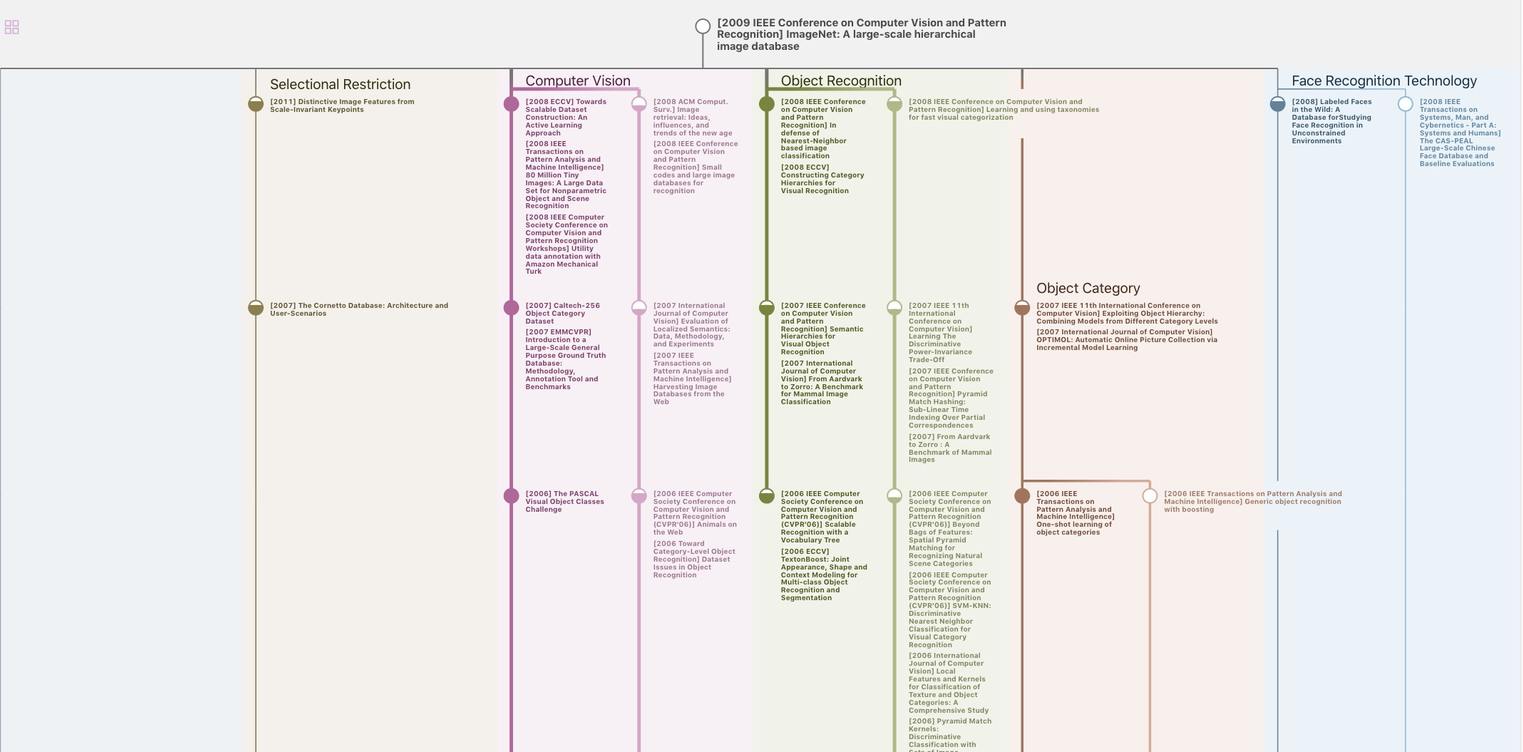
生成溯源树,研究论文发展脉络
Chat Paper
正在生成论文摘要