A Computer Vision-based Pothole and Road Distress Detection System for Extreme Weather and Natural Disaster-Prone Fiji
2023 IEEE International Conference on Advanced Systems and Emergent Technologies (IC_ASET)(2023)
摘要
Fiji's location makes it vulnerable to extreme weather and natural disasters, resulting in heavy rainfall, poor drainage, and deteriorating road structures. Consequently, potholes have become more common, leading to increased vehicle part replacements. The authorities require assistance in locating and quantifying potholes, as monitoring road cracks can help assess the severity of road deterioration, which is the preliminary stage of road degradation. This paper presents a pothole and road distress detection system that employs computer vision techniques based on the TensorFlow Lite mobile library. The models were trained based on the EfficientDet-Lite family architecture using a custom dataset created from photographs of potholes and road cracks in the Suva area. Notably, the model was trained with fewer images than typically required and deployed onto a Raspberry Pi 4 - based handheld prototype. The EfficientDet-Lite0 model provided the fastest detection rate of 6.7 frames per second, making it suitable for detecting both potholes and road cracks at an average walking speed, with an average precision of 17%
更多查看译文
关键词
Pothole detection,TensorFlow Lite,Raspberry Pi,Real-time object detection
AI 理解论文
溯源树
样例
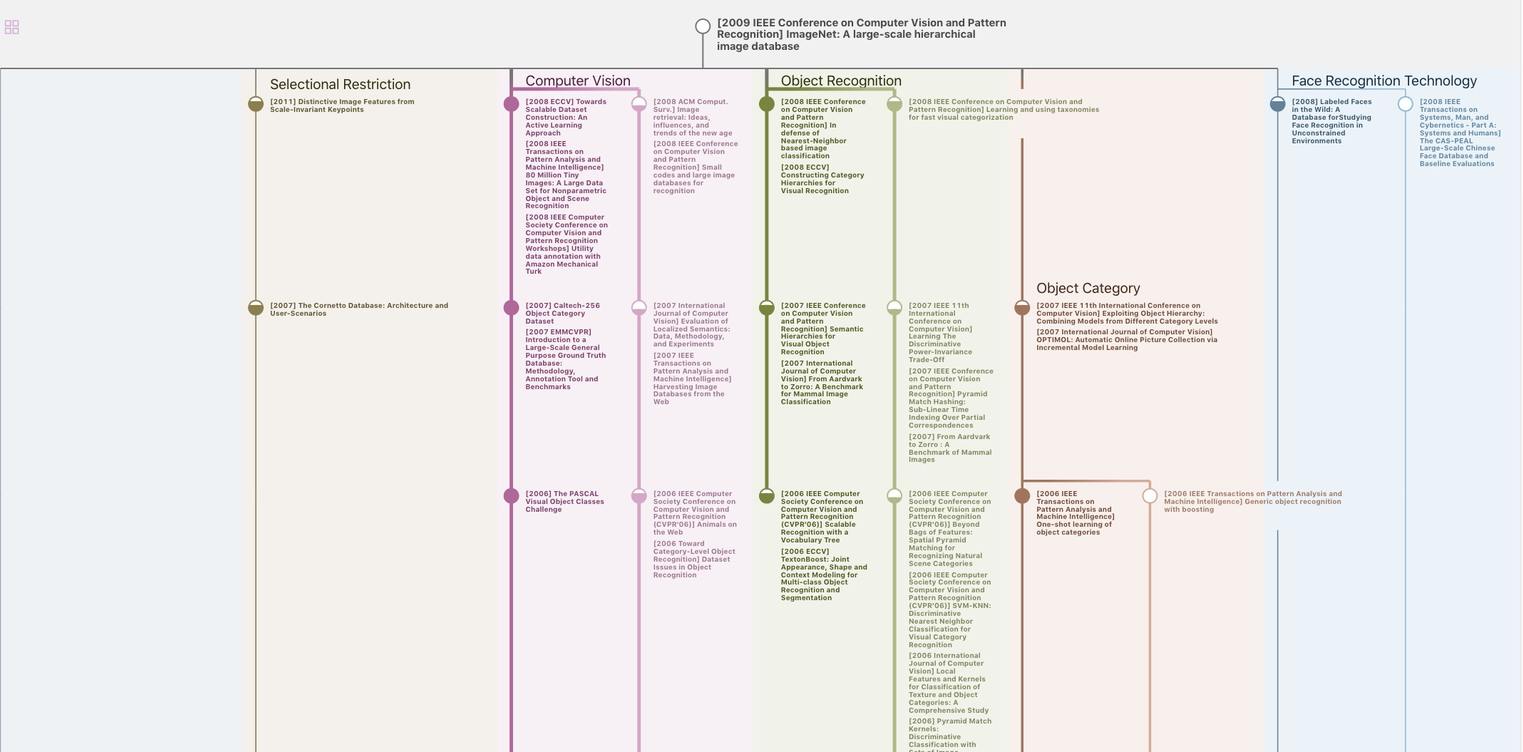
生成溯源树,研究论文发展脉络
Chat Paper
正在生成论文摘要