Semi-supervised learning of order parameter in 2D Ising and XY models using Conditional Variational Autoencoders
arXiv (Cornell University)(2023)
摘要
We investigate the application of deep learning techniques employing the conditional variational autoencoders for semi-supervised learning of latent parameters to describe phase transition in the two-dimensional (2D) ferromagnetic Ising model and the two-dimensional XY model. For both models, we utilize spin configurations generated using the Wolff algorithms below and above the critical temperatures. For the 2D Ising model we find the latent parameter of conditional variational autoencoders is correlated to the known order parameter of magnetization more efficiently than their correspondence in variational autoencoders used previously. It can also clearly identify the restoration of the $\mathbb{Z}_2$ symmetry beyond the critical point. The critical temperature extracted from the latent parameter at larger lattices are found to be approaching its correct value. Similarly, for the 2D XY model, we find our chosen network with the latent representation of conditional variational autoencoders is equally capable of separating the two phases between the high and low temperatures, again at the correct critical temperature with reasonable accuracy. Together these results show that the latent representation of conditional variational autoencoders can be employed efficiently to identify the phases of condensed matter systems, without their prior knowledge.
更多查看译文
关键词
conditional variational autoencoders,2d ising,xy models
AI 理解论文
溯源树
样例
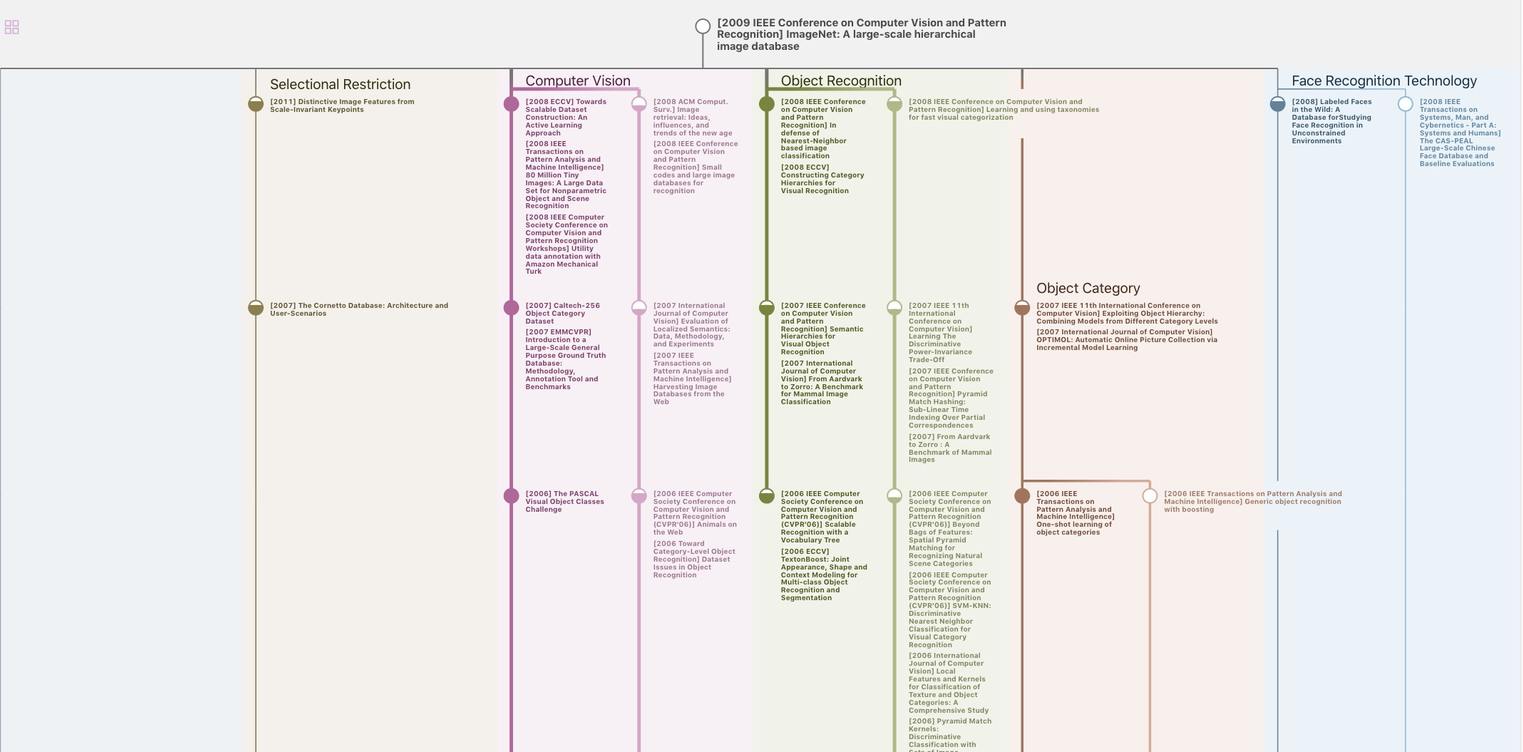
生成溯源树,研究论文发展脉络
Chat Paper
正在生成论文摘要