Classification of Infant Sleep/Wake States: Cross-Attention among Large Scale Pretrained Transformer Networks using Audio, ECG, and IMU Data
CoRR(2023)
摘要
Infant sleep is critical to brain and behavioral development. Prior studies on infant sleep/wake classification have been largely limited to reliance on expensive and burdensome polysomnography (PSG) tests in the laboratory or wearable devices that collect single-modality data. To facilitate data collection and accuracy of detection, we aimed to advance this field of study by using a multi-modal wearable device, LittleBeats (LB), to collect audio, electrocardiogram (ECG), and inertial measurement unit (IMU) data among a cohort of 28 infants. We employed a 3-branch (audio/ECG/IMU) large scale transformer-based neural network (NN) to demonstrate the potential of such multi-modal data. We pretrained each branch independently with its respective modality, then finetuned the model by fusing the transformer layers with cross-attention. We show that multimodal data significantly improves sleep/wake classification (accuracy = 0.880), compared with use of a single modality (accuracy = 0.732). Our approach to multi-modal mid-level fusion may be adaptable to a diverse range of architectures and tasks, expanding future directions of infant behavioral research.
更多查看译文
关键词
infant sleep/wake,scale pretrained transformer networks,sleep/wake states,cross-attention
AI 理解论文
溯源树
样例
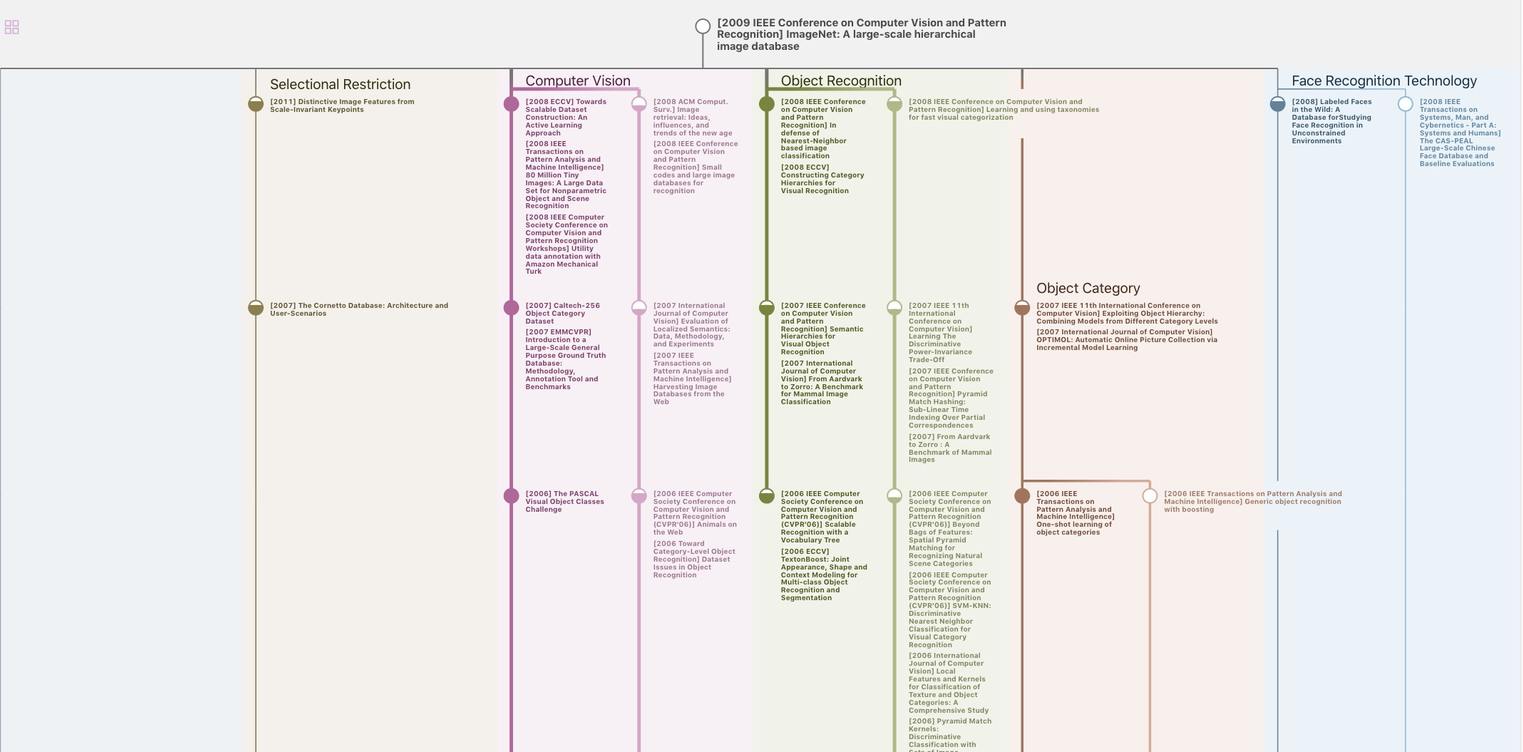
生成溯源树,研究论文发展脉络
Chat Paper
正在生成论文摘要