TrojanNet: Detecting Trojans in Quantum Circuits using Machine Learning
CoRR(2023)
摘要
Quantum computing holds tremendous potential for various applications, but its security remains a crucial concern. Quantum circuits need high-quality compilers to optimize the depth and gate count to boost the success probability on current noisy quantum computers. There is a rise of efficient but unreliable/untrusted compilers; however, they present a risk of tampering such as Trojan insertion. We propose TrojanNet, a novel approach to enhance the security of quantum circuits by detecting and classifying Trojan-inserted circuits. In particular, we focus on the Quantum Approximate Optimization Algorithm (QAOA) circuit that is popular in solving a wide range of optimization problems. We investigate the impact of Trojan insertion on QAOA circuits and develop a Convolutional Neural Network (CNN) model, referred to as TrojanNet, to identify their presence accurately. Using the Qiskit framework, we generate 12 diverse datasets by introducing variations in Trojan gate types, the number of gates, insertion locations, and compiler backends. These datasets consist of both original Trojan-free QAOA circuits and their corresponding Trojan-inserted counterparts. The generated datasets are then utilized for training and evaluating the TrojanNet model. Experimental results showcase an average accuracy of 98.80% and an average F1-score of 98.53% in effectively detecting and classifying Trojan-inserted QAOA circuits. Finally, we conduct a performance comparison between TrojanNet and existing machine learning-based Trojan detection methods specifically designed for conventional netlists.
更多查看译文
关键词
quantum circuits,trojans,machine learning
AI 理解论文
溯源树
样例
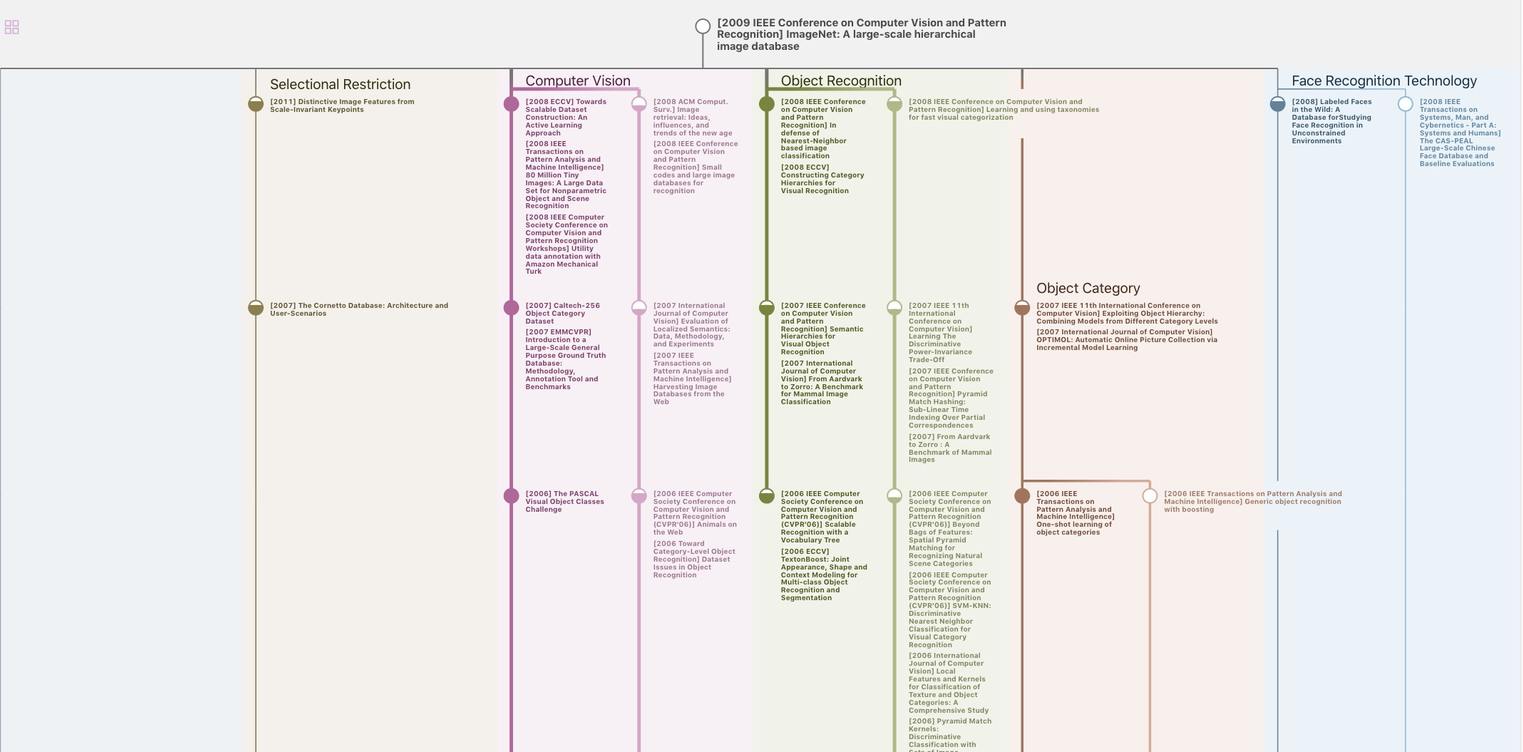
生成溯源树,研究论文发展脉络
Chat Paper
正在生成论文摘要