Exploring the Performance and Efficiency of Transformer Models for NLP on Mobile Devices
CoRR(2023)
摘要
Deep learning (DL) is characterised by its dynamic nature, with new deep neural network (DNN) architectures and approaches emerging every few years, driving the field's advancement. At the same time, the ever-increasing use of mobile devices (MDs) has resulted in a surge of DNN-based mobile applications. Although traditional architectures, like CNNs and RNNs, have been successfully integrated into MDs, this is not the case for Transformers, a relatively new model family that has achieved new levels of accuracy across AI tasks, but poses significant computational challenges. In this work, we aim to make steps towards bridging this gap by examining the current state of Transformers' on-device execution. To this end, we construct a benchmark of representative models and thoroughly evaluate their performance across MDs with different computational capabilities. Our experimental results show that Transformers are not accelerator-friendly and indicate the need for software and hardware optimisations to achieve efficient deployment.
更多查看译文
关键词
transformer models,nlp
AI 理解论文
溯源树
样例
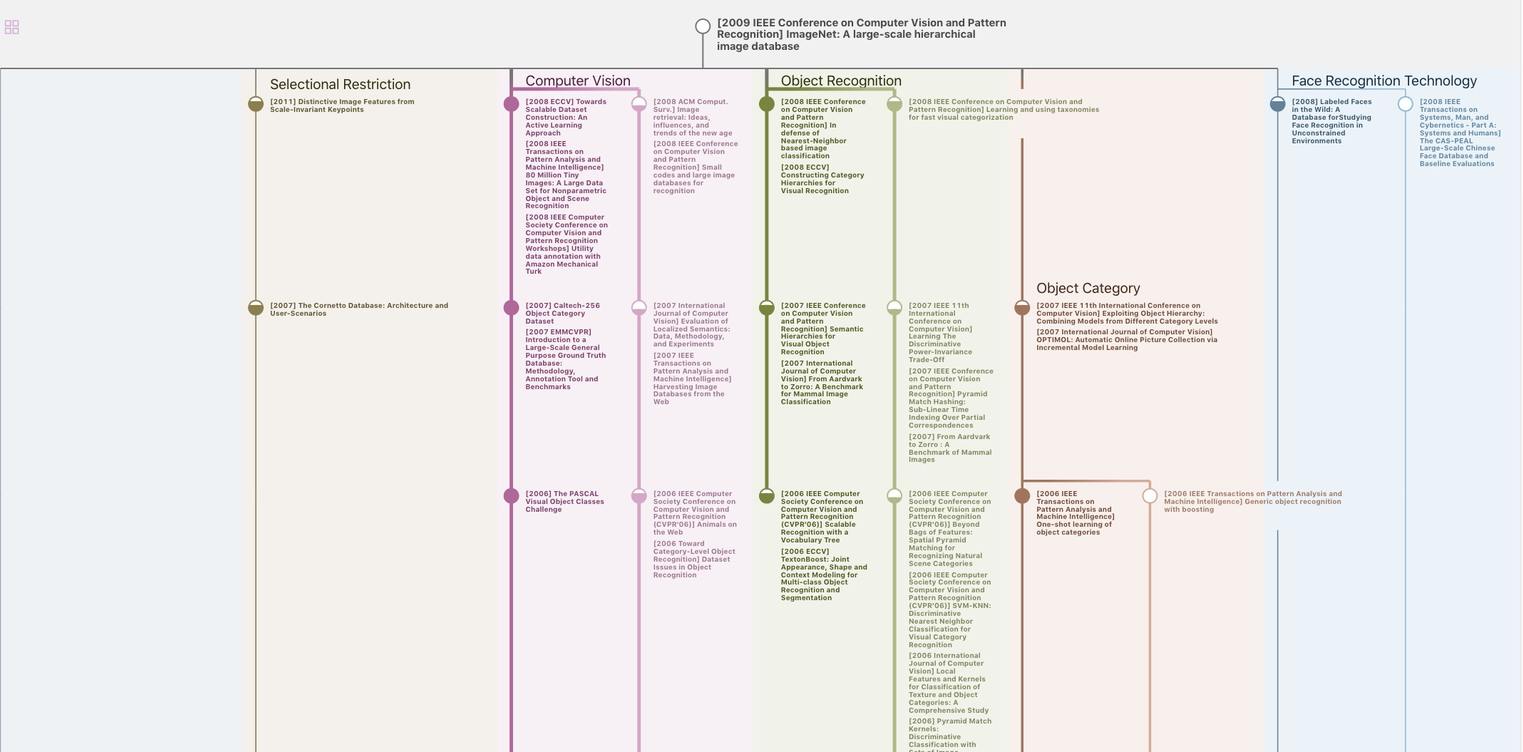
生成溯源树,研究论文发展脉络
Chat Paper
正在生成论文摘要