AugDMC: Data Augmentation Guided Deep Multiple Clustering
CoRR(2023)
摘要
Clustering aims to group similar objects together while separating dissimilar ones apart. Thereafter, structures hidden in data can be identified to help understand data in an unsupervised manner. Traditional clustering methods such as k-means provide only a single clustering for one data set. Deep clustering methods such as auto-encoder based clustering methods have shown a better performance, but still provide a single clustering. However, a given dataset might have multiple clustering structures and each represents a unique perspective of the data. Therefore, some multiple clustering methods have been developed to discover multiple independent structures hidden in data. Although deep multiple clustering methods provide better performance, how to efficiently capture the alternative perspectives in data is still a problem. In this paper, we propose AugDMC, a novel data Augmentation guided Deep Multiple Clustering method, to tackle the challenge. Specifically, AugDMC leverages data augmentations to automatically extract features related to a certain aspect of the data using a self-supervised prototype-based representation learning, where different aspects of the data can be preserved under different data augmentations. Moreover, a stable optimization strategy is proposed to alleviate the unstable problem from different augmentations. Thereafter, multiple clusterings based on different aspects of the data can be obtained. Experimental results on three real-world datasets compared with state-of-the-art methods validate the effectiveness of the proposed method.
更多查看译文
关键词
Multiple Clustering,Data Augmentation,Self-Supervised Representation Learning
AI 理解论文
溯源树
样例
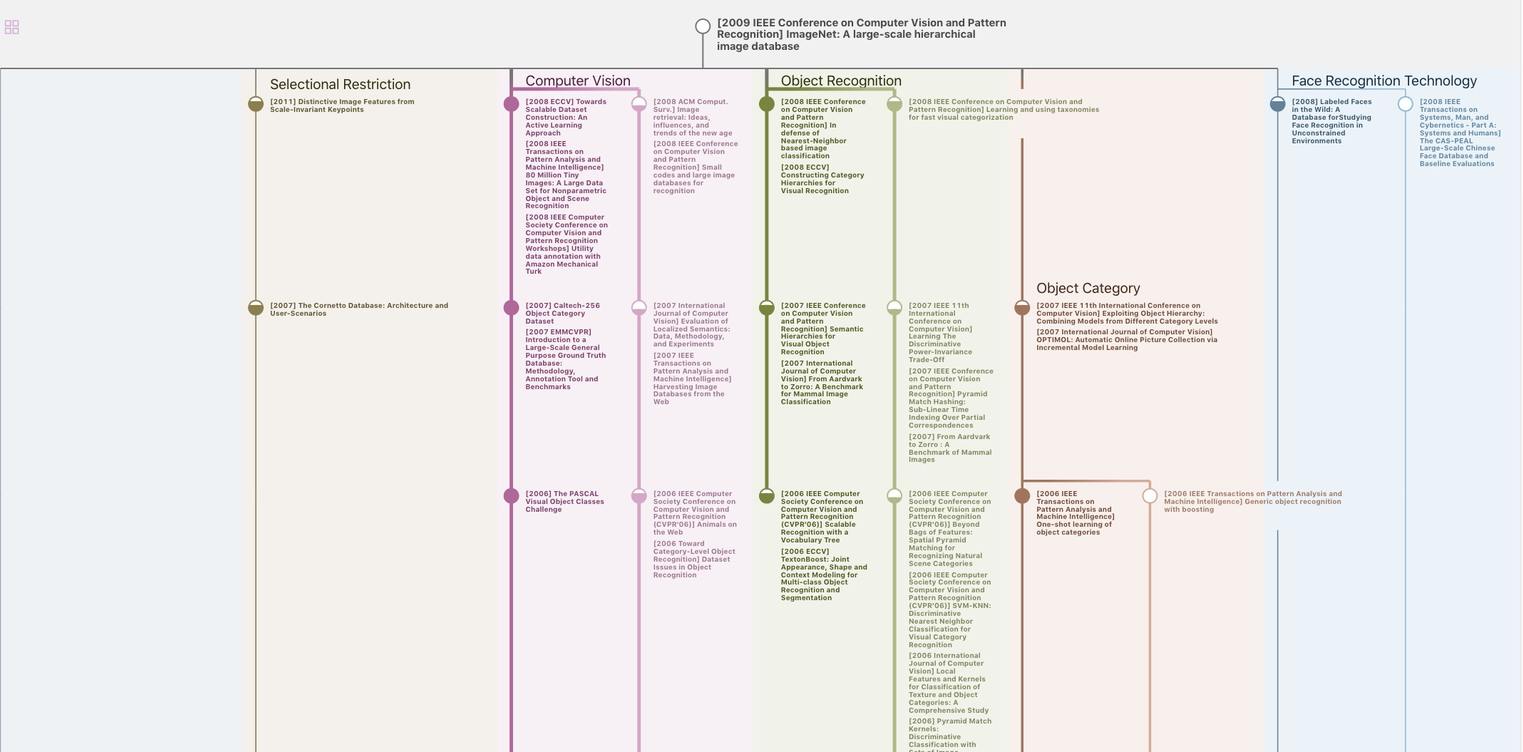
生成溯源树,研究论文发展脉络
Chat Paper
正在生成论文摘要