Discrepancies and the Error Evaluation Metrics for Machine Learning Interatomic Potentials
NPJ COMPUTATIONAL MATERIALS(2023)
摘要
Machine learning interatomic potentials (MLPs) are a promising technique for atomic modeling. While high accuracy and small errors are widely reported for MLPs, an open concern is whether MLPs can accurately reproduce atomistic dynamics and related physical properties in their applications in molecular dynamics (MD) simulations. In this study, we examine the current state-of-the-art MLPs and uncover a number of discrepancies related to atom dynamics, defects, and rare events (REs), in their MD simulations compared to ab initio methods. Our findings reveal that low averaged errors by current MLP testing are insufficient, leading us to develop novel quantitative metrics that better indicate the accurate prediction of related properties by MLPs in MD simulations. The MLPs optimized by the RE-based evaluation metrics are demonstrated to have improved prediction in multiple properties. The identified errors, the developed evaluation metrics, and the proposed process of developing such metrics are general to MLPs, thus providing valuable guidance for future testing, development, and improvements of accurate, robust, and reliable MLPs for atomistic modeling.
更多查看译文
AI 理解论文
溯源树
样例
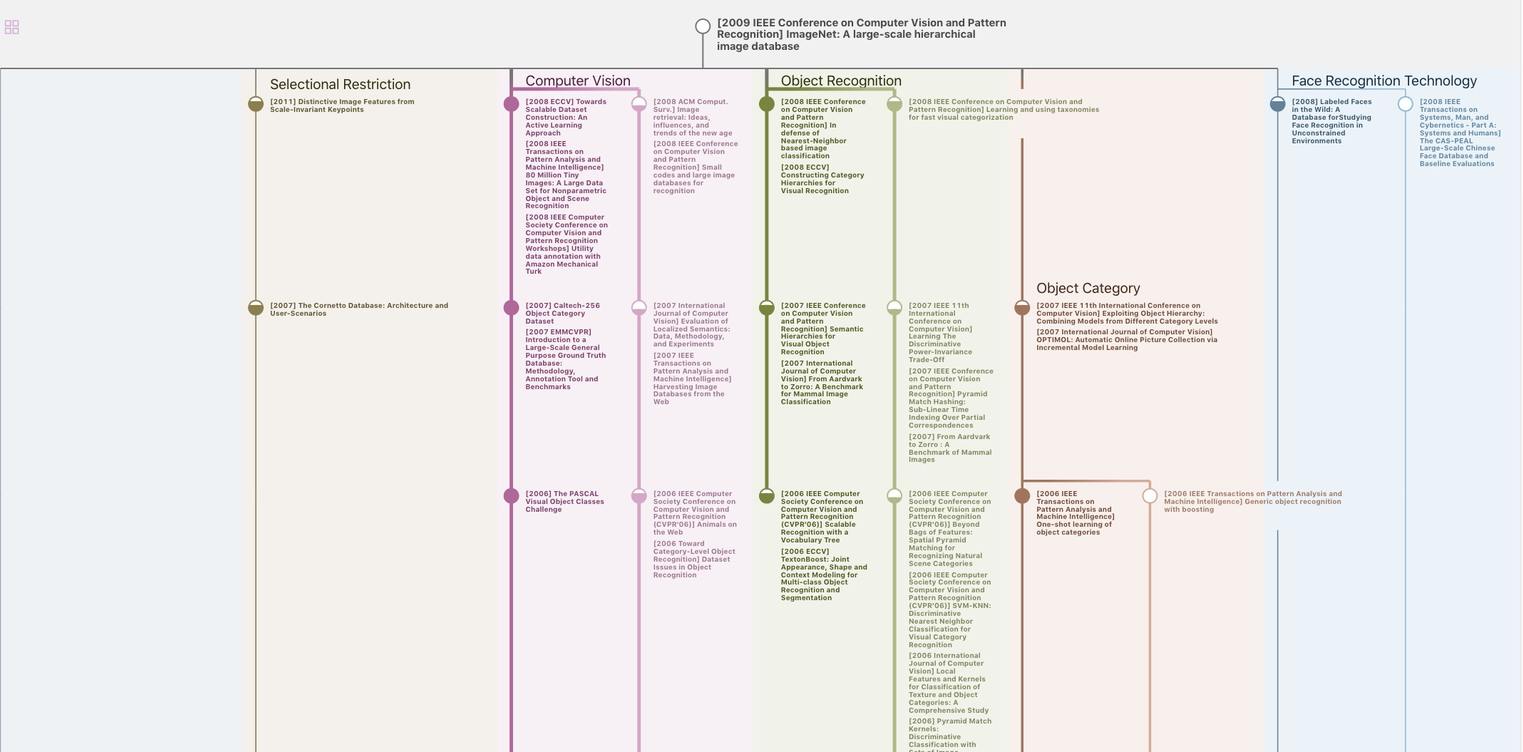
生成溯源树,研究论文发展脉络
Chat Paper
正在生成论文摘要