Using machine learning algorithms to identify predictors of social vulnerability in the event of an earthquake: Istanbul case study
crossref(2022)
Abstract
Abstract. For an effective disaster risk mitigation plan and for building a society more resilient to natural disasters, it is essential to understand the factors that are related to social vulnerability as an important dimension to social risk. This study aims to identify the associations between socio-economic and socio-demographic household characteristics and earthquake related social vulnerability using survey data collected from 41,093 households in Istanbul. Machine learning models, namely: logistic regression, classification tree, random forest, support vector machine, naive bayes, artificial neural network, and K-nearest neighbours, were employed to classify households according to their social vulnerability status. Due to the disparity of class size for the outcome variable, subsampling strategies were applied for dealing with imbalanced data. Artificial Neural Network (ANN) was found to have the optimal predictive performance when random majority under sampling was applied (AUC: 0.813). The results from the ANN method indicated that not having social security, living in a squatter house and having high risk of job loss after an earthquake were among the most important predictors for increasing social vulnerability risk. Additionally, the level of education, the ratio of elderly persons in the household, owning a property, household size, ratio of income earners, and having savings were associated with vulnerability. An open access R-shiny web application was developed to visually display the performance of ML methods, important variables for the social vulnerability risk classification and the spatial distribution of the variables across Istanbul neighbourhoods. The machine learning methodology and the findings that we present in this paper can serve as a guidance for decision makers in identifying and prioritising action towards target groups to reduce their vulnerability risk prior to earthquakes.
MoreTranslated text
AI Read Science
Must-Reading Tree
Example
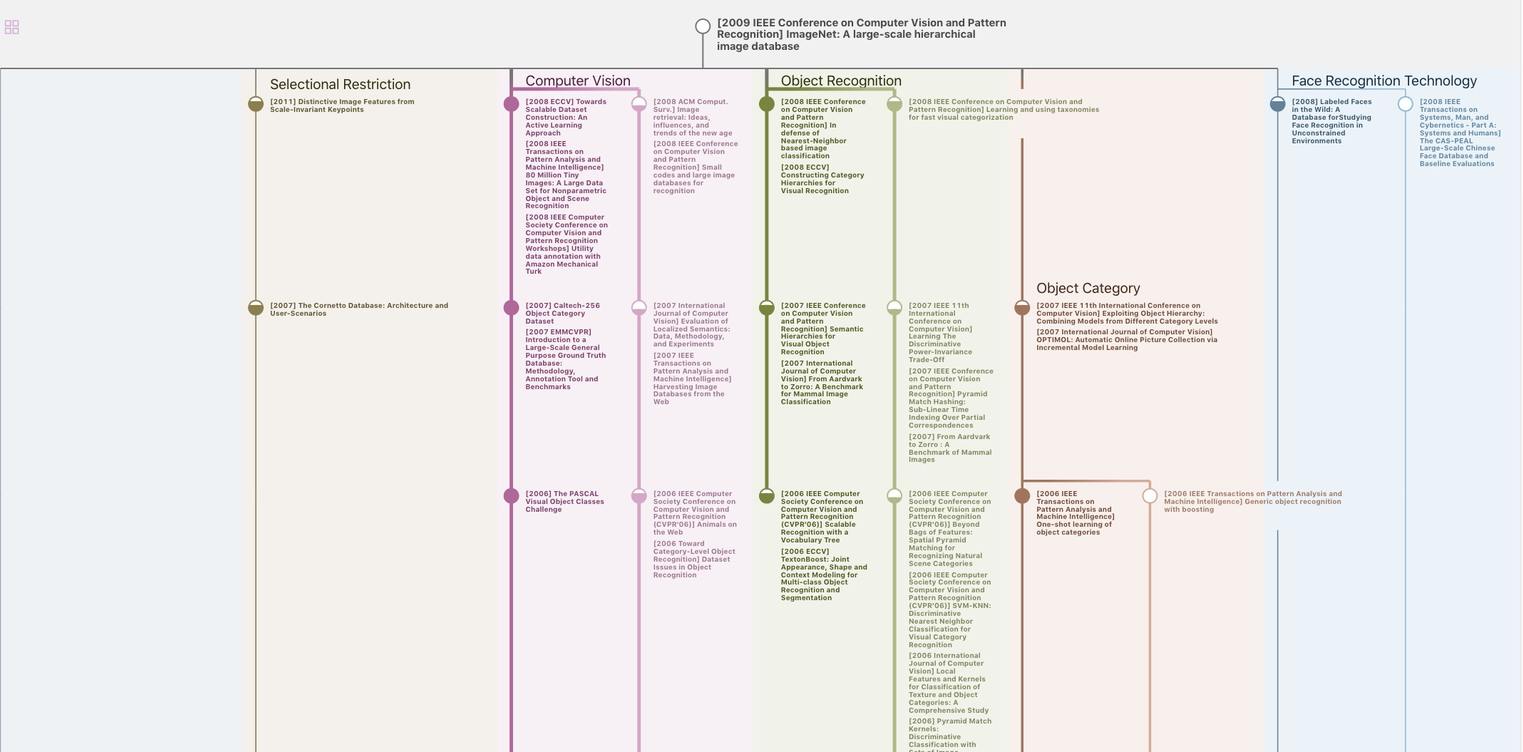
Generate MRT to find the research sequence of this paper
Chat Paper
Summary is being generated by the instructions you defined