Properties of Standard and Sketched Kernel Fisher Discriminant
IEEE Transactions on Pattern Analysis and Machine Intelligence(2023)
摘要
Kernel Fisher discriminant (KFD) is a popular tool as a nonlinear extension of Fisher's linear discriminant, based on the use of the kernel trick. However, its asymptotic properties are still rarely studied. We first present an operator-theoretical formulation of KFD which elucidates the population target of the estimation problem. Convergence of the KFD solution to its population target is then established. However, the complexity of finding the solution poses significant challenges when
$n$
is large and we further propose a sketched estimation approach based on a
$m\times n$
sketching matrix which possesses the same asymptotic properties (in terms of convergence rate) even when
$m$
is much smaller than
$n$
. Some numerical results are presented to illustrate the performances of the sketched estimator.
更多查看译文
关键词
Kernel method,random projection,reproducing kernel hilbert space,variance operator
AI 理解论文
溯源树
样例
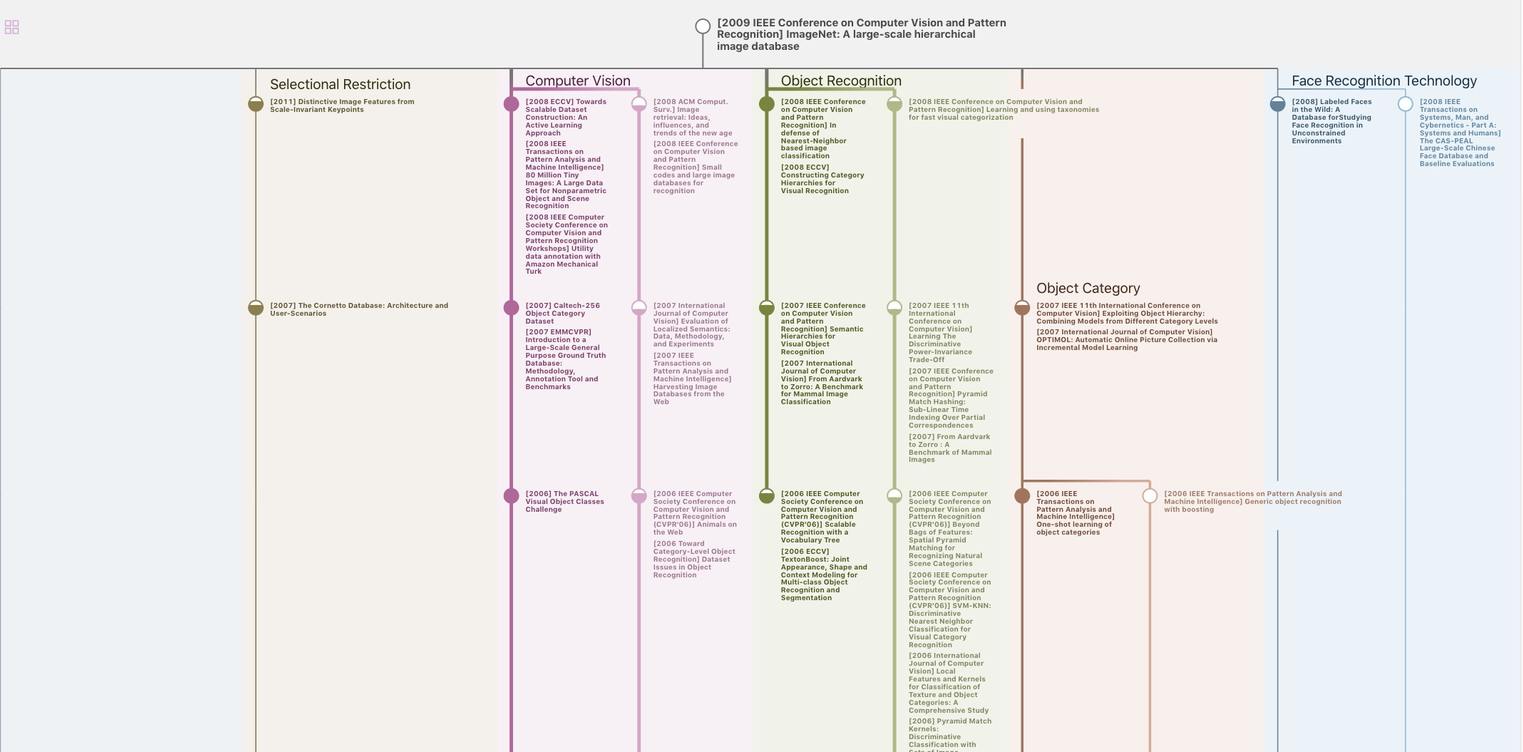
生成溯源树,研究论文发展脉络
Chat Paper
正在生成论文摘要