Informed POMDP: Leveraging Additional Information in Model-Based RL
CoRR(2023)
摘要
In this work, we generalize the problem of learning through interaction in a POMDP by accounting for eventual additional information available at training time. First, we introduce the informed POMDP, a new learning paradigm offering a clear distinction between the training information and the execution observation. Next, we propose an objective for learning a sufficient statistic from the history for the optimal control that leverages this information. We then show that this informed objective consists of learning an environment model from which we can sample latent trajectories. Finally, we show for the Dreamer algorithm that the convergence speed of the policies is sometimes greatly improved on several environments by using this informed environment model. Those results and the simplicity of the proposed adaptation advocate for a systematic consideration of eventual additional information when learning in a POMDP using model-based RL.
更多查看译文
关键词
informed pomdp,information,model-based
AI 理解论文
溯源树
样例
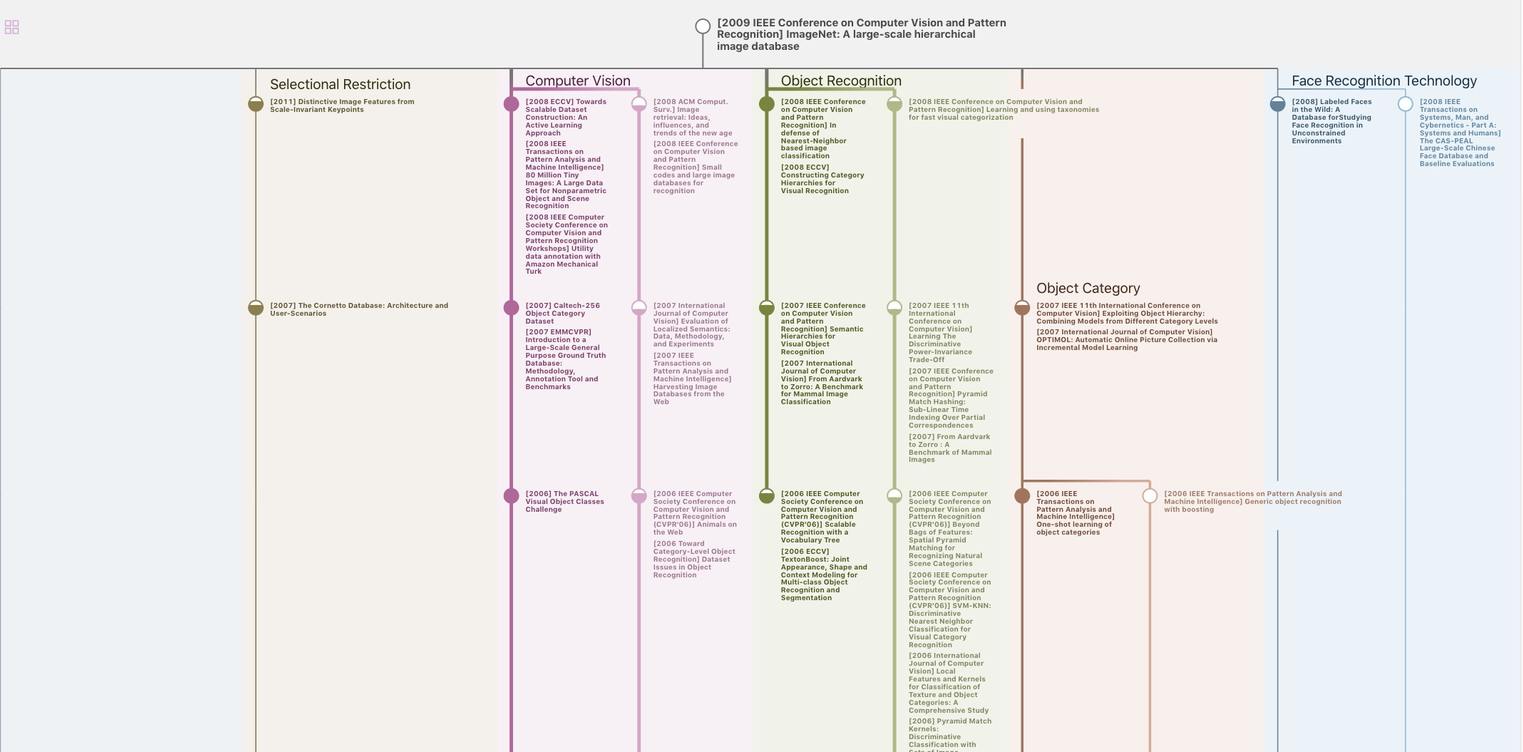
生成溯源树,研究论文发展脉络
Chat Paper
正在生成论文摘要