A Lightweight Causal Model for Interpretable Subject-level Prediction
CoRR(2023)
摘要
Recent years have seen a growing interest in methods for predicting a variable of interest, such as a subject's diagnosis, from medical images. Methods based on discriminative modeling excel at making accurate predictions, but are challenged in their ability to explain their decisions in anatomically meaningful terms. In this paper, we propose a simple technique for single-subject prediction that is inherently interpretable. It augments the generative models used in classical human brain mapping techniques, in which cause-effect relations can be encoded, with a multivariate noise model that captures dominant spatial correlations. Experiments demonstrate that the resulting model can be efficiently inverted to make accurate subject-level predictions, while at the same time offering intuitive causal explanations of its inner workings. The method is easy to use: training is fast for typical training set sizes, and only a single hyperparameter needs to be set by the user. Our code is available at https://github.com/chiara-mauri/Interpretable-subject-level-prediction.
更多查看译文
关键词
lightweight causal model,prediction,subject-level
AI 理解论文
溯源树
样例
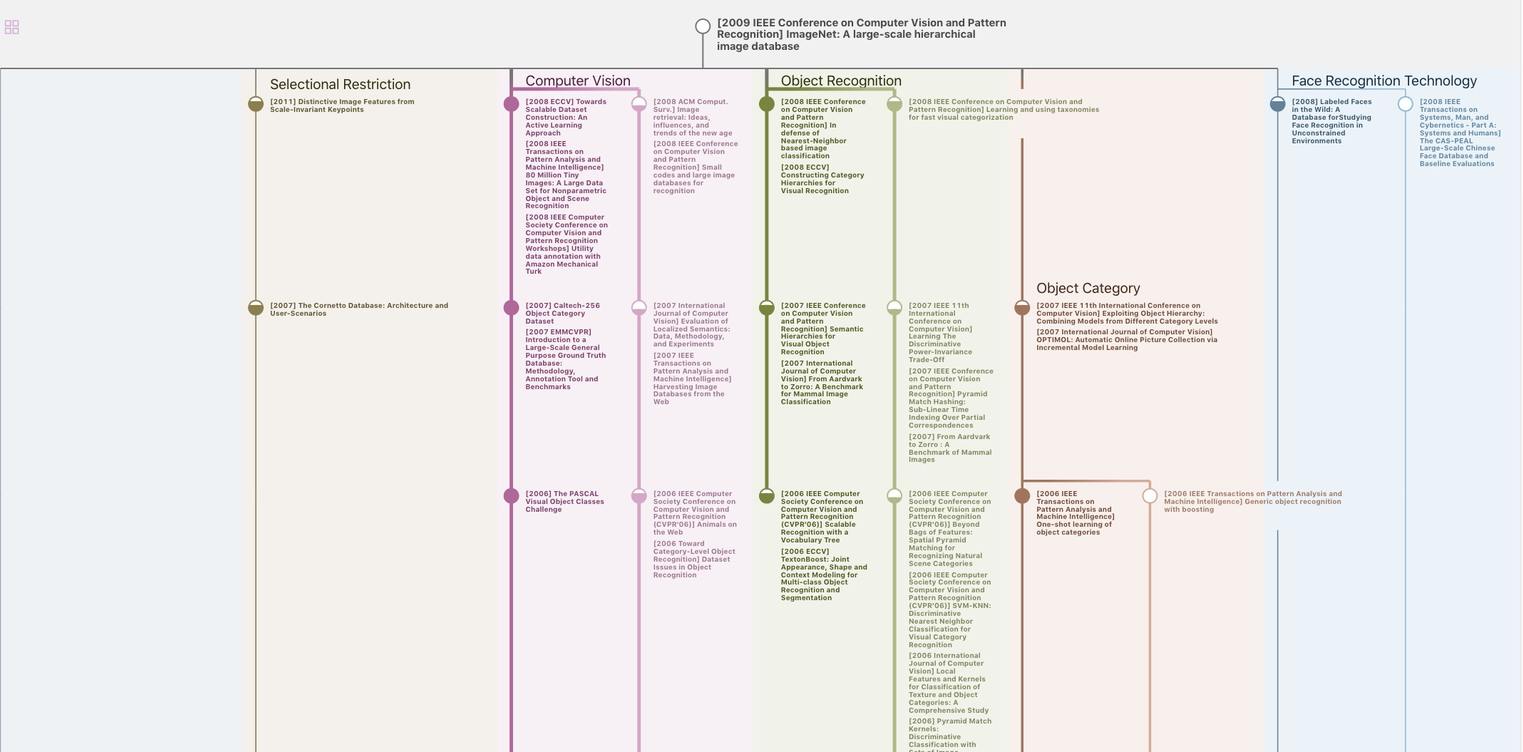
生成溯源树,研究论文发展脉络
Chat Paper
正在生成论文摘要