Deep convolutional neural networks for filtering out normal frames in reviewing wireless capsule endoscopy videos
Research Square (Research Square)(2022)
摘要
Abstract Wireless capsule endoscopy (WCE) has provided us with the ability to non-invasively visualize the gastrointestinal (GI) tract with high quality. This work presents an assistant diagnostic system to accelerate the costly and time-consuming process of manually evaluating captured videos. Due to the fact that the major portion of WCE frames is Normal, a diagnostic model with a high negative predictive value (NPV) is desired and is highly demanded by gastroenterologists. In this study, we have proposed six deep convolutional neural networks (DCNNs) to satisfy the need for high classification accuracy and high NPV. The proposed architectures consist of a convolutional part coming from some well-known trained networks and a specifically designed classifier part. VG_BFCG exhibited the best classification performance (test_acc:0.967, NPV: 0.989). As a contribution, we realized that the proposed architectures could avoid mislabeling new pathologies not included in the training dataset, which implies the generalization of NPV. These new pathologies are the most common GI pathologies in patients including Ulcer, Erosion, Polyp, Blood, Erythematous, and Lymphoid hyperplasia, which are presented to the proposed networks in order to assess the generalization accuracy of the Normal class. DN_BFCG exhibited the best generalization accuracy (>0.99) for all new pathologies proving that the decision boundary obtained for Normal class is robust and generalizable.
更多查看译文
关键词
deep convolutional neural networks,videos,normal frames,neural networks
AI 理解论文
溯源树
样例
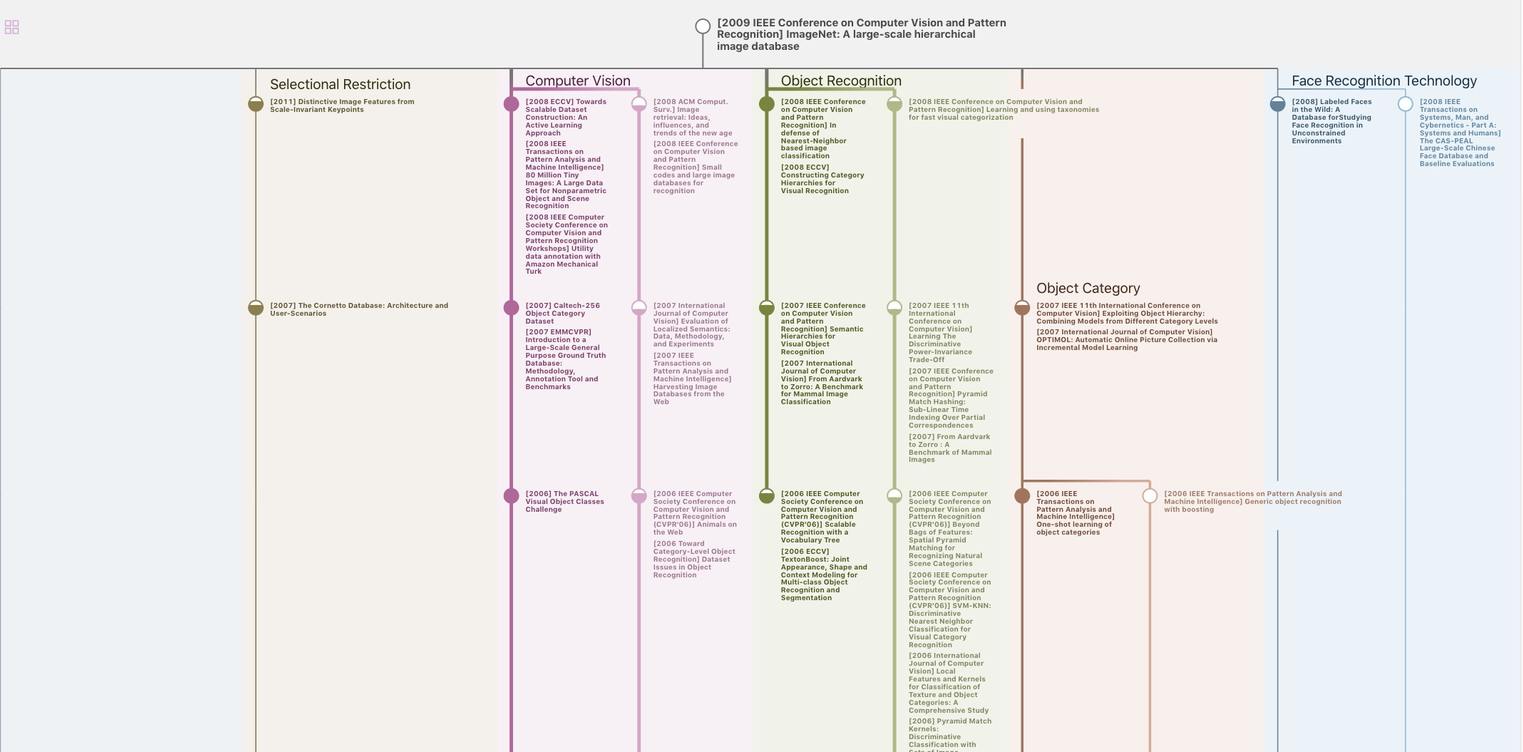
生成溯源树,研究论文发展脉络
Chat Paper
正在生成论文摘要