A differentiable Gaussian Prototype Layer for explainable Segmentation
CoRR(2023)
摘要
We introduce a Gaussian Prototype Layer for gradient-based prototype learning and demonstrate two novel network architectures for explainable segmentation one of which relies on region proposals. Both models are evaluated on agricultural datasets. While Gaussian Mixture Models (GMMs) have been used to model latent distributions of neural networks before, they are typically fitted using the EM algorithm. Instead, the proposed prototype layer relies on gradient-based optimization and hence allows for end-to-end training. This facilitates development and allows to use the full potential of a trainable deep feature extractor. We show that it can be used as a novel building block for explainable neural networks. We employ our Gaussian Prototype Layer in (1) a model where prototypes are detected in the latent grid and (2) a model inspired by Fast-RCNN with SLIC superpixels as region proposals. The earlier achieves a similar performance as compared to the state-of-the art while the latter has the benefit of a more precise prototype localization that comes at the cost of slightly lower accuracies. By introducing a gradient-based GMM layer we combine the benefits of end-to-end training with the simplicity and theoretical foundation of GMMs which will allow to adapt existing semi-supervised learning strategies for prototypical part models in future.
更多查看译文
关键词
explainable segmentation,differentiable gaussian prototype layer
AI 理解论文
溯源树
样例
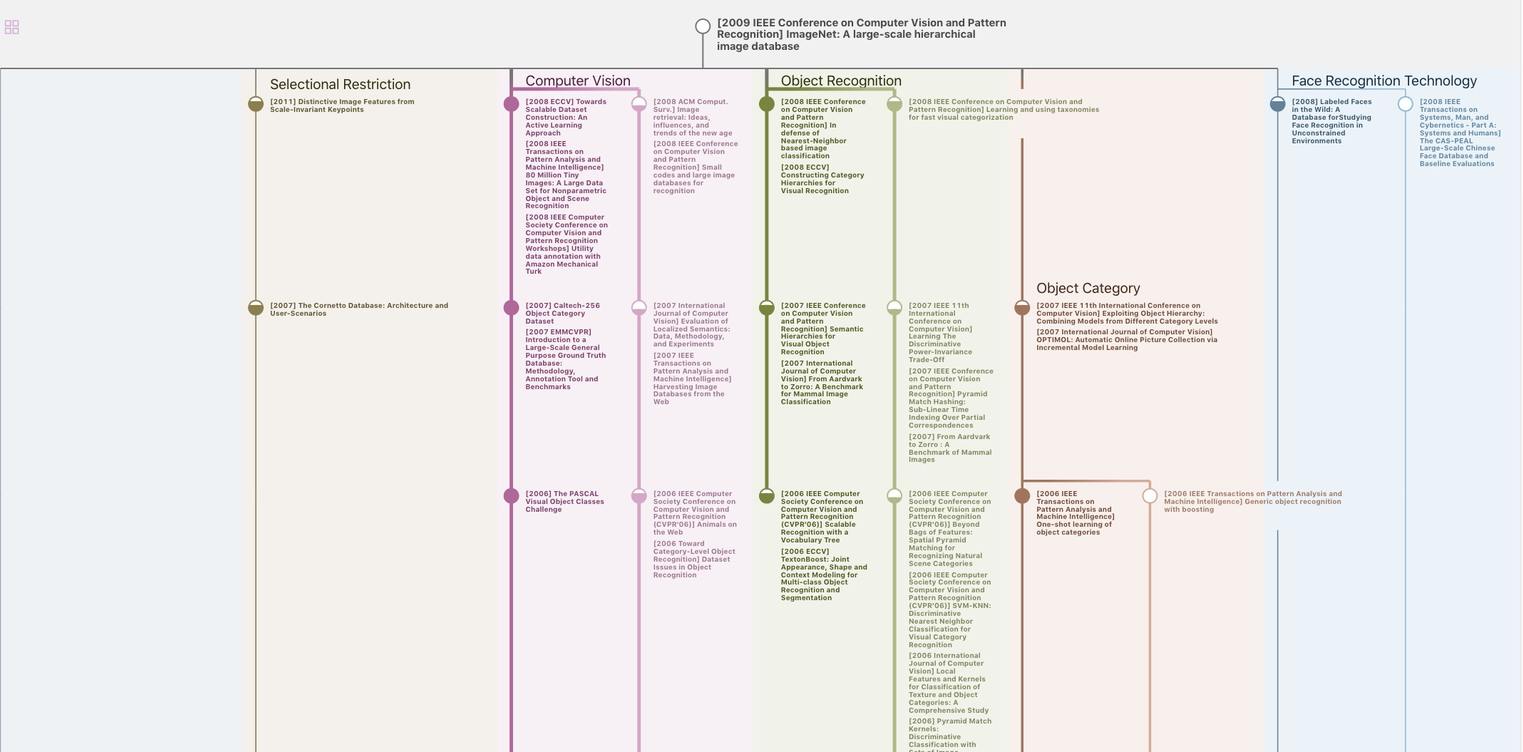
生成溯源树,研究论文发展脉络
Chat Paper
正在生成论文摘要