Scaling Laws for Discriminative Speech Recognition Rescoring Models
arxiv(2023)
摘要
Recent studies have found that model performance has a smooth power-law relationship, or scaling laws, with training data and model size, for a wide range of problems. These scaling laws allow one to choose nearly optimal data and model sizes. We study whether this scaling property is also applicable to second-pass rescoring, which is an important component of speech recognition systems. We focus on RescoreBERT as the rescoring model, which uses a pre-trained Transformer-based architecture fined tuned with an ASR discriminative loss. Using such a rescoring model, we show that the word error rate (WER) follows a scaling law for over two orders of magnitude as training data and model size increase. In addition, it is found that a pre-trained model would require less data than a randomly initialized model of the same size, representing effective data transferred from pre-training step. This effective data transferred is found to also follow a scaling law with the data and model size.
更多查看译文
关键词
scaling,recognition,models,speech
AI 理解论文
溯源树
样例
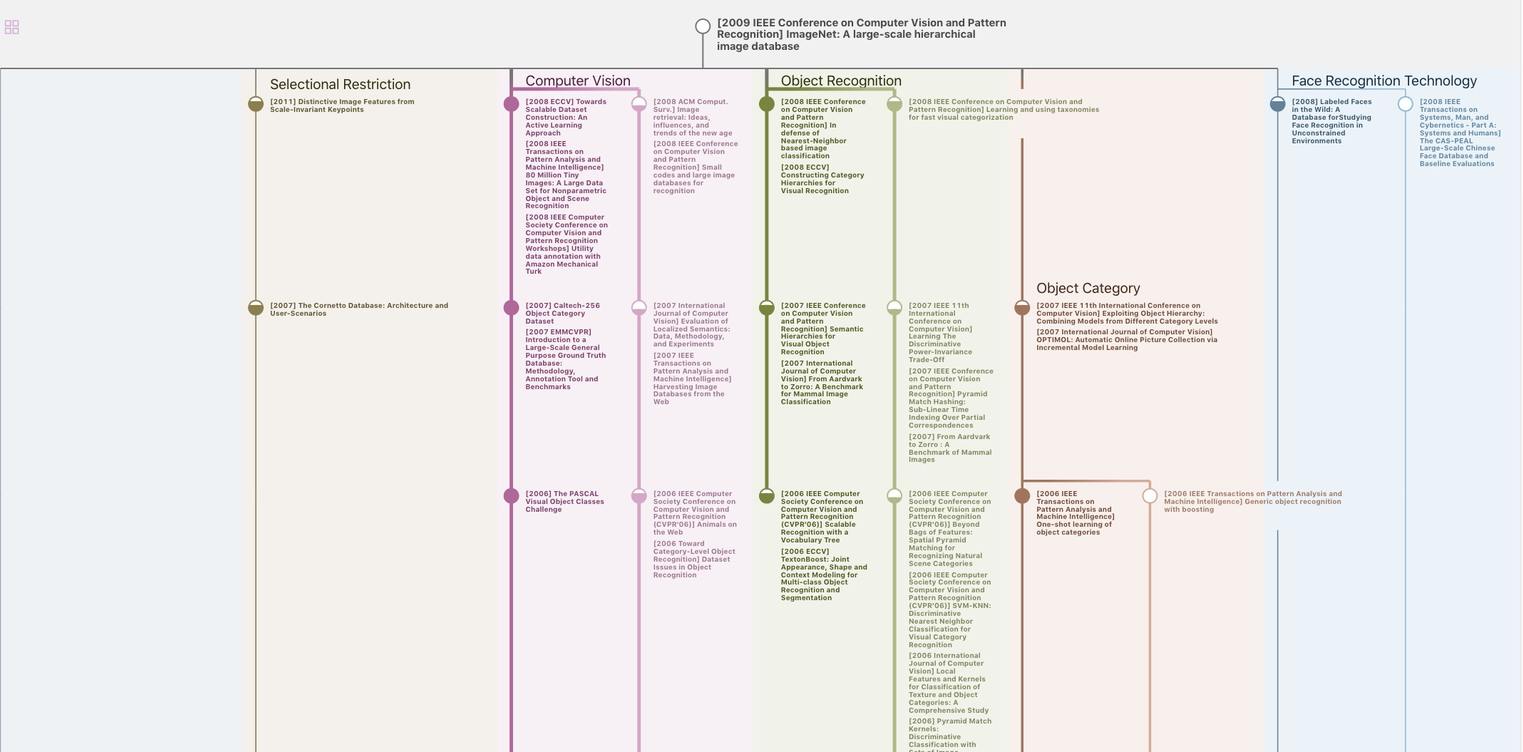
生成溯源树,研究论文发展脉络
Chat Paper
正在生成论文摘要