Towards predicting Pedestrian Evacuation Time and Density from Floorplans using a Vision Transformer
CoRR(2023)
摘要
Conventional pedestrian simulators are inevitable tools in the design process of a building, as they enable project engineers to prevent overcrowding situations and plan escape routes for evacuation. However, simulation runtime and the multiple cumbersome steps in generating simulation results are potential bottlenecks during the building design process. Data-driven approaches have demonstrated their capability to outperform conventional methods in speed while delivering similar or even better results across many disciplines. In this work, we present a deep learning-based approach based on a Vision Transformer to predict density heatmaps over time and total evacuation time from a given floorplan. Specifically, due to limited availability of public datasets, we implement a parametric data generation pipeline including a conventional simulator. This enables us to build a large synthetic dataset that we use to train our architecture. Furthermore, we seamlessly integrate our model into a BIM-authoring tool to generate simulation results instantly and automatically.
更多查看译文
关键词
pedestrian evacuation time,floorplans,vision
AI 理解论文
溯源树
样例
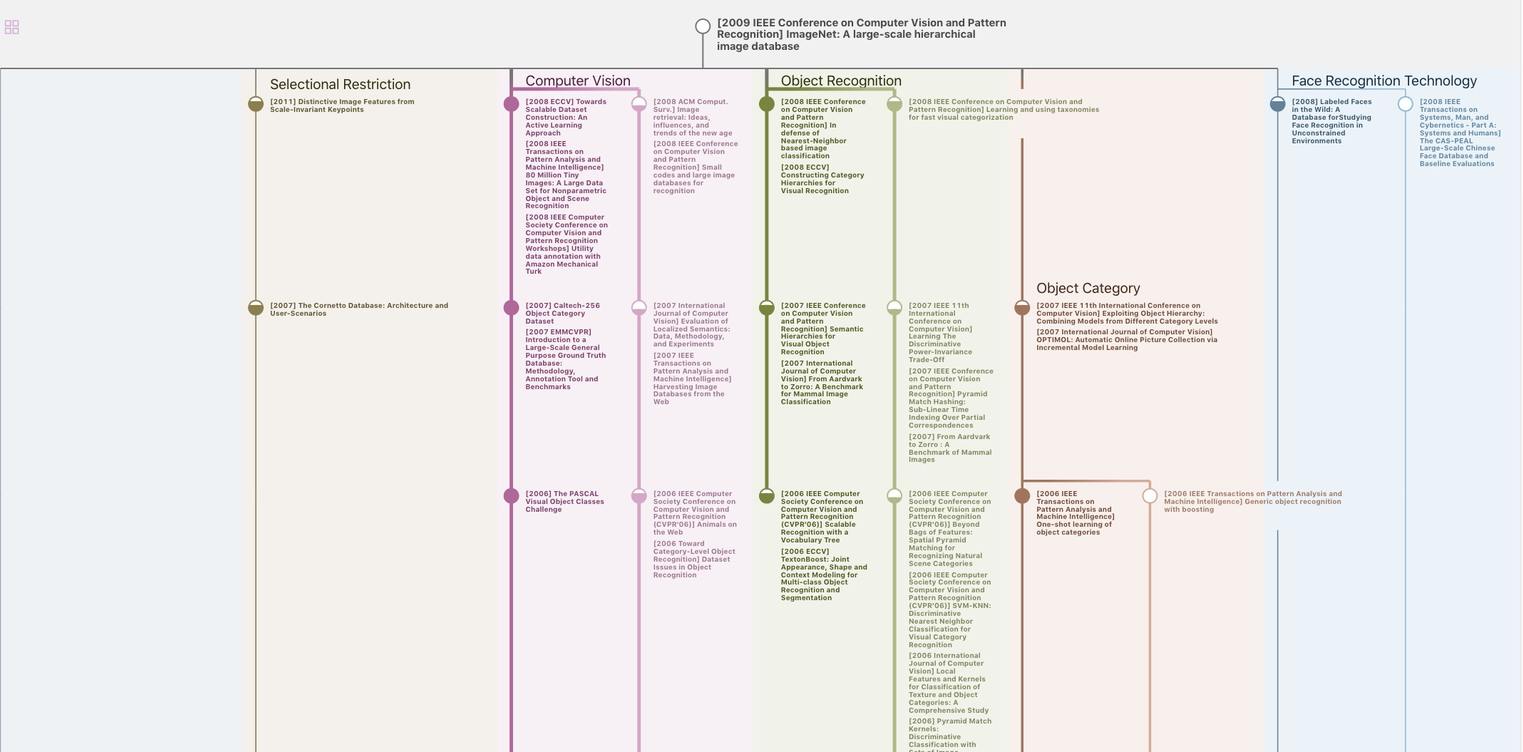
生成溯源树,研究论文发展脉络
Chat Paper
正在生成论文摘要