Histopathology Image Classification Using Deep Manifold Contrastive Learning
MEDICAL IMAGE COMPUTING AND COMPUTER ASSISTED INTERVENTION, MICCAI 2023, PT VI(2023)
摘要
Contrastive learning has gained popularity due to its robustness with good feature representation performance. However, cosine distance, the commonly used similarity metric in contrastive learning, is not well suited to represent the distance between two data points, especially on a nonlinear feature manifold. Inspired by manifold learning, we propose a novel extension of contrastive learning that leverages geodesic distance between features as a similarity metric for histopathology whole slide image classification. To reduce the computational overhead in manifold learning, we propose geodesic-distance-based feature clustering for efficient contrastive loss evaluation using prototypes without time-consuming pairwise feature similarity comparison. The efficacy of the proposed method is evaluated on two real-world histopathology image datasets. Results demonstrate that our method outperforms state-of-the-art cosine-distance-based contrastive learning methods.
更多查看译文
关键词
Contrastive learning,Manifold learning,Geodesic distance,Histopathology image classification,Multiple instance learning
AI 理解论文
溯源树
样例
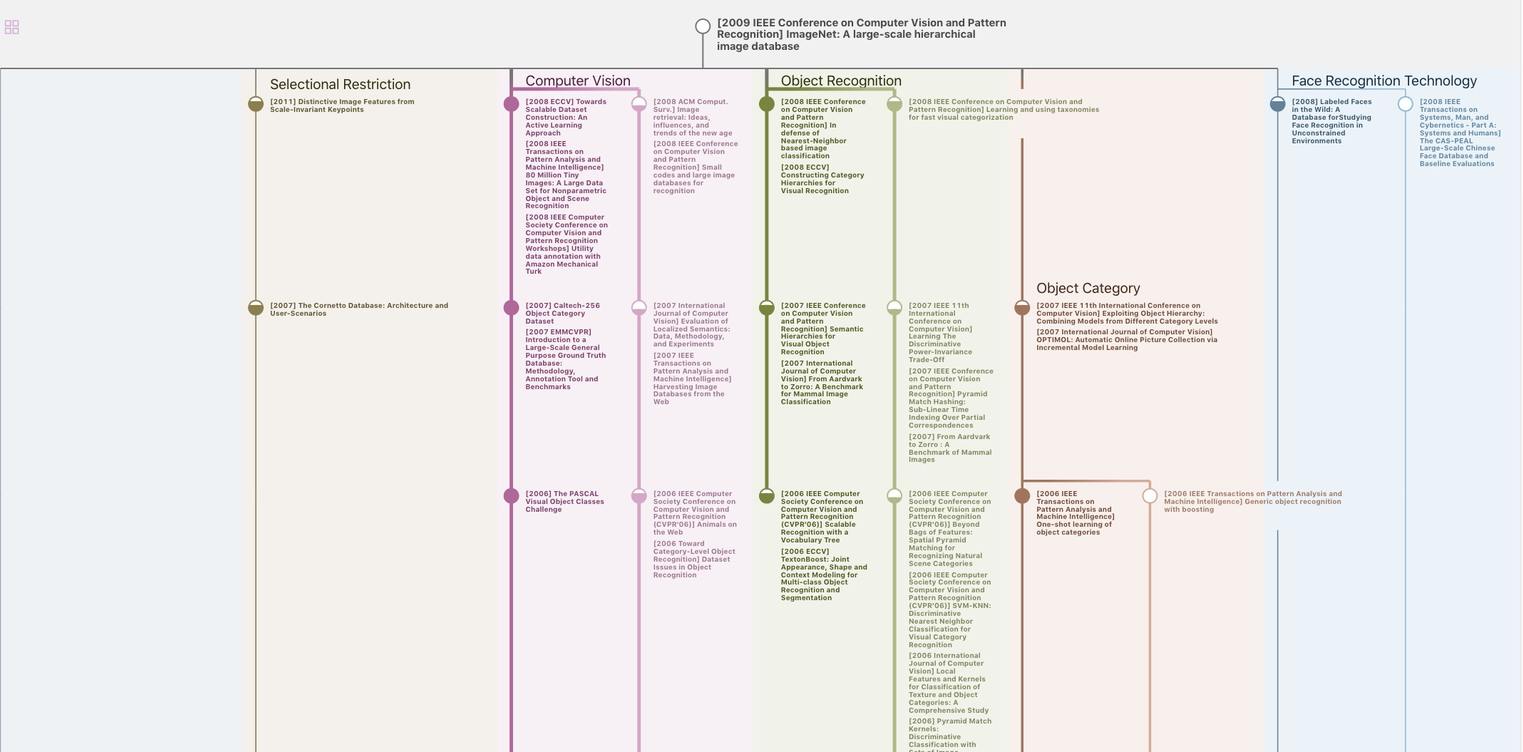
生成溯源树,研究论文发展脉络
Chat Paper
正在生成论文摘要