Cardiotocography-dependent experimental comparison between artificial intelligence and human judgement of fetal asphyxia during delivery
crossref(2022)
摘要
Abstract Cardiotocography (CTG) has been widely used to promote newborn prognosis and has saved a vast number of pregnancies worldwide. The efficacy of this method which depends on real-time diagnosis has not changed for half a century. However, the establishment the computational diagnosis method of CTG with Artificial intelligence (AI) had been required to prevent misinterpretation and lessness of medical resource. Experimental and retrospective studies to estimate the efficiency of AI diagnosis and how it contributes to human diagnosis have thus far only been implemented using small cohorts. The usage of previously developed machine learning (ML) and deep learning (DL) methods has generated areas under the curve (AUC) of 0.730 and 0.949, respectively. In this study, diagnoses based on human judgement were collected from obstetricians and midwives. Neither ML nor DL could reach the high performance of humans. However, an aggregation analysis model mimicking the corroboration of newborn asphyxia predictions by human judgment and DL showed higher specificity and accuracy, though the AUC of receiver operating characteristics analyses were still lower than those with humans alone. While further data accumulation and analyses are necessary to increase the accuracy of these methods, they bear the promise of constituting a helpful tool for the safe management of delivery.
更多查看译文
AI 理解论文
溯源树
样例
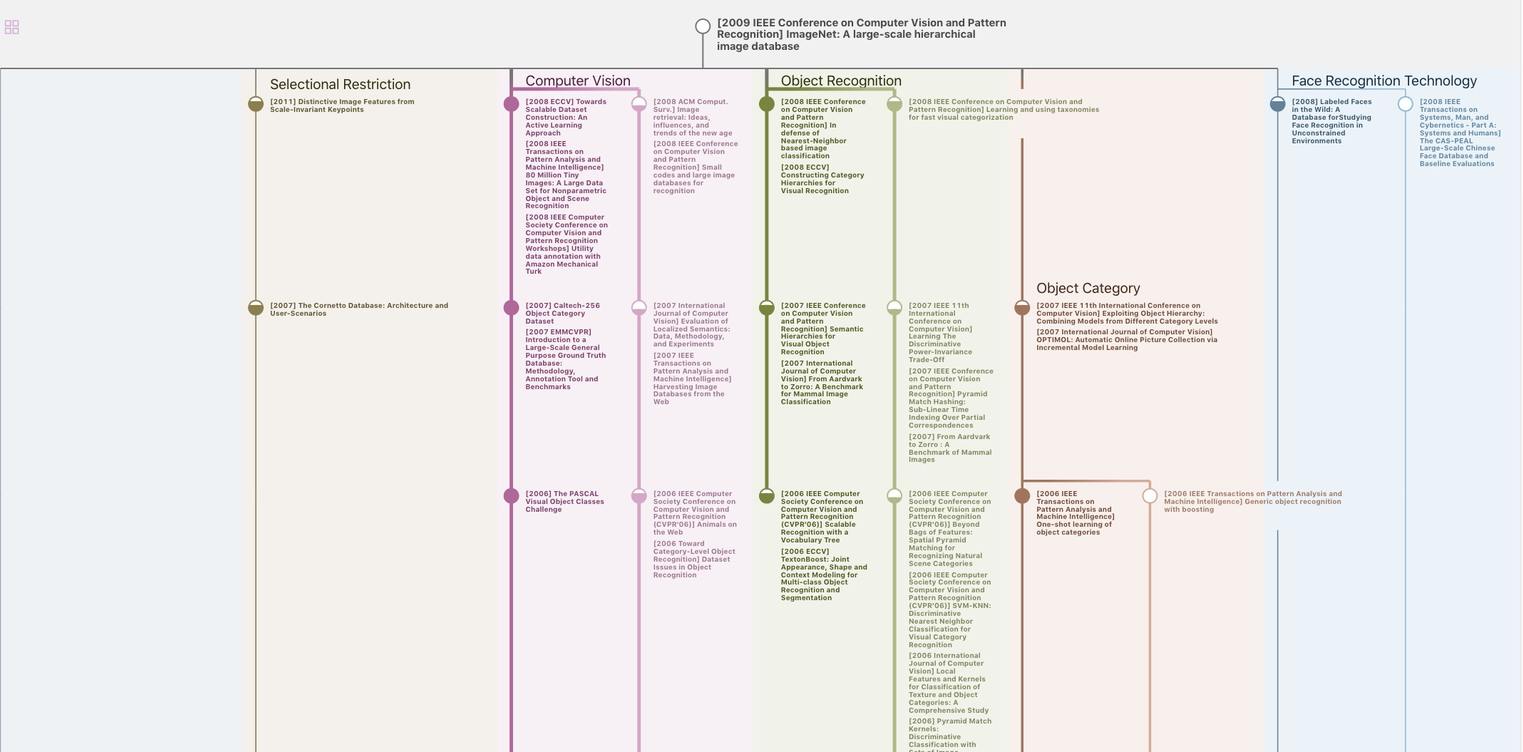
生成溯源树,研究论文发展脉络
Chat Paper
正在生成论文摘要