Improving Load Forecasting Performance via Sample Reweighting
IEEE Transactions on Smart Grid(2023)
摘要
Load forecasting techniques have been well developed and almost all typical models are trying to minimize the error over all equally weighted data samples. However, treating all data equally in the training process is unreasonable and even harmful to model generalization. This letter investigates the issue of data inequality which is crucial but is overlooked in the field of load forecasting. To this end, we propose a novel load forecasting method via sample reweighting to tackle the data inequality issue. The proposed method determines the weights of samples using their gradients and influence functions in the process of training. Case studies on a real-world load dataset using linear models, artificial neural networks, and long short term memory in both deterministic and probabilistic situations all reveal that assigning different weights to various samples can improve forecasting accuracy.
更多查看译文
关键词
load forecasting performance
AI 理解论文
溯源树
样例
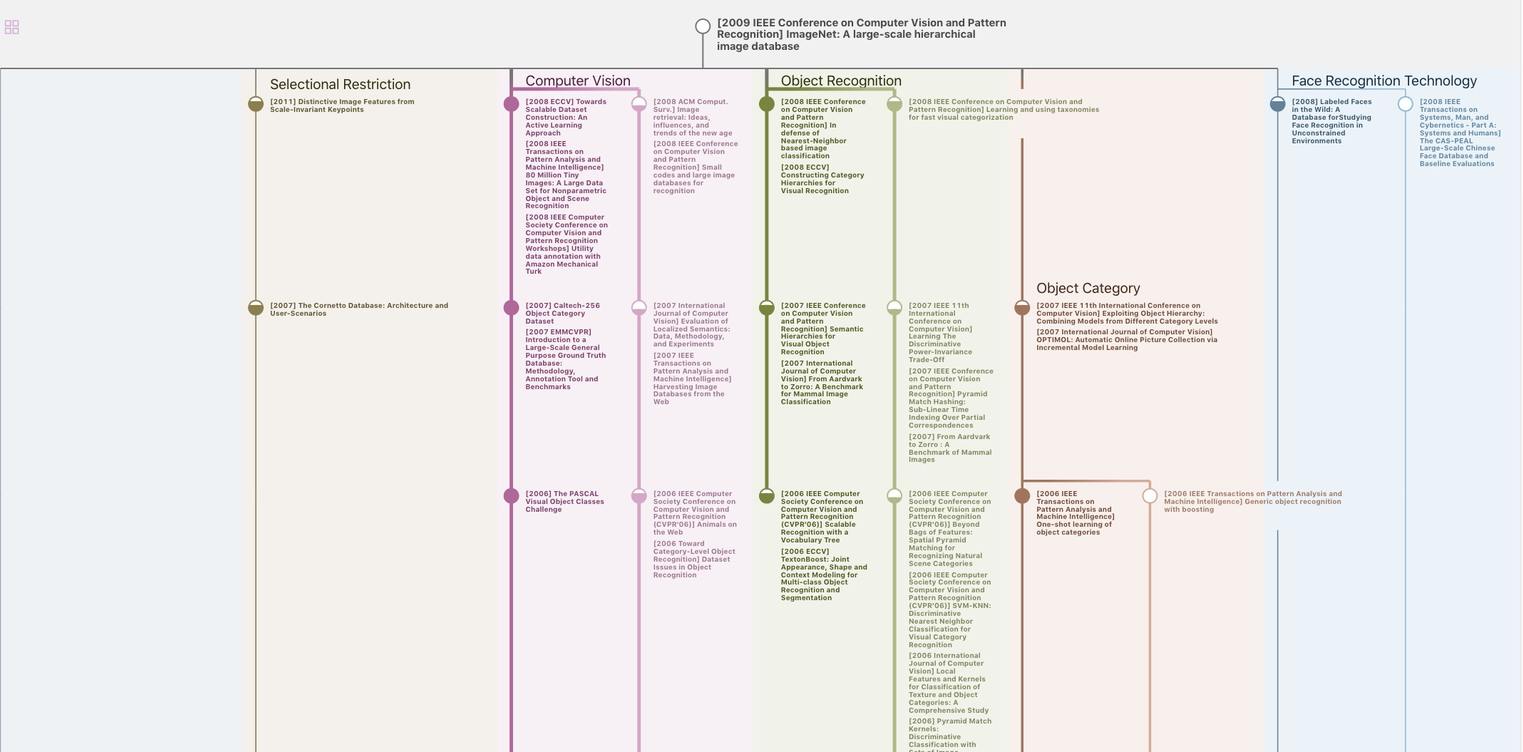
生成溯源树,研究论文发展脉络
Chat Paper
正在生成论文摘要