OSP: Boosting Distributed Model Training with 2-stage Synchronization
PROCEEDINGS OF THE 52ND INTERNATIONAL CONFERENCE ON PARALLEL PROCESSING, ICPP 2023(2023)
Abstract
Distributed deep learning (DDL) is a promising research area, which aims to increase the efficiency of training deep learning tasks with large size of datasets and models. As the computation capability of DDL nodes continues to increase, the network connection between nodes is becoming a major bottleneck. Various methods of gradient compression and improved model synchronization have been proposed to address this bottleneck in Parameter-Server-based DDL. However, these two types of methods can result in accuracy loss due to discarded gradients and have limited enhancement on the throughput of model synchronization, respectively. To address these challenges, we propose a new model synchronization method named Overlapped Synchronization Parallel (OSP), which achieves efficient communication with a 2-stage synchronization approach and uses Local-Gradient-based Parameter correction (LGP) to avoid accuracy loss caused by stale parameters. The prototype of OSP has been implemented using PyTorch and evaluated on commonly used deep learning models and datasets with a 9-node testbed. Evaluation results show that OSP can achieve up to 50% improvement in throughput without accuracy loss compared to popular synchronization models.
MoreTranslated text
Key words
Deep learning,distributed training,data parallel
AI Read Science
Must-Reading Tree
Example
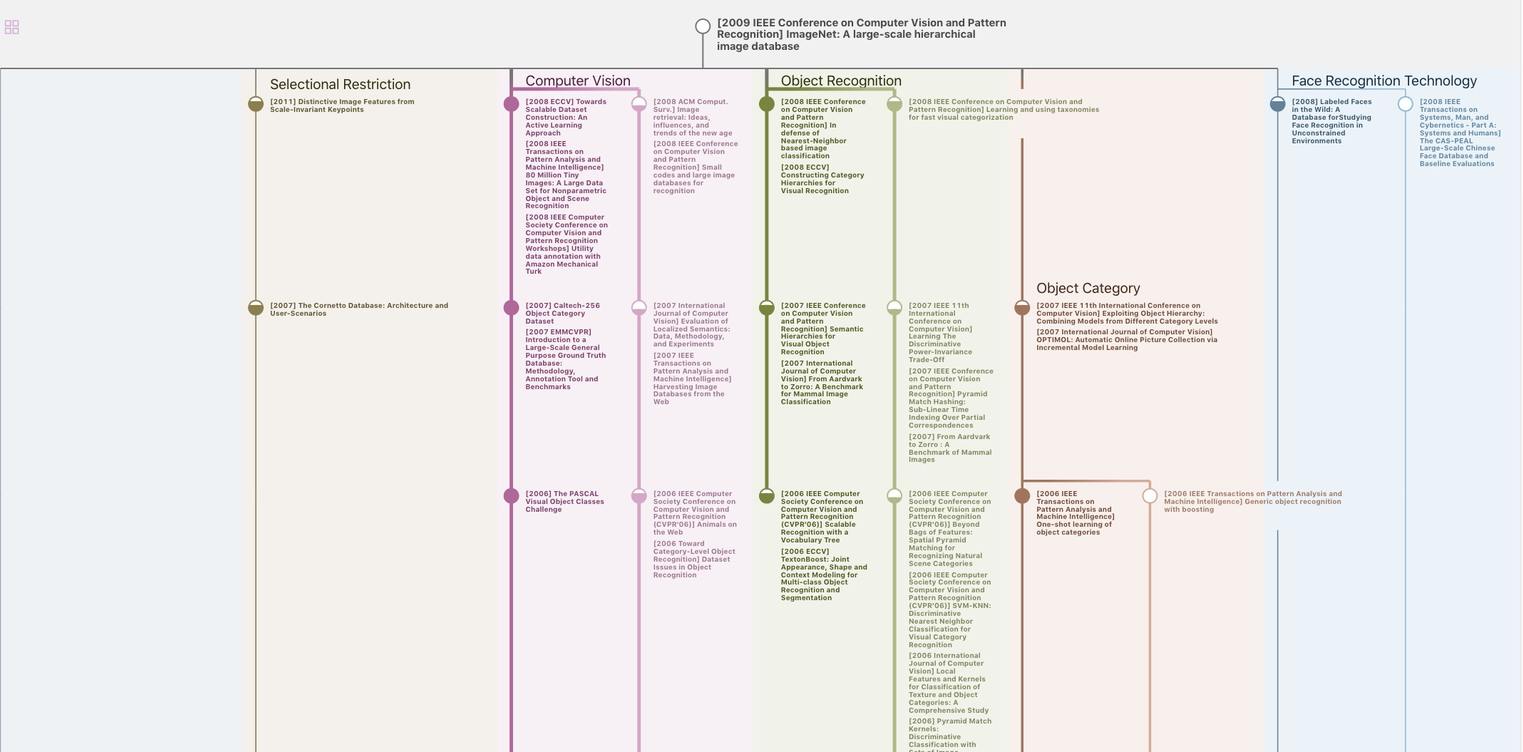
Generate MRT to find the research sequence of this paper
Chat Paper
Summary is being generated by the instructions you defined