On Frequency-Wise Normalizations for Better Recording Device Generalization in Audio Spectrogram Transformers.
CoRR(2023)
摘要
Varying conditions between the data seen at training and at application time remain a major challenge for machine learning. We study this problem in the context of Acoustic Scene Classification (ASC) with mismatching recording devices. Previous works successfully employed frequency-wise normalization of inputs and hidden layer activations in convolutional neural networks to reduce the recording device discrepancy. The main objective of this work was to adopt frequency-wise normalization for Audio Spectrogram Transformers (ASTs), which have recently become the dominant model architecture in ASC. To this end, we first investigate how recording device characteristics are encoded in the hidden layer activations of ASTs. We find that recording device information is initially encoded in the frequency dimension; however, after the first self-attention block, it is largely transformed into the token dimension. Based on this observation, we conjecture that suppressing recording device characteristics in the input spectrogram is the most effective. We propose a frequency-centering operation for spectrograms that improves the ASC performance on unseen recording devices on average by up to 18.2 percentage points.
更多查看译文
关键词
better recording device
AI 理解论文
溯源树
样例
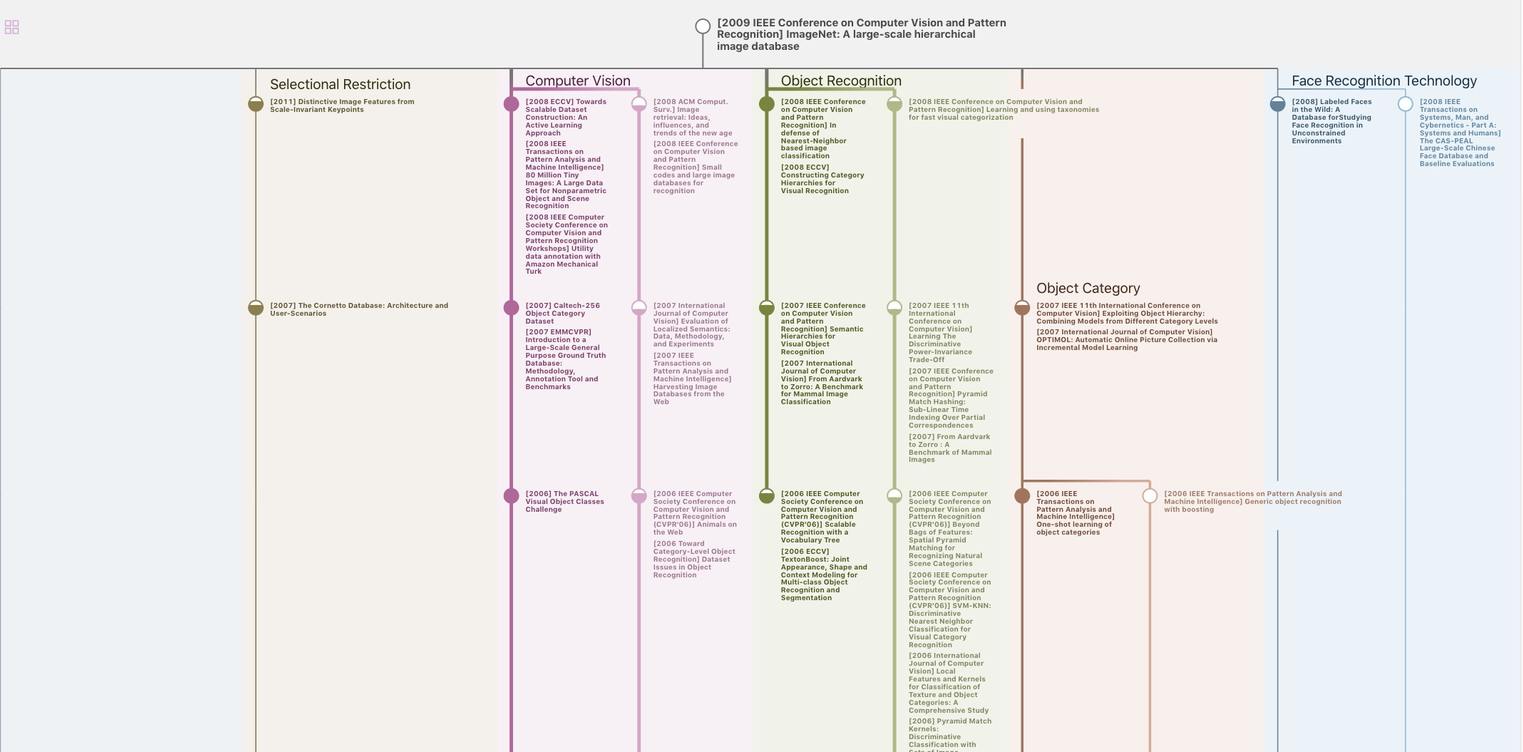
生成溯源树,研究论文发展脉络
Chat Paper
正在生成论文摘要