Low Latency Edge Classification GNN for Particle Trajectory Tracking on FPGAs
CoRR(2023)
摘要
In-time particle trajectory reconstruction in the Large Hadron Collider is challenging due to the high collision rate and numerous particle hits. Using GNN (Graph Neural Network) on FPGA has enabled superior accuracy with flexible trajectory classification. However, existing GNN architectures have inefficient resource usage and insufficient parallelism for edge classification. This paper introduces a resource-efficient GNN architecture on FPGAs for low latency particle tracking. The modular architecture facilitates design scalability to support large graphs. Leveraging the geometric properties of hit detectors further reduces graph complexity and resource usage. Our results on Xilinx UltraScale+ VU9P demonstrate 1625x and 1574x performance improvement over CPU and GPU respectively.
更多查看译文
关键词
Graph Neural Network,Particle tracking,FPGA
AI 理解论文
溯源树
样例
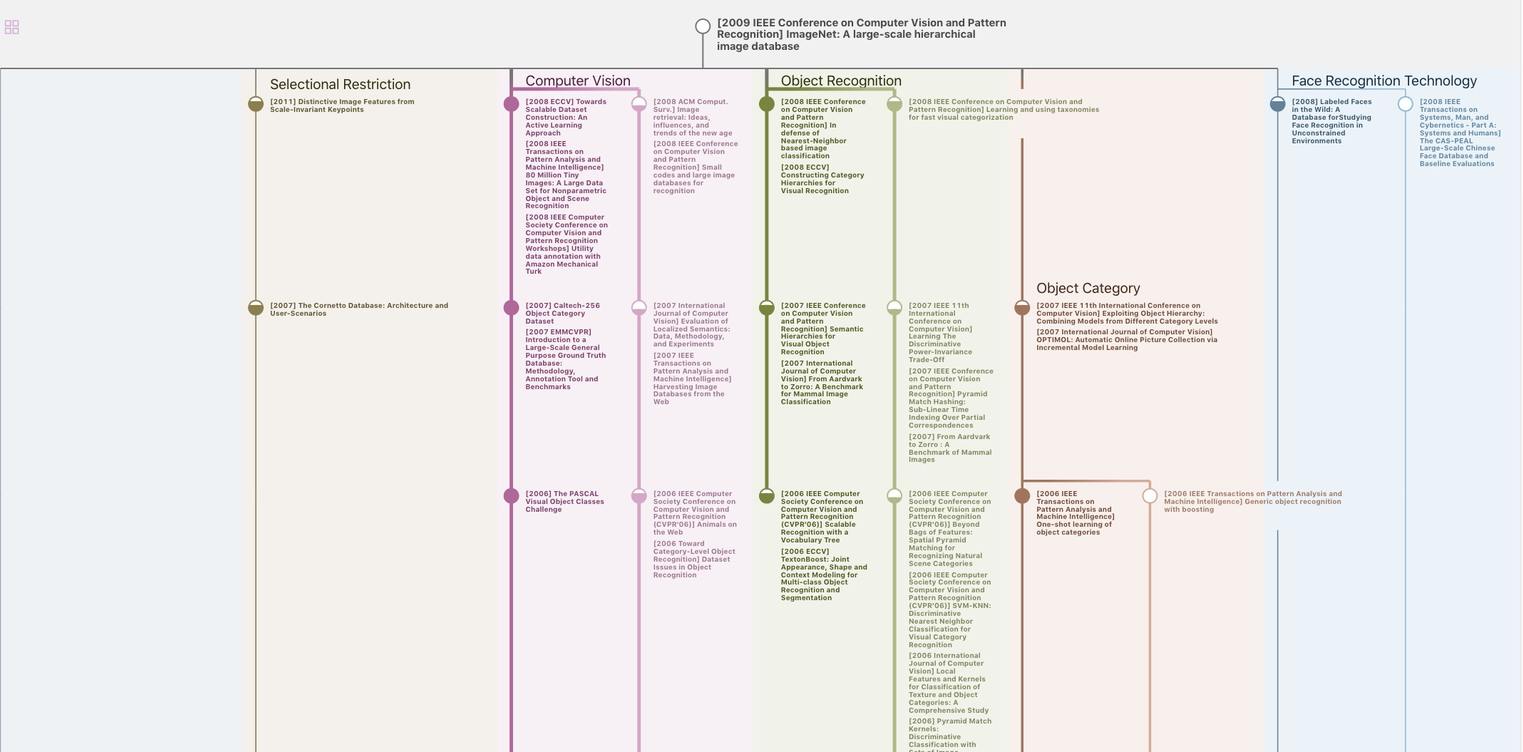
生成溯源树,研究论文发展脉络
Chat Paper
正在生成论文摘要