Verifying Global Neural Network Specifications using Hyperproperties
CoRR(2023)
摘要
Current approaches to neural network verification focus on specifications that target small regions around known input data points, such as local robustness. Thus, using these approaches, we can not obtain guarantees for inputs that are not close to known inputs. Yet, it is highly likely that a neural network will encounter such truly unseen inputs during its application. We study global specifications that - when satisfied - provide guarantees for all potential inputs. We introduce a hyperproperty formalism that allows for expressing global specifications such as monotonicity, Lipschitz continuity, global robustness, and dependency fairness. Our formalism enables verifying global specifications using existing neural network verification approaches by leveraging capabilities for verifying general computational graphs. Thereby, we extend the scope of guarantees that can be provided using existing methods. Recent success in verifying specific global specifications shows that attaining strong guarantees for all potential data points is feasible.
更多查看译文
关键词
global neural network specifications,hyperproperties,neural network
AI 理解论文
溯源树
样例
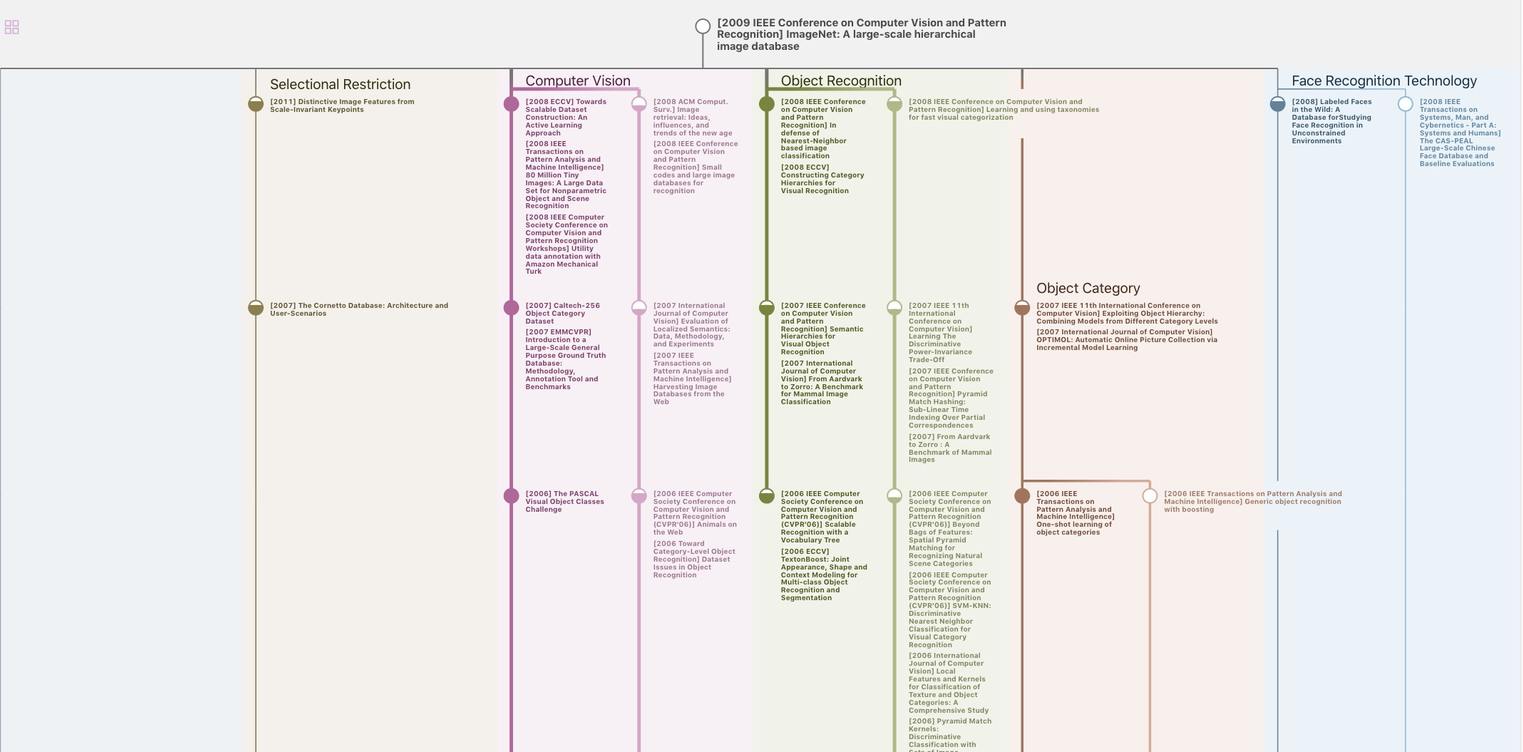
生成溯源树,研究论文发展脉络
Chat Paper
正在生成论文摘要