ADD-UNet: An Adjacent Dual-Decoder UNet for SAR-to-Optical Translation.
Remote Sensing(2023)
Abstract
Synthetic aperture radar (SAR) imagery has the advantages of all-day and all-weather observation. However, due to the imaging mechanism of microwaves, it is difficult for nonexperts to interpret SAR images. Transferring SAR imagery into optical imagery can better improve the interpretation of SAR data and support the further fusion research of multi-source remote sensing. Methods based on generative adversarial networks (GAN) have been proven to be effective in SAR-to-optical translation tasks. To further improve the translation results of SAR data, we propose a method of an adjacent dual-decoder UNet (ADD-UNet) based on conditional GAN (cGAN) for SAR-to-optical translation. The proposed network architecture adds an adjacent scale of the decoder to the UNet, and the multi-scale feature aggregation of the two decoders improves structures, details, and edge sharpness of generated images while introducing fewer parameters compared with UNet++. In addition, we combine multi-scale structure similarity (MS-SSIM) loss and L1 loss as loss functions with cGAN loss together to help preserve structures and details. The experimental results demonstrate the superiority of our method compared with several state-of-the-art methods.
MoreTranslated text
Key words
SAR-to-optical translation,conditional generative adversarial networks (cGAN),SAR,ADD-UNet,MS-SSIM
AI Read Science
Must-Reading Tree
Example
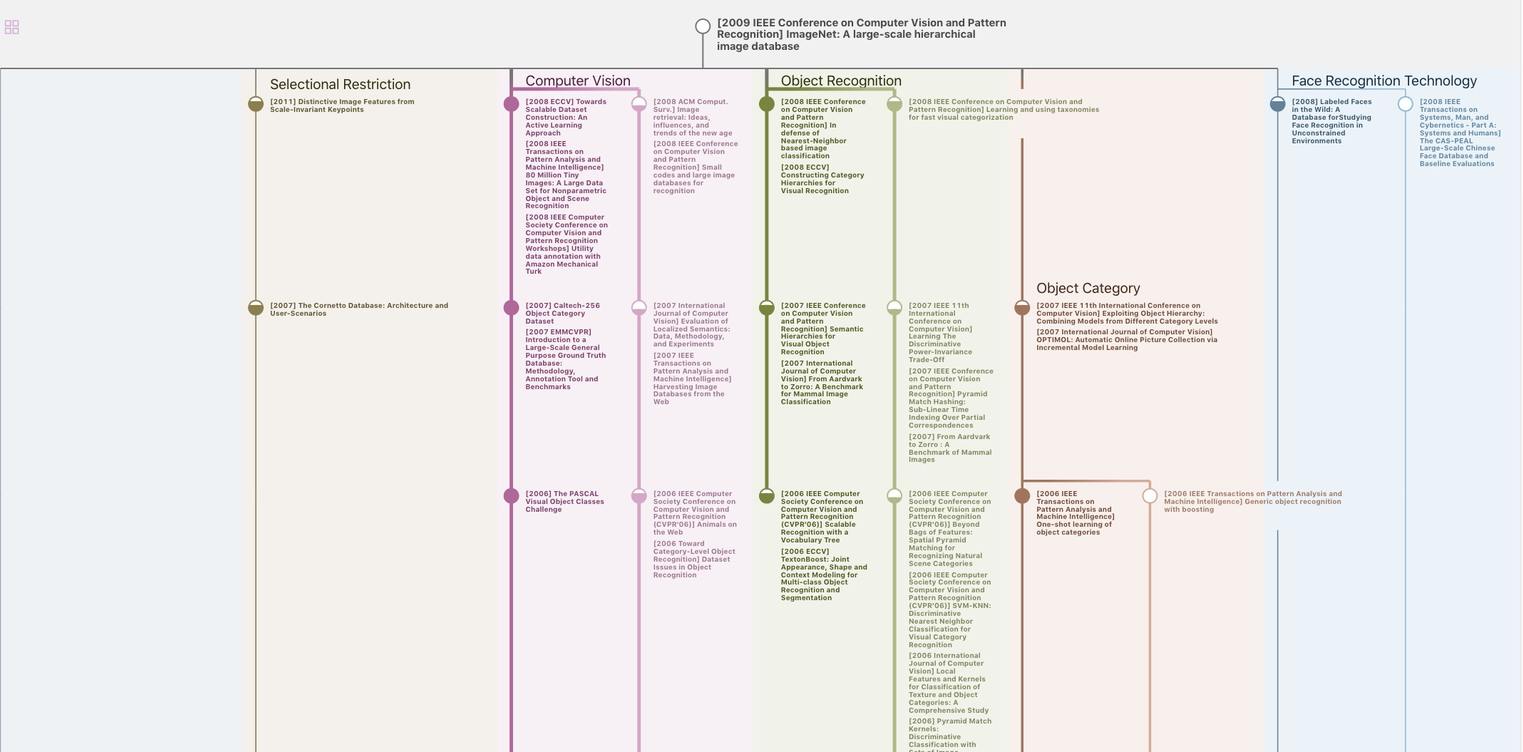
Generate MRT to find the research sequence of this paper
Chat Paper
Summary is being generated by the instructions you defined