Three-round learning strategy based on 3D Deep Convolutional GANs for Alzheimer’s disease staging
Research Square (Research Square)(2022)
摘要
Abstract The accurate diagnosis of Alzheimer's disease (AD) and its early stage is essential for early dementia detection and treatment planning. For 2D Convolutional neural networks (CNNs), the networks pre-trained on ImageNet always outperformed the models trained from scratch. 3D CNN is easily to lead to an overfitting problem due to the lack of 3D neuroimage datasets. To alleviate the overfitting caused by small to medium-sized datasets, a three-round learning strategy based on 3D Deep Convolutional Generative Adversarial Networks (DCGAN) is used for staging the spectrum of AD. In the first round, the common features of sMRI were trained in the context of 3D DCGAN by unsupervised learning. In the second round, the pre-trained discriminator (D) of the DCGAN with residual architecture was transferred and fine-tuned for the classification task between AD and cognitive normal (CN). In the last round of the transfer learning approach, the weights learned in the AD versus CN classification task were transferred to MCI diagnosis. The proposed model attained accuracies of 92.8 %, 78.1 %, and 76.4 % in the classification of AD versus CN, AD versus MCI, and MCI versus CN, respectively. The experimental results demonstrate that our proposed model has a good classification performance on staging the AD spectrum with small to medium-sized datasets, compared with other state-of-the-art studies.
更多查看译文
关键词
3d deep convolutional gans,alzheimers,three-round
AI 理解论文
溯源树
样例
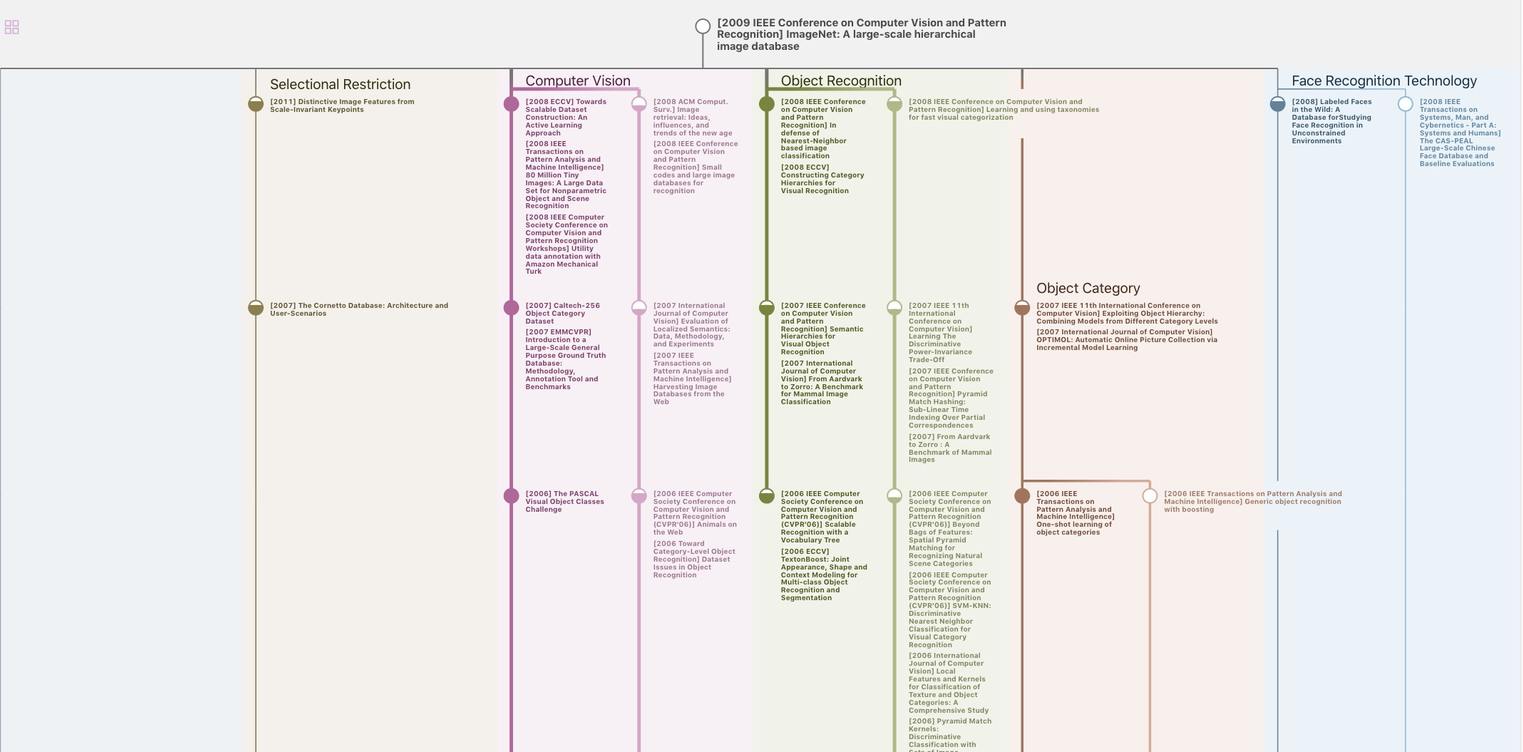
生成溯源树,研究论文发展脉络
Chat Paper
正在生成论文摘要