Value-aware Importance Weighting for Off-policy Reinforcement Learning
CoRR(2023)
摘要
Importance sampling is a central idea underlying off-policy prediction in reinforcement learning. It provides a strategy for re-weighting samples from a distribution to obtain unbiased estimates under another distribution. However, importance sampling weights tend to exhibit extreme variance, often leading to stability issues in practice. In this work, we consider a broader class of importance weights to correct samples in off-policy learning. We propose the use of $\textit{value-aware importance weights}$ which take into account the sample space to provide lower variance, but still unbiased, estimates under a target distribution. We derive how such weights can be computed, and detail key properties of the resulting importance weights. We then extend several reinforcement learning prediction algorithms to the off-policy setting with these weights, and evaluate them empirically.
更多查看译文
关键词
reinforcement learning,importance,value-aware,off-policy
AI 理解论文
溯源树
样例
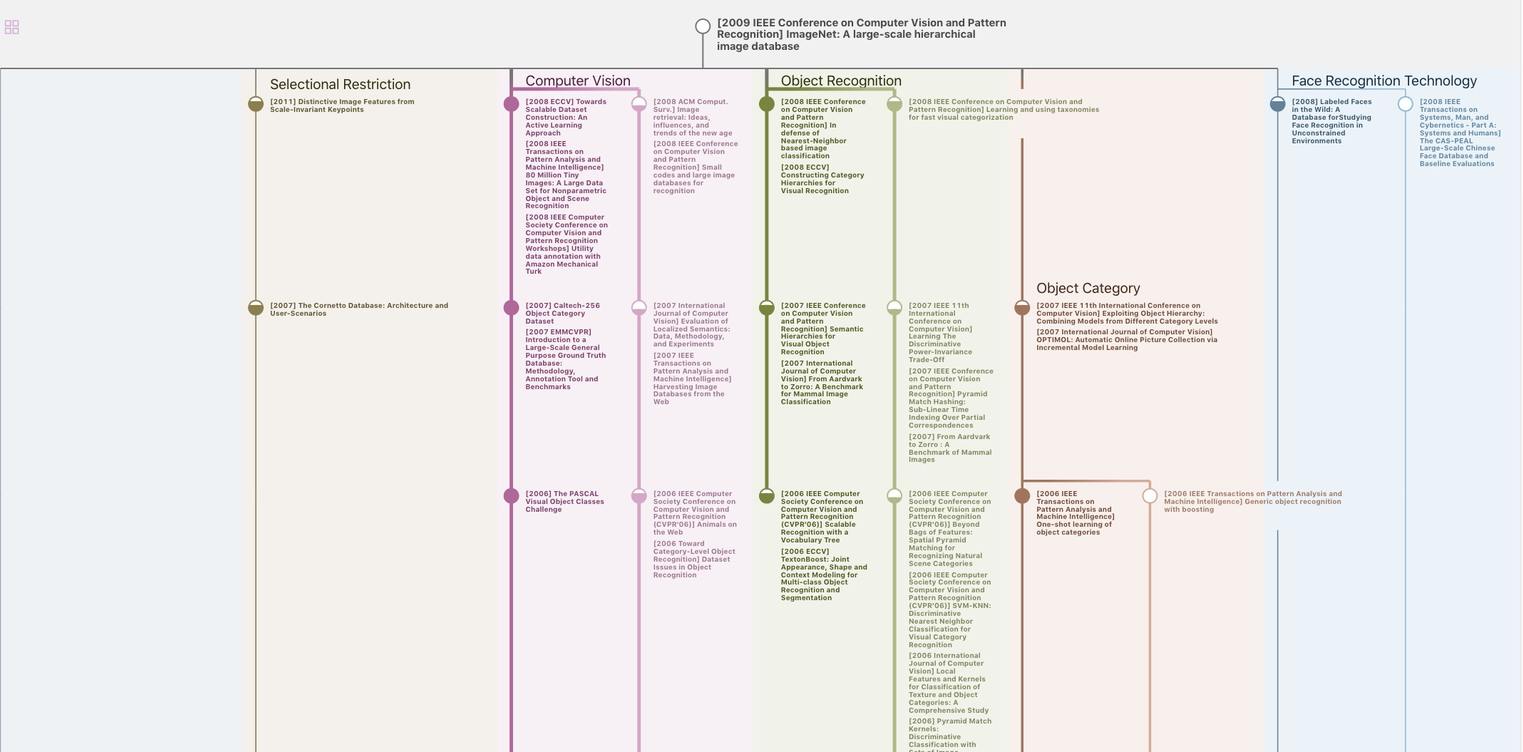
生成溯源树,研究论文发展脉络
Chat Paper
正在生成论文摘要