Plantorgan hunter: a deep learning-based framework for quantitative profiling plant subcellular morphology
crossref(2022)
摘要
Abstract Accurate delineation of plant cell organelles from electron microscope images is essential to understand subcellular behaviors and functions. Here, we develop a deep learning pipeline, organelle segmentation network (OrgSegNet) for pixel-wise segmentation to identify chloroplasts, mitochondria, nuclei, and vacuoles. OrgSegNet was evaluated on a large manually-annotated dataset of 6371 organelles collected from 13 plant species, and achieved a state-of-the-art segmentation performance of these organelles. To generalize the morphological characteristics of plant organelles, we defined three morphological metrics (shape-complexity, electron-density, and area), and released an open-source web tool “Plantorgan Hunter” allowing quantitative profiling of subcellular morphology. The functionalities of Plantorgan Hunter can be easily operated, and we believe that it will increase the efficiency and productivity of plant subcellular morphological characteristics for the plant science community.
更多查看译文
AI 理解论文
溯源树
样例
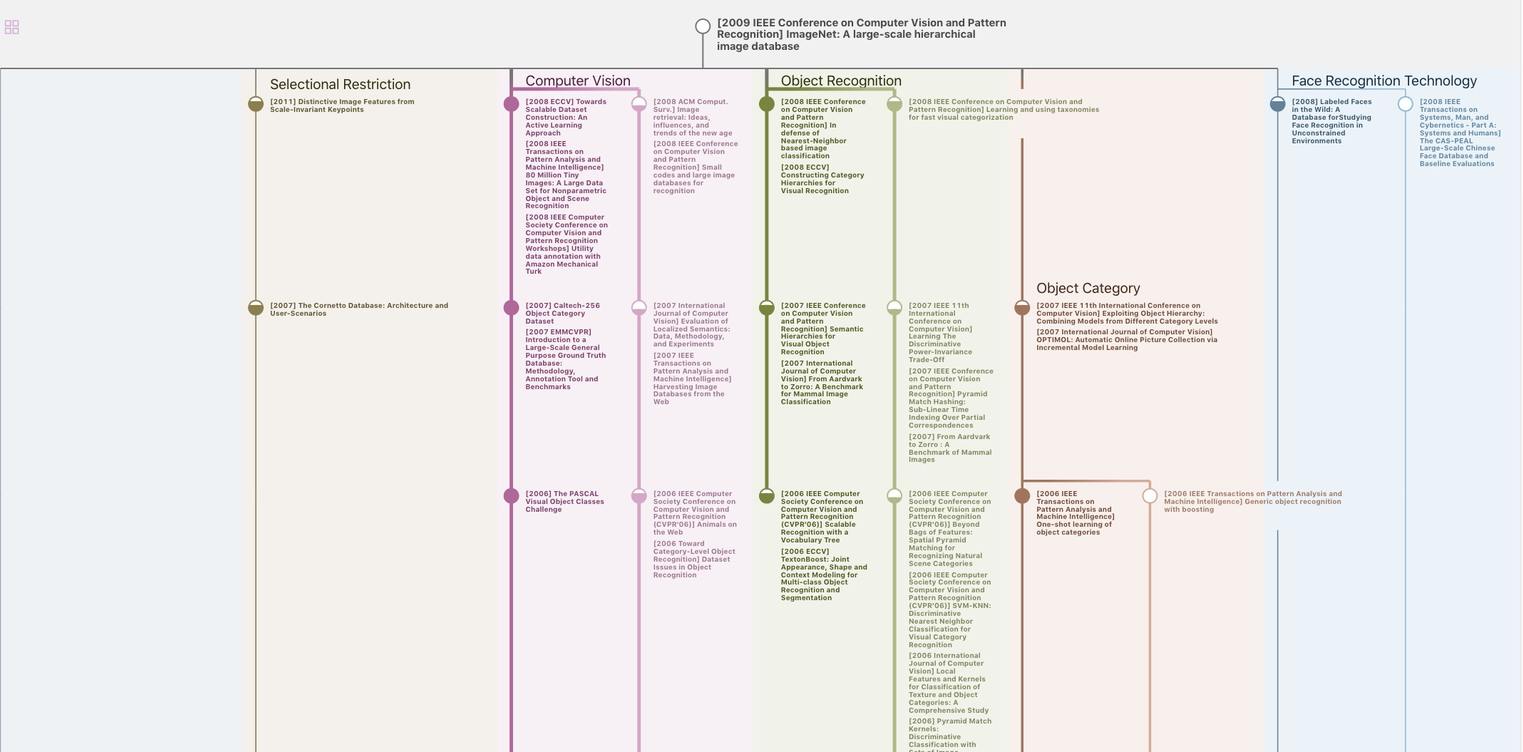
生成溯源树,研究论文发展脉络
Chat Paper
正在生成论文摘要