Incremental Learning on Food Instance Segmentation
CoRR(2023)
摘要
Food instance segmentation is essential to estimate the serving size of dishes in a food image. The recent cutting-edge techniques for instance segmentation are deep learning networks with impressive segmentation quality and fast computation. Nonetheless, they are hungry for data and expensive for annotation. This paper proposes an incremental learning framework to optimize the model performance given a limited data labelling budget. The power of the framework is a novel difficulty assessment model, which forecasts how challenging an unlabelled sample is to the latest trained instance segmentation model. The data collection procedure is divided into several stages, each in which a new sample package is collected. The framework allocates the labelling budget to the most difficult samples. The unlabelled samples that meet a certain qualification from the assessment model are used to generate pseudo-labels. Eventually, the manual labels and pseudo-labels are sent to the training data to improve the instance segmentation model. On four large-scale food datasets, our proposed framework outperforms current incremental learning benchmarks and achieves competitive performance with the model trained on fully annotated samples.
更多查看译文
关键词
segmentation,instance,food,learning
AI 理解论文
溯源树
样例
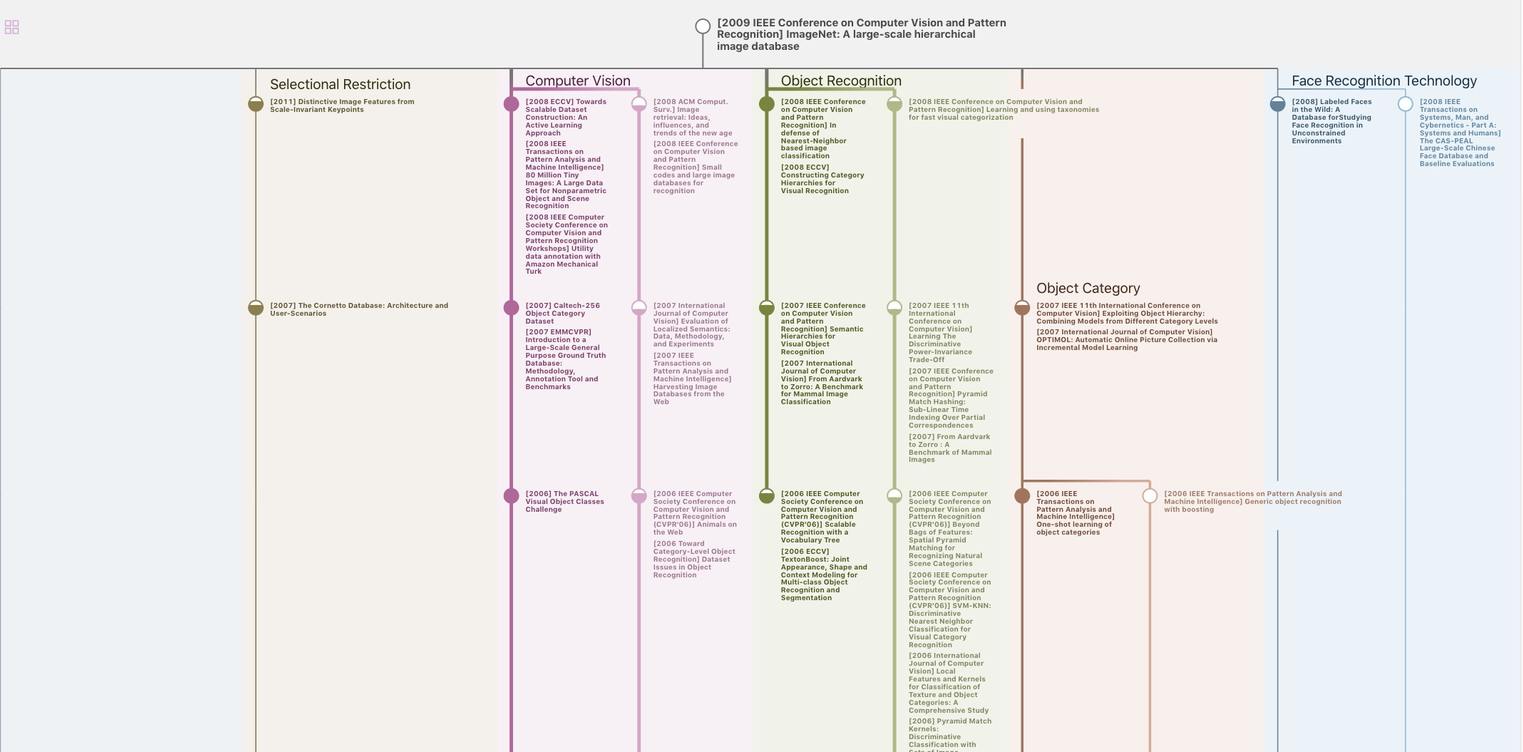
生成溯源树,研究论文发展脉络
Chat Paper
正在生成论文摘要