Sum-of-squares relaxations for polynomial min–max problems over simple sets
Mathematical Programming(2024)
摘要
We consider min–max optimization problems for polynomial functions, where a multivariate polynomial is maximized with respect to a subset of variables, and the resulting maximal value is minimized with respect to the remaining variables. When the variables belong to simple sets (e.g., a hypercube, the Euclidean hypersphere, or a ball), we derive a sum-of-squares formulation based on a primal-dual approach. In the simplest setting, we provide a convergence proof when the degree of the relaxation tends to infinity and observe empirically that it can be finitely convergent in several situations. Moreover, our formulation leads to an interesting link with feasibility certificates for polynomial inequalities based on Putinar’s Positivstellensatz.
更多查看译文
关键词
Polynomial optimization,Sum-of-squares,Min–max problems,Semidefinite programming,90C22,11E25
AI 理解论文
溯源树
样例
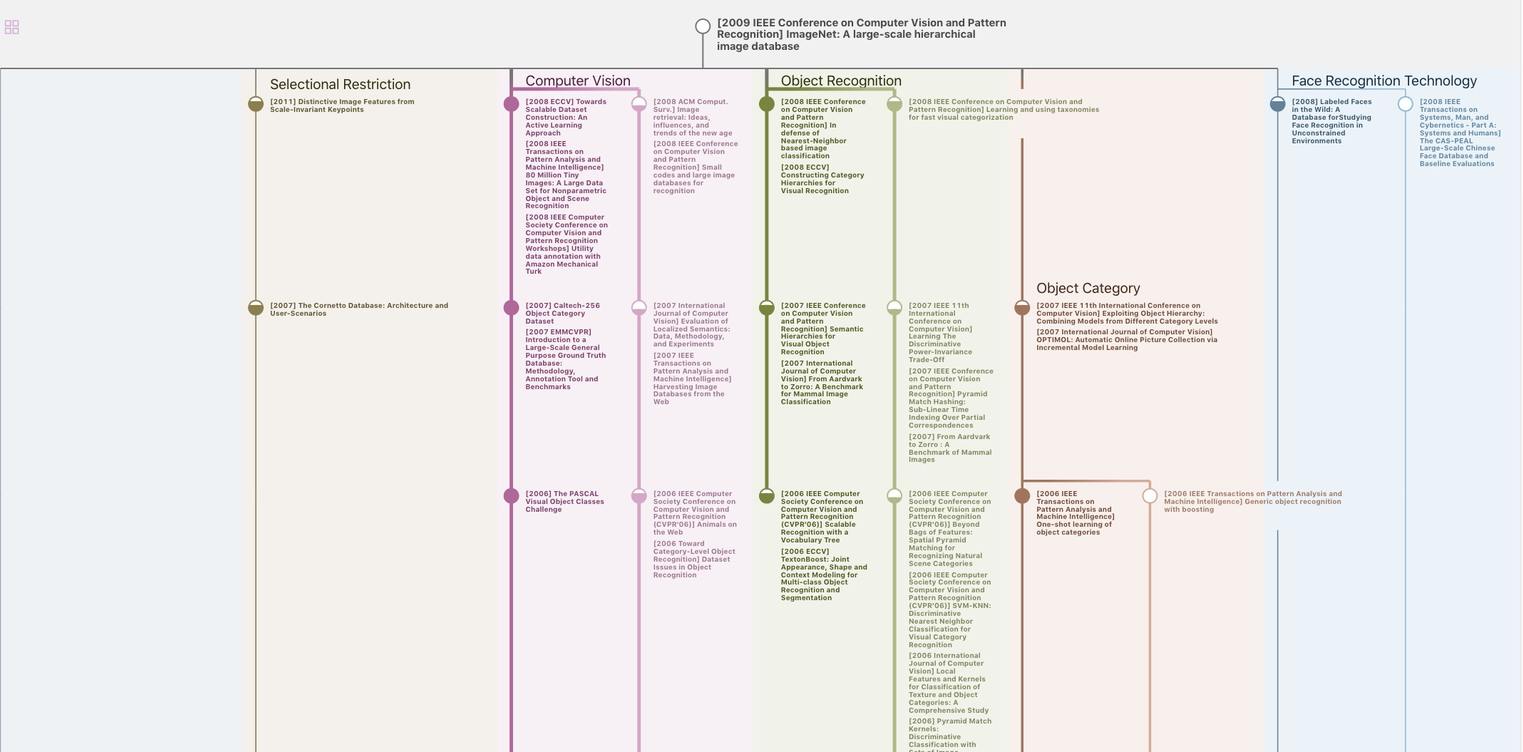
生成溯源树,研究论文发展脉络
Chat Paper
正在生成论文摘要