PrivSketch: A Private Sketch-based Frequency Estimation Protocol for Data Streams
CoRR(2023)
摘要
Local differential privacy (LDP) has recently become a popular privacy-preserving data collection technique protecting users' privacy. The main problem of data stream collection under LDP is the poor utility due to multi-item collection from a very large domain. This paper proposes PrivSketch, a high-utility frequency estimation protocol taking advantage of sketches, suitable for private data stream collection. Combining the proposed background information and a decode-first collection-side workflow, PrivSketch improves the utility by reducing the errors introduced by the sketching algorithm and the privacy budget utilization when collecting multiple items. We analytically prove the superior accuracy and privacy characteristics of PrivSketch, and also evaluate them experimentally. Our evaluation, with several diverse synthetic and real datasets, demonstrates that PrivSketch is 1-3 orders of magnitude better than the competitors in terms of utility in both frequency estimation and frequent item estimation, while being up to ~100x faster.
更多查看译文
关键词
frequency estimation protocol,privsketch,sketch-based
AI 理解论文
溯源树
样例
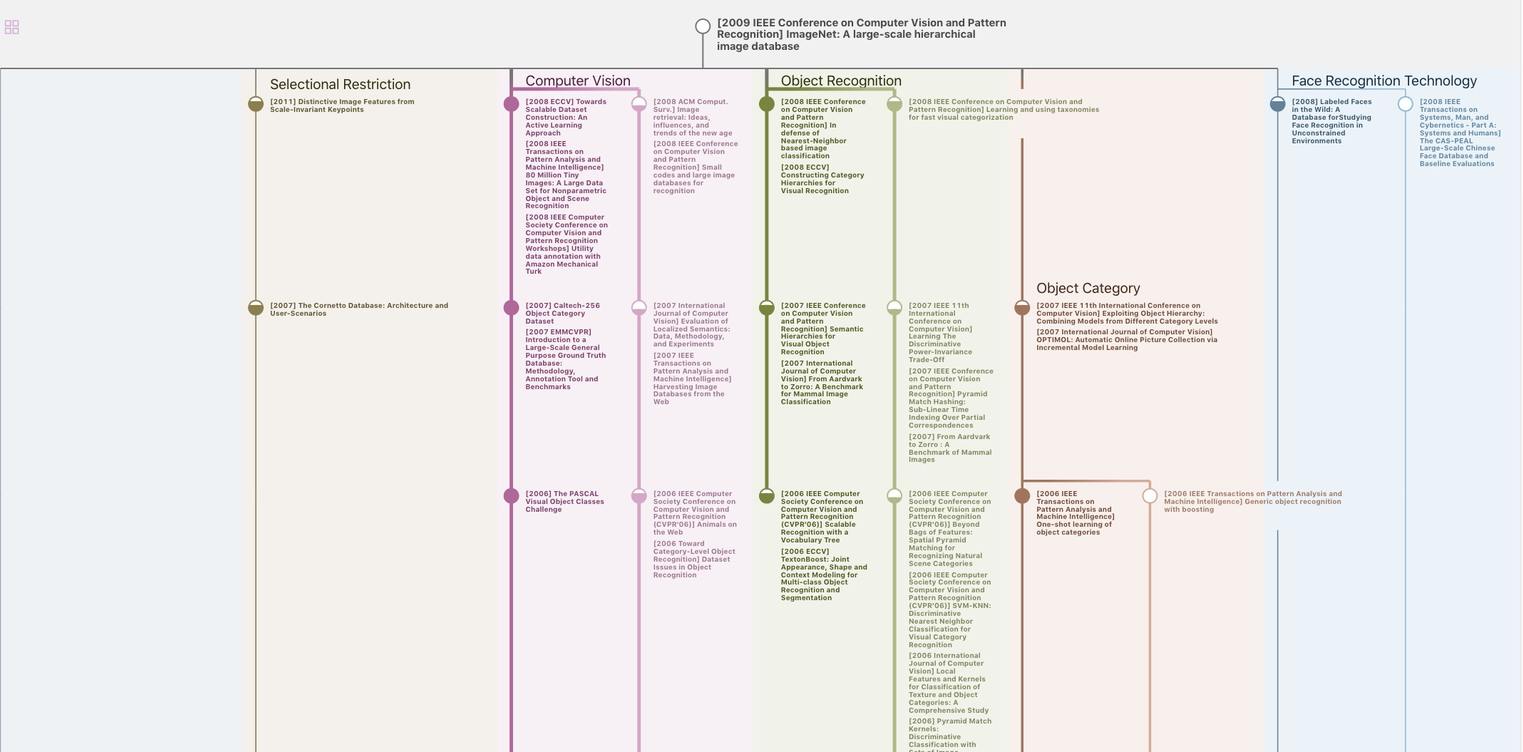
生成溯源树,研究论文发展脉络
Chat Paper
正在生成论文摘要