Object Recognition Using Mechanical Impact, Viscoelasticity, and Surface Friction During Interaction
IEEE Transactions on Haptics(2023)
摘要
Current robotic haptic object recognition relies on statistical measures derived from movement dependent interaction signals such as force, vibration or position. Mechanical properties, which can be estimated from these signals, are intrinsic object properties that may yield a more robust object representation. Therefore, this paper proposes an object recognition framework using multiple representative mechanical properties: stiffness, viscosity and friction coefficient as well as the coefficient of restitution, which has been rarely used to recognise objects. These properties are estimated in real-time using a dual Kalman filter (without tangential force measurements) and then are used for object classification and clustering. The proposed framework was tested on a robot identifying 20 objects through haptic exploration. The results demonstrate the technique's effectiveness and efficiency, and that all four mechanical properties are required for the best recognition rate of 98.18
$\pm$
0.424%. For object clustering, the use of these mechanical properties also results in superior performance when compared to methods based on statistical parameters.
更多查看译文
关键词
Haptic exploration, interaction mechanics, feature extraction, supervised learning for classification, clustering
AI 理解论文
溯源树
样例
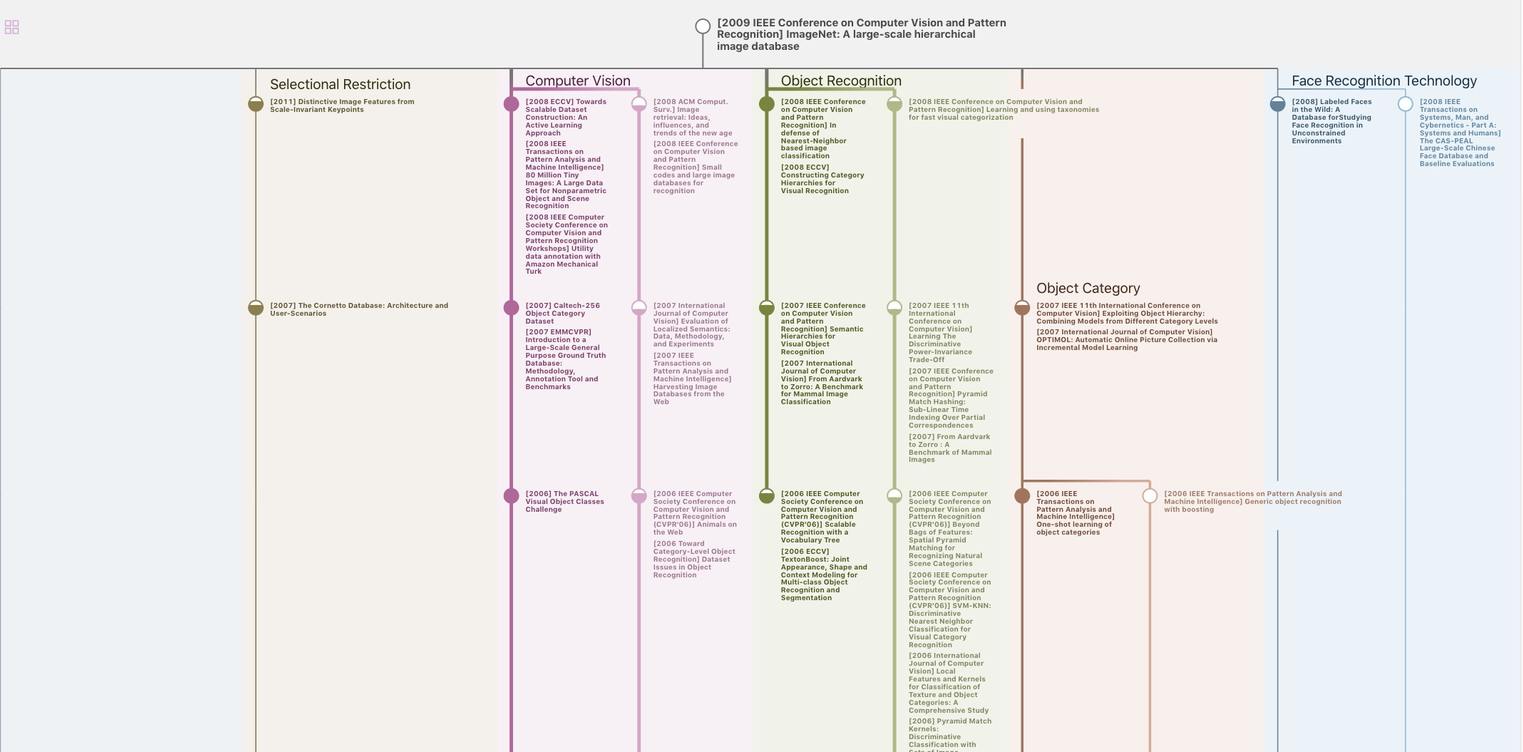
生成溯源树,研究论文发展脉络
Chat Paper
正在生成论文摘要