GPatcher: A Simple and Adaptive MLP Model for Alleviating Graph Heterophily
CoRR(2023)
摘要
While graph heterophily has been extensively studied in recent years, a fundamental research question largely remains nascent: How and to what extent will graph heterophily affect the prediction performance of graph neural networks (GNNs)? In this paper, we aim to demystify the impact of graph heterophily on GNN spectral filters. Our theoretical results show that it is essential to design adaptive polynomial filters that adapts different degrees of graph heterophily to guarantee the generalization performance of GNNs. Inspired by our theoretical findings, we propose a simple yet powerful GNN named GPatcher by leveraging the MLP-Mixer architectures. Our approach comprises two main components: (1) an adaptive patch extractor function that automatically transforms each node's non-Euclidean graph representations to Euclidean patch representations given different degrees of heterophily, and (2) an efficient patch mixer function that learns salient node representation from both the local context information and the global positional information. Through extensive experiments, the GPatcher model demonstrates outstanding performance on node classification compared with popular homophily GNNs and state-of-the-art heterophily GNNs.
更多查看译文
关键词
adaptive mlp model,graph
AI 理解论文
溯源树
样例
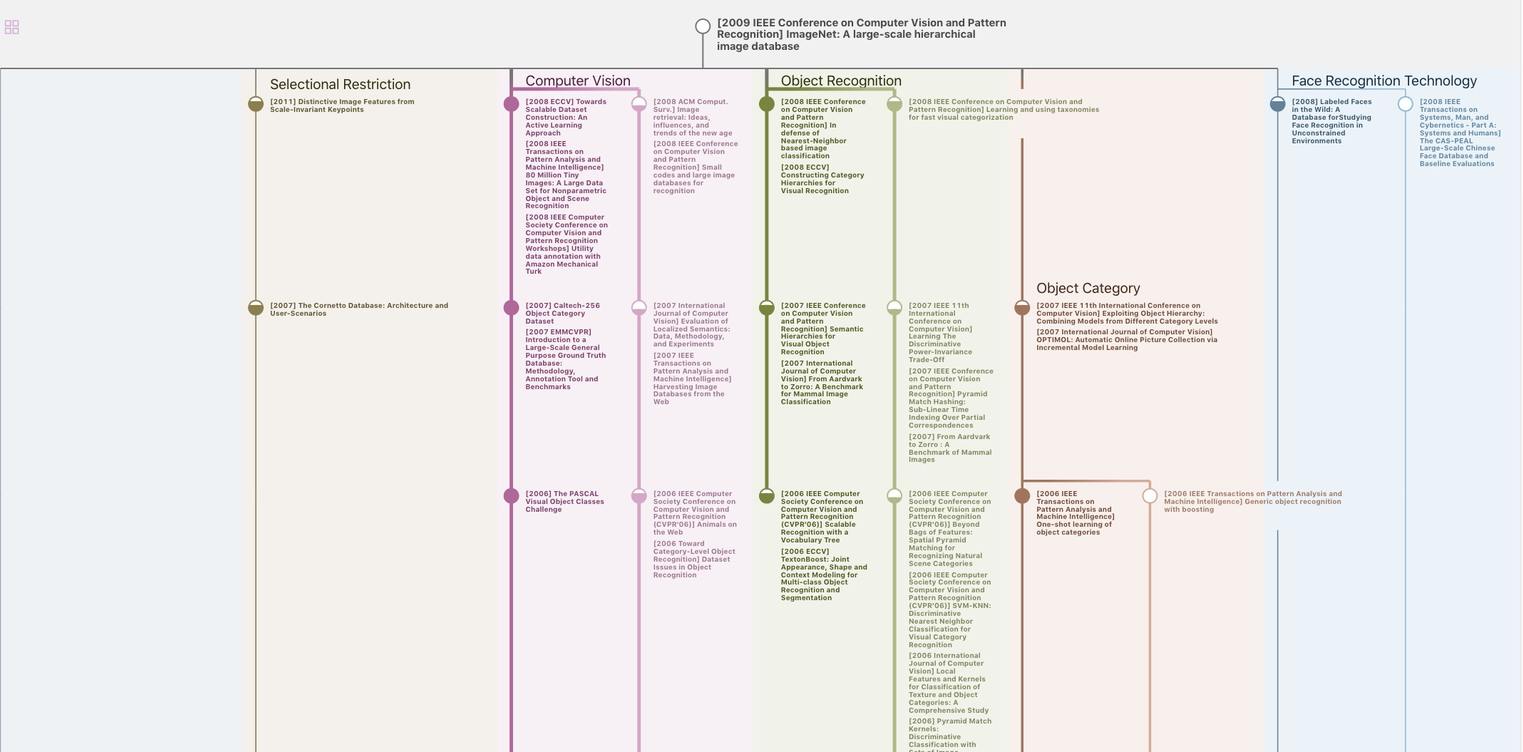
生成溯源树,研究论文发展脉络
Chat Paper
正在生成论文摘要