Large-Scale Unsupervised Audio Pre-Training for Video-to-Speech Synthesis
IEEE-ACM TRANSACTIONS ON AUDIO SPEECH AND LANGUAGE PROCESSING(2024)
摘要
Video-to-speech synthesis is the task of reconstructing the speech signal from a silent video of a speaker. Previous approaches train on data from almost exclusively audio-visual datasets, i.e., every audio sample has a corresponding video sample. This precludes the use of abundant audio-only datasets which may not have a corresponding visual modality such as audiobooks, radio podcasts, and speech recognition datasets. In this paper we propose to train encoder-decoder models on more than 3,500 hours of audio data at 24 kHz, and then use the pre-trained decoders to initialize the audio decoders for the video-to-speech synthesis task. The pre-training step uses audio samples only and does not require labels or corresponding samples from other modalities (visual, text). We demonstrate that this improves the reconstructed speech and that it is an unexplored way to improve the quality of the generator in a cross-modal task while only requiring samples from one of the modalities. We conduct experiments using both raw audio and mel spectrograms as target outputs and benchmark our models with existing work.
更多查看译文
关键词
Video-to-speech,speech synthesis,generative adversarial networks (GANs),conformer,pre-training
AI 理解论文
溯源树
样例
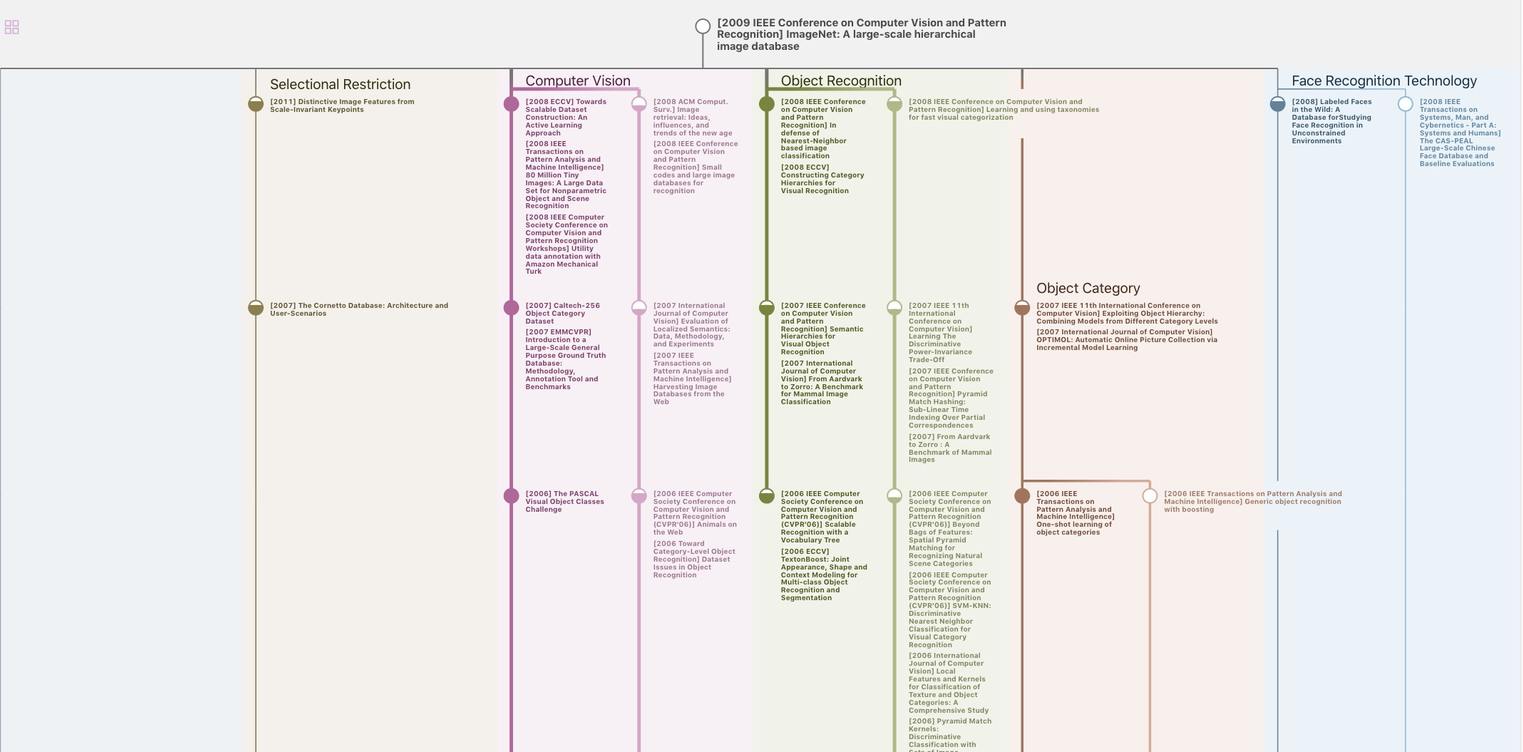
生成溯源树,研究论文发展脉络
Chat Paper
正在生成论文摘要