BMAD: Benchmarks for Medical Anomaly Detection
CoRR(2023)
Abstract
Anomaly detection (AD) is a fundamental research problem in machine learning and computer vision, with practical applications in industrial inspection, video surveillance, and medical diagnosis. In medical imaging, AD is especially vital for detecting and diagnosing anomalies that may indicate rare diseases or conditions. However, there is a lack of a universal and fair benchmark for evaluating AD methods on medical images, which hinders the development of more generalized and robust AD methods in this specific domain. To bridge this gap, we introduce a comprehensive evaluation benchmark for assessing anomaly detection methods on medical images. This benchmark encompasses six reorganized datasets from five medical domains (i.e. brain MRI, liver CT, retinal OCT, chest X-ray, and digital histopathology) and three key evaluation metrics, and includes a total of fourteen state-of-the-art AD algorithms. This standardized and well-curated medical benchmark with the well-structured codebase enables comprehensive comparisons among recently proposed anomaly detection methods. It will facilitate the community to conduct a fair comparison and advance the field of AD on medical imaging. More information on BMAD is available in our GitHub repository: https://github.com/DorisBao/BMAD
MoreTranslated text
Key words
medical anomaly detection,anomaly detection,benchmarks
AI Read Science
Must-Reading Tree
Example
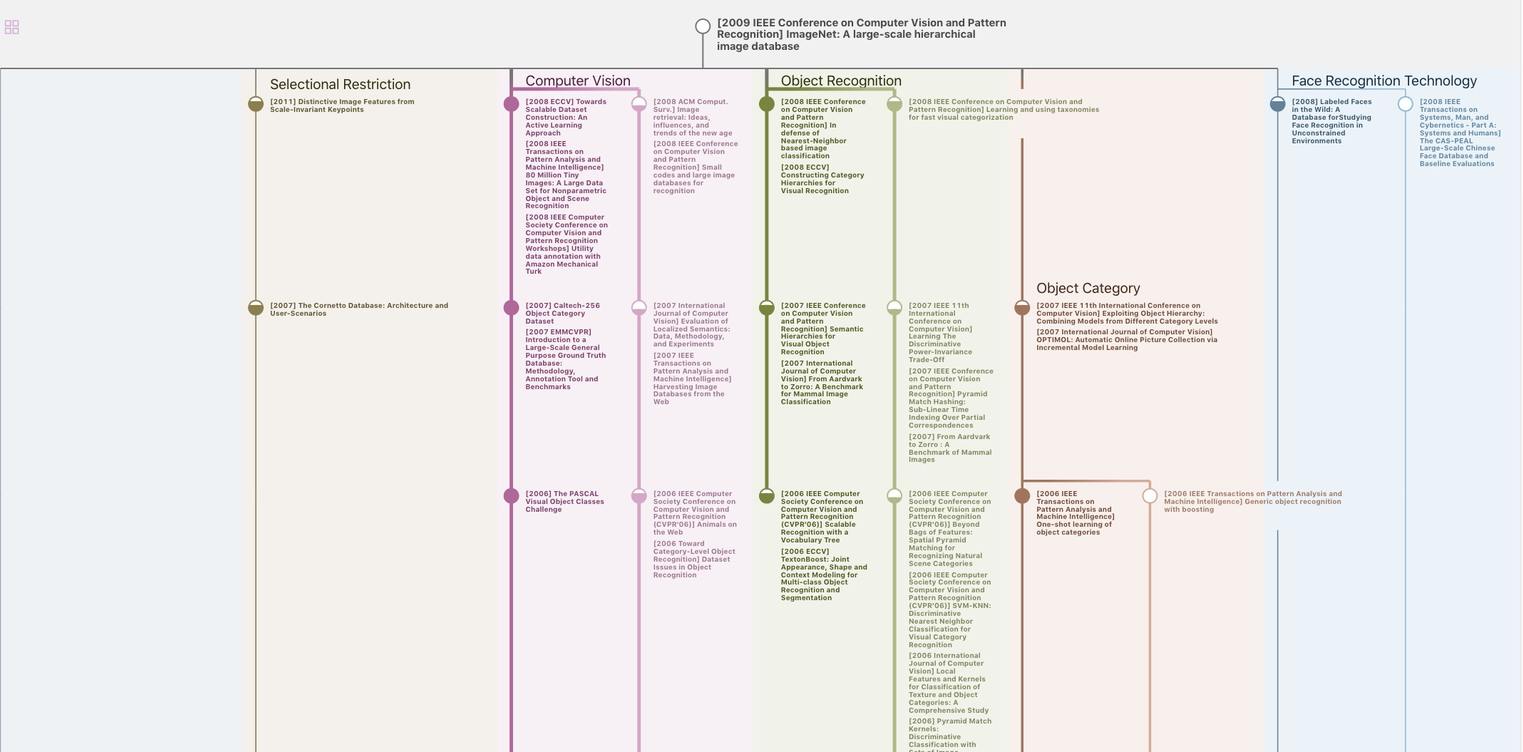
Generate MRT to find the research sequence of this paper
Chat Paper
Summary is being generated by the instructions you defined