A na{\i}ve Bayesian graphical elastic net: driving advances in differential network analysis
arXiv (Cornell University)(2023)
摘要
Differential Networks (DNs), tools that encapsulate interactions within intricate systems, are brought under the Bayesian lens in this research. A novel na{\i}ve Bayesian adaptive graphical elastic net (BAE) prior is introduced to estimate the components of the DN. A heuristic structure determination mechanism and a block Gibbs sampler are derived. Performance is initially gauged on synthetic datasets encompassing various network topologies, aiming to assess and compare the flexibility to those of the Bayesian adaptive graphical lasso and ridge-type procedures. The na{\i}ve BAE estimator consistently ranks within the top two performers, highlighting its inherent adaptability. Finally, the BAE is applied to real-world datasets across diverse domains such as oncology, nephrology, and enology, underscoring its potential utility in comprehensive network analysis.
更多查看译文
关键词
network,differential,bayesian
AI 理解论文
溯源树
样例
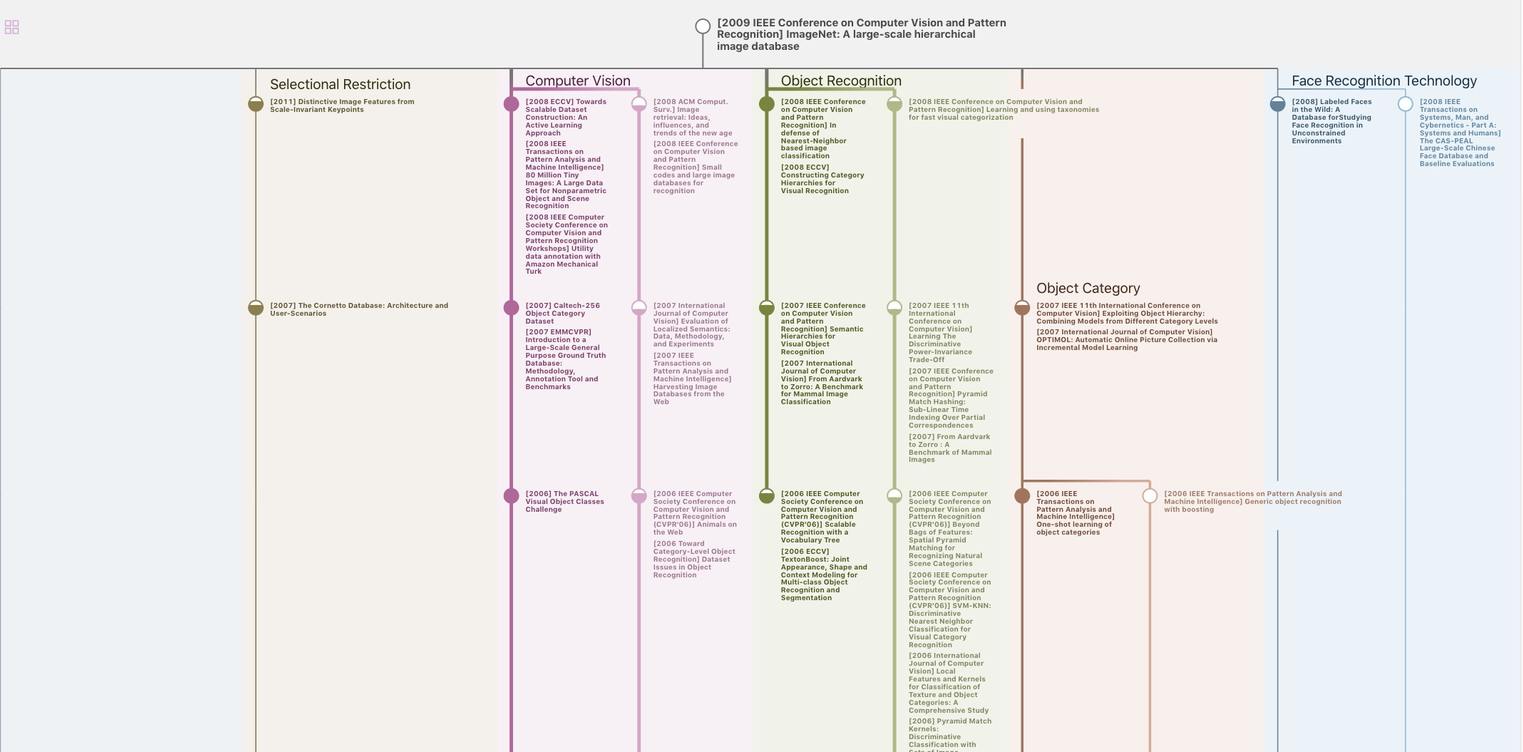
生成溯源树,研究论文发展脉络
Chat Paper
正在生成论文摘要