Reciprocal Sequential Recommendation
PROCEEDINGS OF THE 17TH ACM CONFERENCE ON RECOMMENDER SYSTEMS, RECSYS 2023(2023)
摘要
Reciprocal recommender system (RRS), considering a two-way matching between two parties, has been widely applied in online platforms like online dating and recruitment. Existing RRS models mainly capture static user preferences, which have neglected the evolving user tastes and the dynamic matching relation between the two parties. Although dynamic user modeling has been well-studied in sequential recommender systems, existing solutions are developed in a user-oriented manner. Therefore, it is non-trivial to adapt sequential recommendation algorithms to reciprocal recommendation. In this paper, we formulate RRS as a distinctive sequence matching task, and further propose a new approach ReSeq for RRS, which is short for Reciprocal Sequential recommendation. To capture dual-perspective matching, we propose to learn fine-grained sequence similarities by co-attention mechanism across different time steps. Further, to improve the inference efficiency, we introduce the self-distillation technique to distill knowledge from the fine-grained matching module into the more efficient student module. In the deployment stage, only the efficient student module is used, greatly speeding up the similarity computation. Extensive experiments on five real-world datasets from two scenarios demonstrate the effectiveness and efficiency of the proposed method. Our code is available at https://github.com/RUCAIBox/ReSeq/.
更多查看译文
关键词
reciprocal recommendation,self-distillation,sequential recommendation
AI 理解论文
溯源树
样例
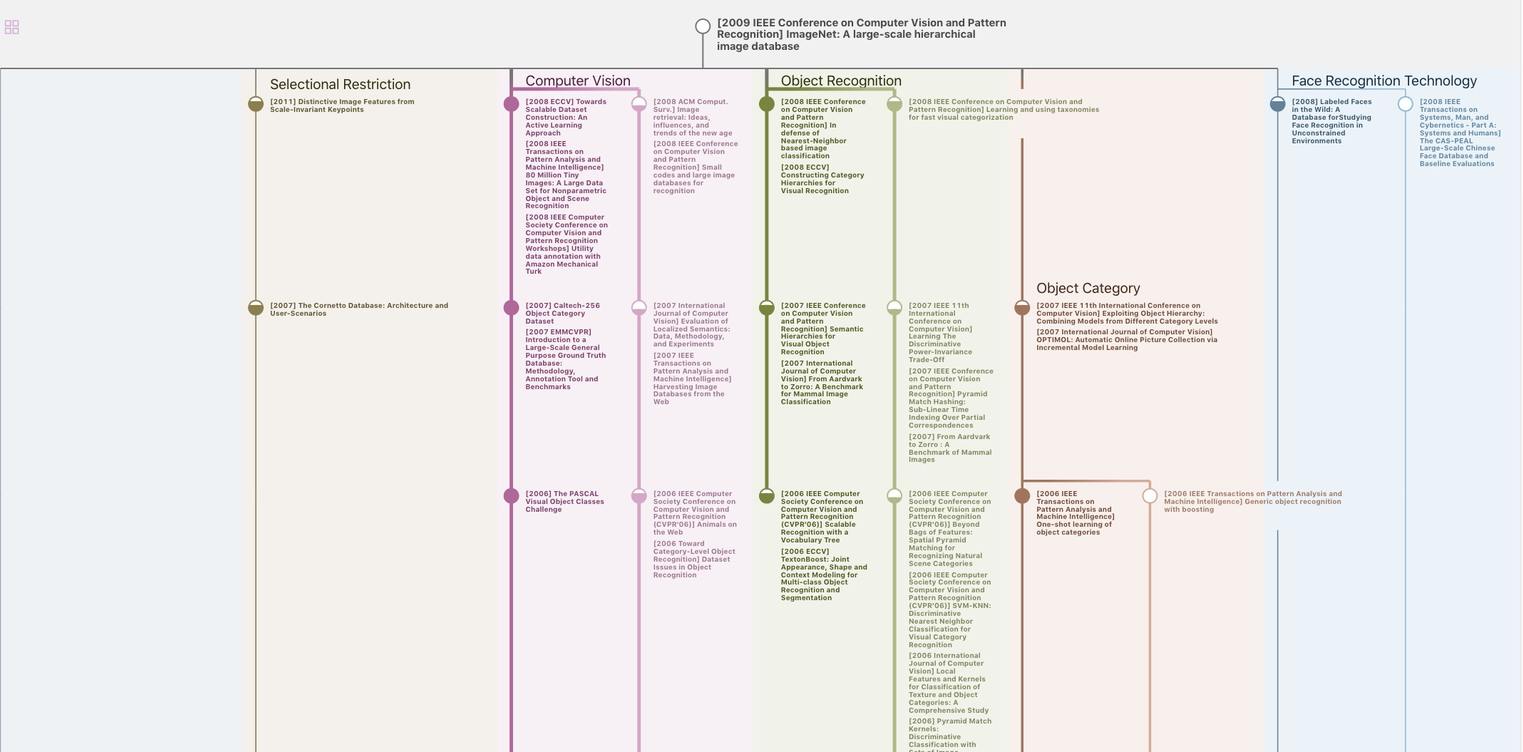
生成溯源树,研究论文发展脉络
Chat Paper
正在生成论文摘要