Disjunctive Threshold Networks for Tabular Data Classification
IEEE Open Journal of the Computer Society(2023)
摘要
While neural networks have been achieving increasingly significant excitement in solving classification tasks such as natural language processing, their lack of interpretability becomes a great challenge for neural networks to be deployed in certain high-stakes human-centered applications. To address this issue, we propose a new approach for generating interpretable predictions by inferring a simple three-layer neural network with threshold activations, so that it can benefit from effective neural network training algorithms and at the same time, produce human-understandable explanations for the results. In particular, the hidden layer neurons in the proposed model are trained with floating point weights and binary output activations. The output neuron is also trainable as a threshold logic function that implements a disjunctive operation, forming the logical-OR of the first-level threshold logic functions. This neural network can be trained using state-of-the-art training methods to achieve high prediction accuracy. An important feature of the proposed architecture is that only a simple greedy algorithm is required to provide an explanation with the prediction that is human-understandable. In comparison with other explainable decision models, our proposed approach achieves more accurate predictions on a broad set of tabular data classification datasets.
更多查看译文
关键词
Interpretable artificial intelligence,decision rule learning,tabular data classification,neural networks
AI 理解论文
溯源树
样例
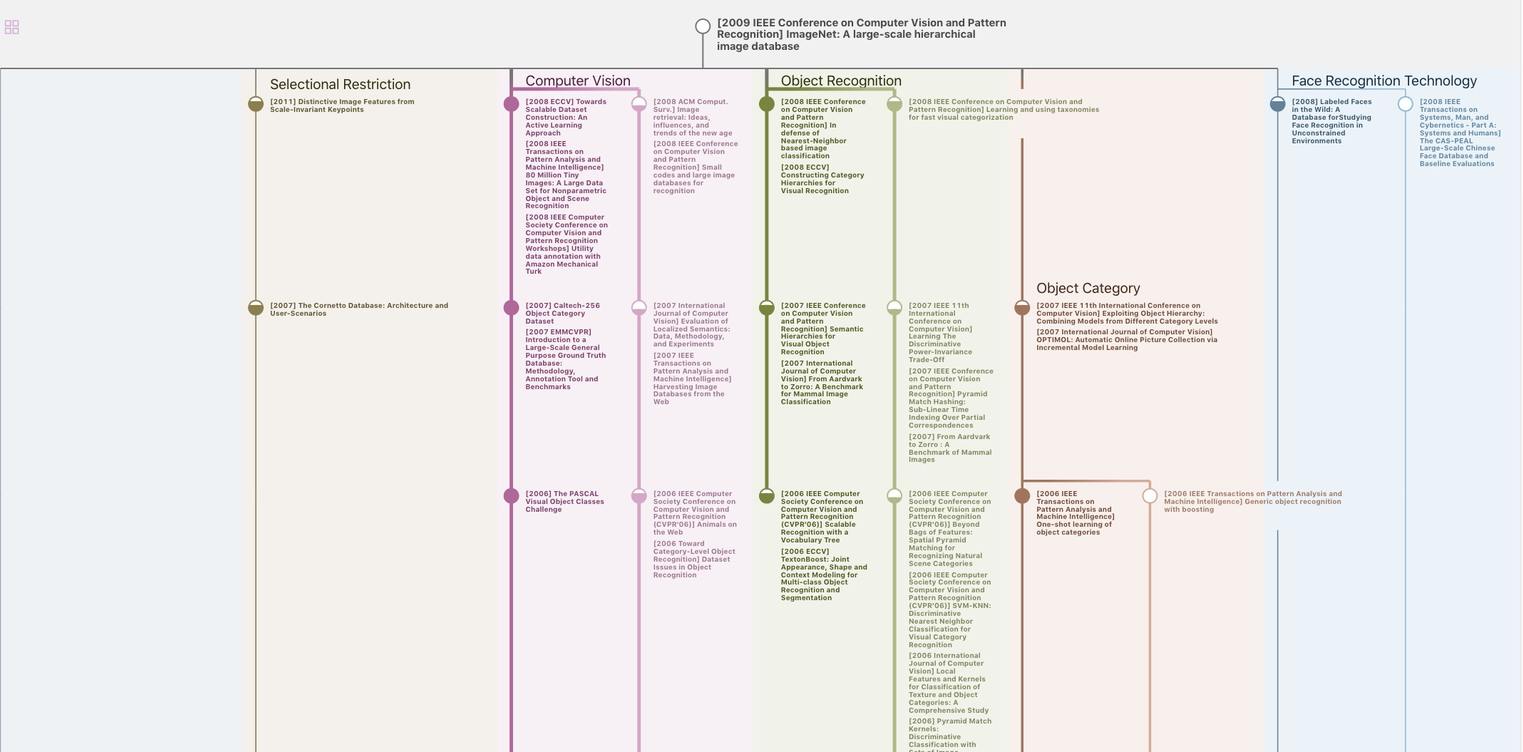
生成溯源树,研究论文发展脉络
Chat Paper
正在生成论文摘要