Phase Unwrapping of Color Doppler Echocardiography Using Deep Learning
IEEE transactions on ultrasonics, ferroelectrics, and frequency control(2023)
摘要
Color Doppler echocardiography is a widely used noninvasive imaging modality that provides real-time information about intracardiac blood flow. In an apical long-axis view of the left ventricle, color Doppler is subject to phase wrapping, or aliasing, especially during cardiac filling and ejection. When setting up quantitative methods based on color Doppler, it is necessary to correct this wrapping artifact. We developed an unfolded primal-dual network (PDNet) to unwrap (dealias) color Doppler echocardiographic images and compared its effectiveness against two state-of-the-art segmentation approaches based on nnU-Net and transformer models. We trained and evaluated the performance of each method on an in-house dataset and found that the nnU-Net-based method provided the best dealiased results, followed by the primal-dual approach and the transformer-based technique. Noteworthy, the PDNet, which had significantly fewer trainable parameters, performed competitively with respect to the other two methods, demonstrating the high potential of deep unfolding methods. Our results suggest that deep learning (DL)-based methods can effectively remove aliasing artifacts in color Doppler echocardiographic images, outperforming DeAN, a state-of-the-art semiautomatic technique. Overall, our results show that DL-based methods have the potential to effectively preprocess color Doppler images for downstream quantitative analysis.
更多查看译文
关键词
Color Doppler,dealiasing,deep learning (DL),deep unfolding,echocardiography,flow imaging,phase unwrapping,primal-dual,transformer,U-Net
AI 理解论文
溯源树
样例
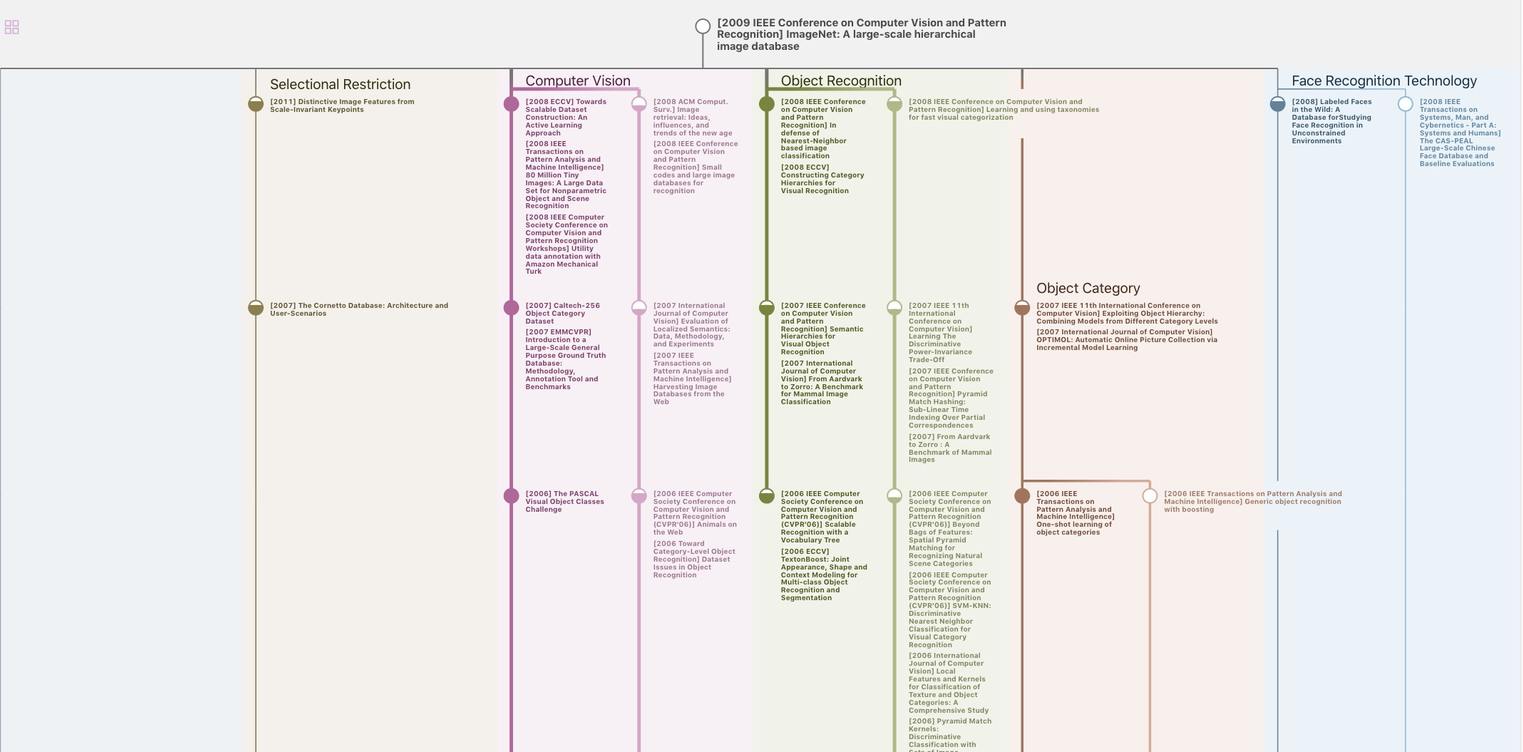
生成溯源树,研究论文发展脉络
Chat Paper
正在生成论文摘要