A Multivariate KPIs Anomaly Detection Framework With Dynamic Balancing Loss Training
IEEE Transactions on Network and Service Management(2023)
摘要
Anomaly detection on multivariate KPIs (Key Performance Indicators, such as CPU utilization, sockets status, and HTTP requests per second) is of utmost importance to the systems’ reliability. Unsupervised methods have been of considerable interests and have significantly progressed due to their superior effectiveness. However, the state-of-art unsupervised anomaly detection methods still suffer from high false or missed alarm rates. To this end, in this paper, we propose MM, a practical
M
ultivariate KPIs anomaly detection framework following the principles of
M
ulti-task learning with the proposed dynamic balancing loss function. To capture KPIs’ characteristics to the most extent, we simultaneously train multiple sequential autoencoders with different connections based on a designed semi-Random Connection Recurrent Neural Network (sRC-RNN). These autoencoders can be treated as different reconstruction tasks while training. Furthermore, we propose a dynamic loss function to adaptively balance the tasks’ weights. Extensive experiments show that MM outperforms the state-of-art unsupervised multivariate KPIs anomaly detection algorithms and achieves an average F1-score of 0.95 on two public machine-level KPIs datasets and 0.96 on an internal container-level KPIs dataset.
更多查看译文
关键词
anomaly detection
AI 理解论文
溯源树
样例
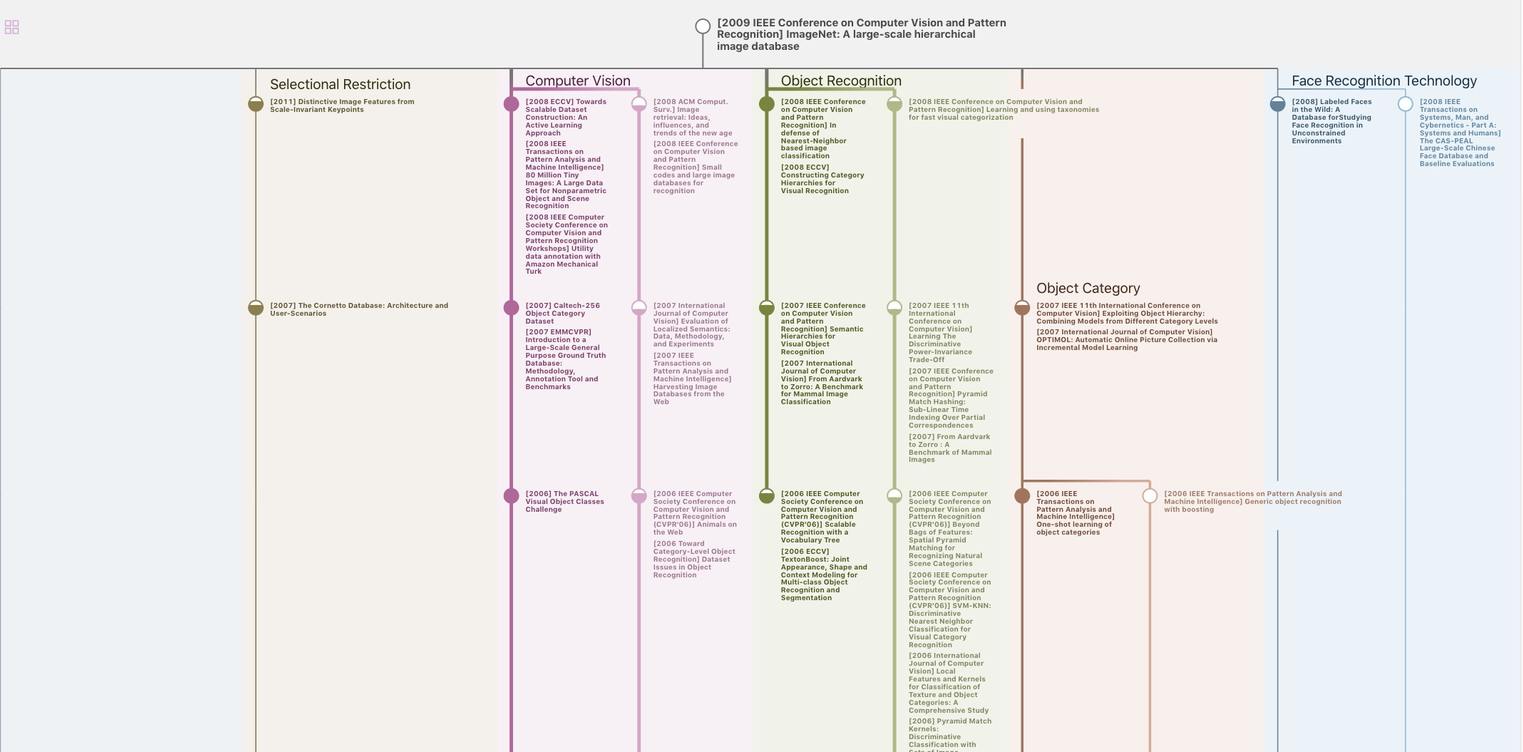
生成溯源树,研究论文发展脉络
Chat Paper
正在生成论文摘要