Robust Proxy: Improving Adversarial Robustness by Robust Proxy Learning
IEEE Trans. Inf. Forensics Secur.(2023)
Abstract
Recently, it has been widely known that deep neural networks are highly vulnerable and easily broken by adversarial attacks. To mitigate the adversarial vulnerability, many defense algorithms have been proposed. Recently, to improve adversarial robustness, many works try to enhance feature representation by imposing more direct supervision on the discriminative feature. However, existing approaches lack an understanding of learning adversarially robust feature representation. In this paper, we propose a novel training framework called Robust Proxy Learning. In the proposed method, the model explicitly learns robust feature representations with robust proxies. To this end, firstly, we demonstrate that we can generate class-representative robust features by adding class-wise robust perturbations. Then, we use the class representative features as robust proxies. With the class-wise robust features, the model explicitly learns adversarially robust features through the proposed robust proxy learning framework. Through extensive experiments, we verify that we can manually generate robust features, and our proposed learning framework could increase the robustness of the DNNs.
MoreTranslated text
Key words
Robust perturbation,class-wise robust perturbation,robust proxy learning
AI Read Science
Must-Reading Tree
Example
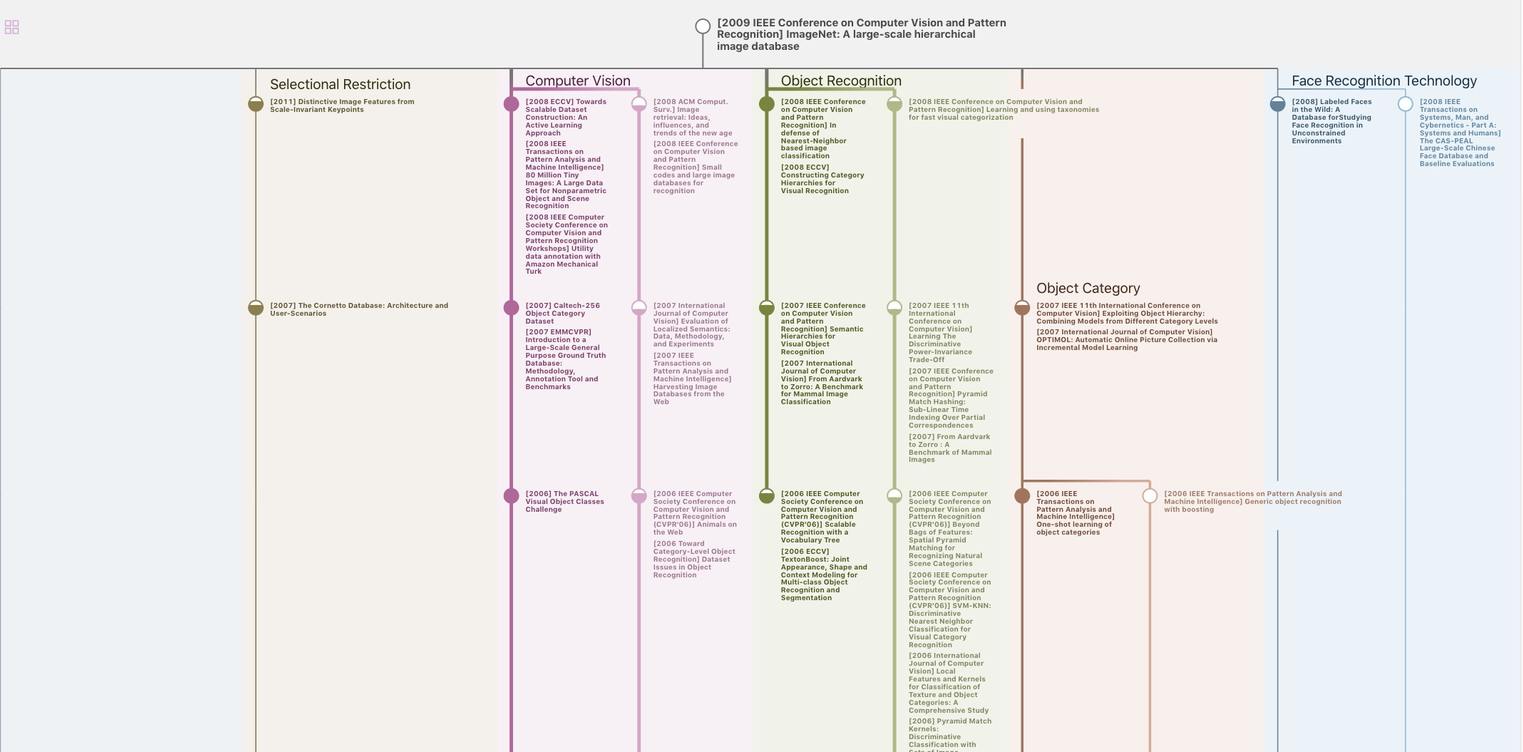
Generate MRT to find the research sequence of this paper
Chat Paper
Summary is being generated by the instructions you defined