Predicting risky sexual behavior among college students through machine learning approaches: Analysis of individual data from 1264 universities in 31 provinces in China (Preprint)
crossref(2022)
摘要
BACKGROUND Risky sexual behavior (RSB), as the most direct risk factor for sexually transmitted infections (STIs), is common among college students. Thus, it is important to intervene and prevent it among college students by identifying relevant risk factors and making predictions. OBJECTIVE We aimed to establish a predictive model for RSB among college students to facilitate timely prevention and intervention before contraction of STIs. METHODS We included a total of 8,290 self-reported heterosexual Chinese students with sexual intercourse experience from November 2019 to February 2020. We identified RSB among those students and attributed it to four dimensions: whether contraception was used; whether the contraceptive method was safe; whether students engaged in casual sex or sex with multiple partners; and integrated RSB, which combined the first three dimensions. For each type, we compared various machine learning (ML) models according to multiple validation indicators and chose the optimal model for both RSB prediction and risk factor identification. RESULTS In total, 4993 (60·2%) students had ever engaged in RSB. Among them, 3422 (41·3%) did not use contraception every time they had sexual intercourse, 3393 (40·93%) had ever used an unsafe contraceptive method, and 1069 (12·9%) had casual sex or sex with multiple partners. Through comparison, the XGBoost (XGB) and gradient boosting machine (GBM) models achieved the optimal predictive performance on integrated RSB, with an area under the receiver operator characteristic curve (AUC) reaching 0·80. Under the condition of ensuring the stability of various validation indicators, the 12 most predictive variables were finally selected by XGB, including participants’ relationship status, sexual knowledge, sexual attitude, and previous sexual experience. CONCLUSIONS RSB is prevalent among college students, and ML is an effective approach to predict RSB and identify corresponding risk factors.
更多查看译文
AI 理解论文
溯源树
样例
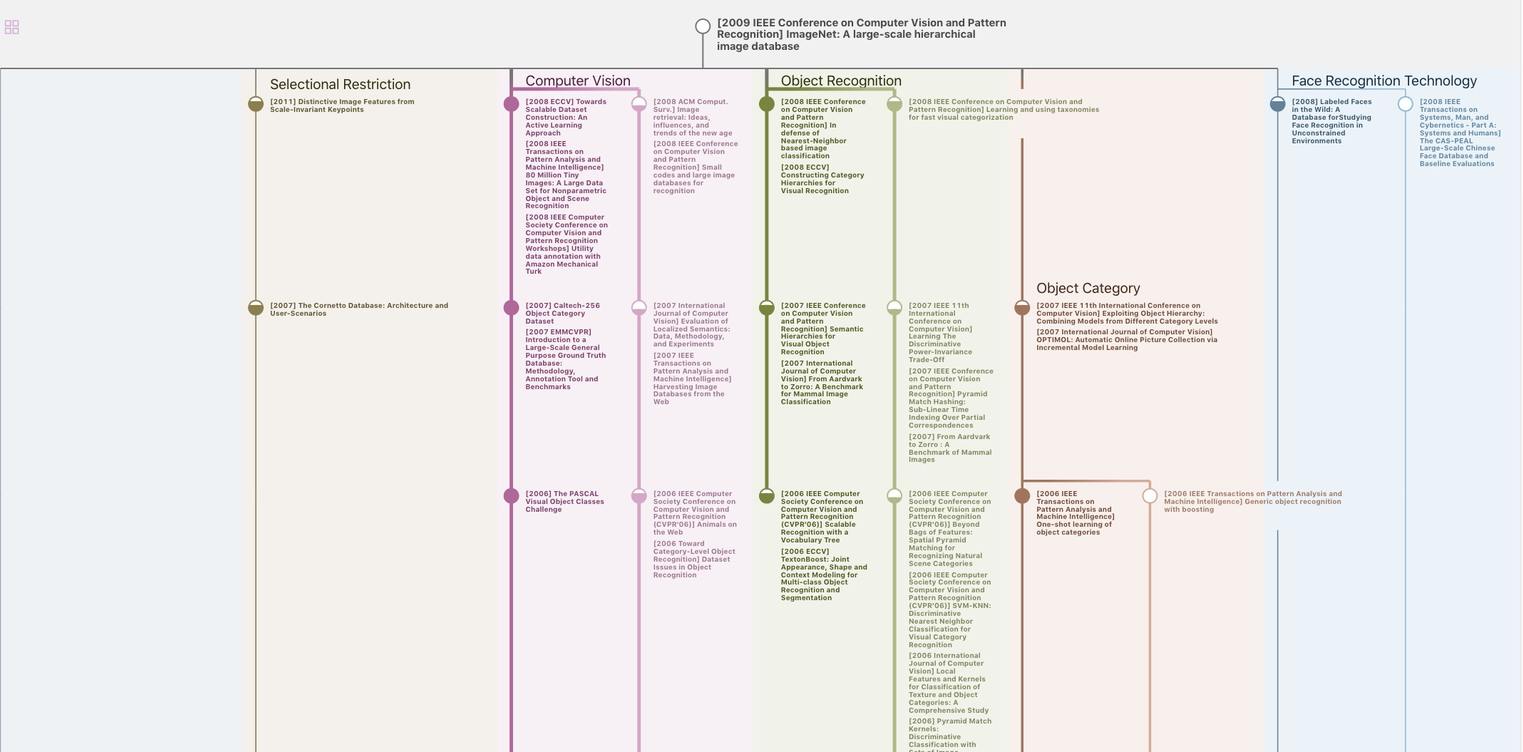
生成溯源树,研究论文发展脉络
Chat Paper
正在生成论文摘要